An Efficient Classification of Focal and Non-Focal EEG Signals Using Adaptive DCT Filter Bank
CIRCUITS SYSTEMS AND SIGNAL PROCESSING(2023)
摘要
A precise identification of the epileptogenic focus in the brain plays a significant role in treating patients suffering from pharmacoresistant focal epilepsy. Various machine learning techniques have been developed recently to aid neurologists in accurate diagnosis for epileptic patients. In this work, the epileptogenic region is identified by detecting focal (F) EEG signals using Fourier-based signal decomposition scheme. The F and non-focal (NF) EEG signals are segregated into various Fourier intrinsic band functions (FIBFs), obtained by dividing the entire bandwidth of the signal into uniform frequency bands, using discrete cosine transform (DCT)-based filter bank. Six features, namely variance, interquartile range, complexity, kurtosis, range, and mean frequency, are calculated from FIBFs of the original EEG signals. In addition to this, complexity, mean frequency, and interquartile range are also extracted from the first derivative of the EEG signals. To reduce computational complexity, the Wilcoxon rank-sum statistical test is employed to check the discriminating ability of the obtained features. Applying a support vector machine (SVM) classifier with tenfold cross-validation scheme on publicly available Bern-Barcelona dataset, the proposed method achieved an average classification accuracy of 99.44% considering 50 signals each from both the categories. The proposed model is also validated on Bonn EEG dataset, and an average accuracy of 99.64% is obtained while discriminating between seizure and non-seizure EEG signals. This method produces better classification results than the existing state-of-the-art algorithms and can be easily implemented in real-time systems.
更多查看译文
关键词
Discrete cosine transform,Epileptogenic zone,Focal EEG,Fourier intrinsic band functions,Support vector machine
AI 理解论文
溯源树
样例
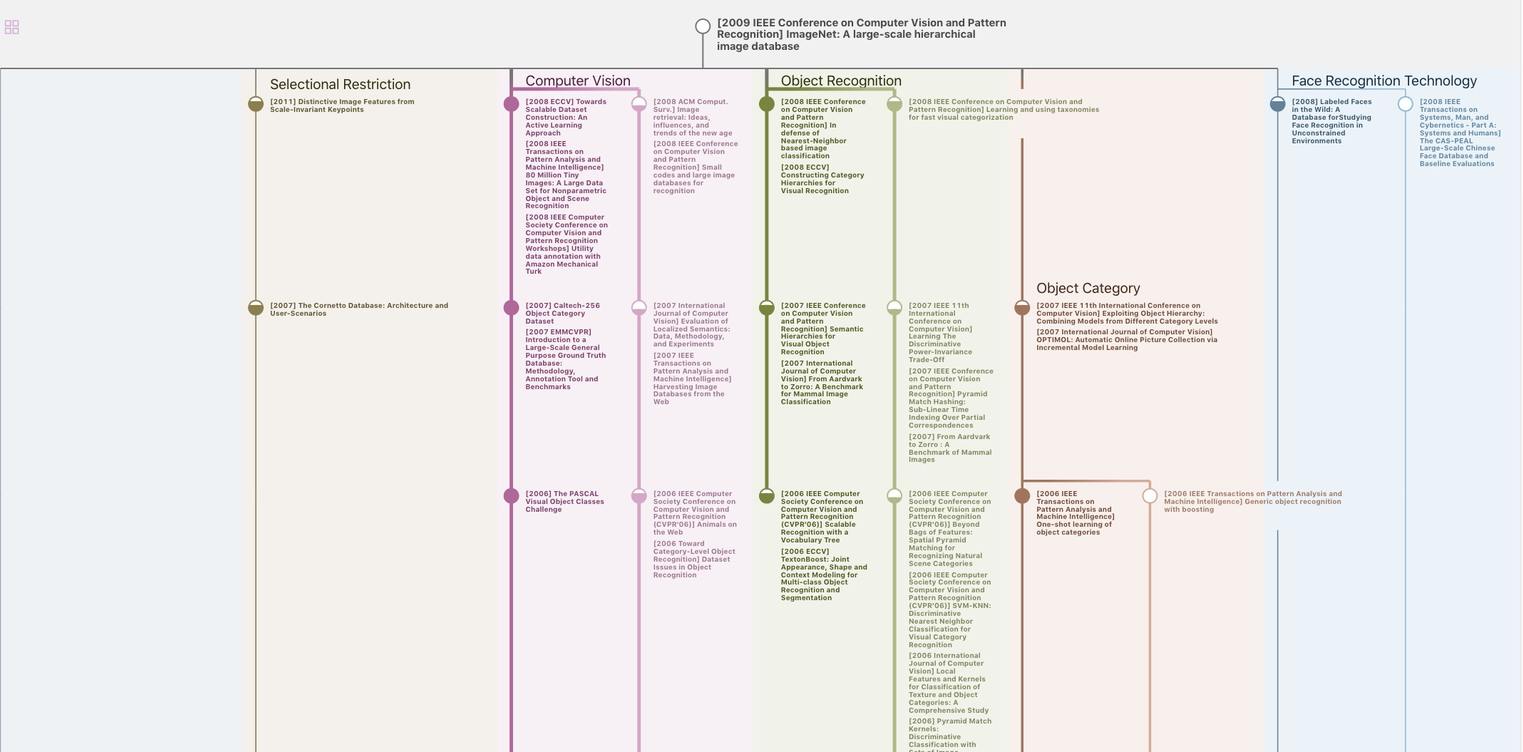
生成溯源树,研究论文发展脉络
Chat Paper
正在生成论文摘要