Random forest classifier and neural network for fraction identification of refuse-derived fuel images
FUEL(2023)
摘要
The current paper aims at the evaluation of computer vision methods to identify RDF (Refuse-derived fuels) fractions based on images of the RDF particles. For this purpose, images of 1345 single RDF particles, with a typical size in the cm-range, were taken in bird's eye view and assigned manually to one of six predefined material fractions.Two possible methods were tested for fraction identification: First, a classical machine learning approach consisting of feature extraction of color histograms and Haralick-textures as input for a random forest classifier and, second, a neural network approach with transfer learning.In the machine learning approach, the random forest classifier with feature extraction based on image color distribution (histograms of colors in images) and Haralick-textures (matrix of gray-value co-occurrences) ach-ieved an accuracy of 49 %. The neural network approach is based on the Xception network, a state-of-the-art convolutional neural network with depthwise separable convolutions. The accuracy of the Xception network for recognizing RDF fractions with transfer learning is 69%, thus considerably better than with the machine learning approach and a good starting point for identifying the very inhomogeneous appearance of RDF fractions. The Xception approach was then used in a more realistic setup where the RDF particles are transported by a conveyor belt which allowed for simplified image acquisition. Here, some additional measures are needed for image recognition like edge detection performed by the Canny algorithm. The accuracy for a test data set could be increased to 71 % after using images from this setup to improve the neural network.
更多查看译文
关键词
Refuse-derived fuel,Computer vision,Neural network,Xception,Conveyor belt
AI 理解论文
溯源树
样例
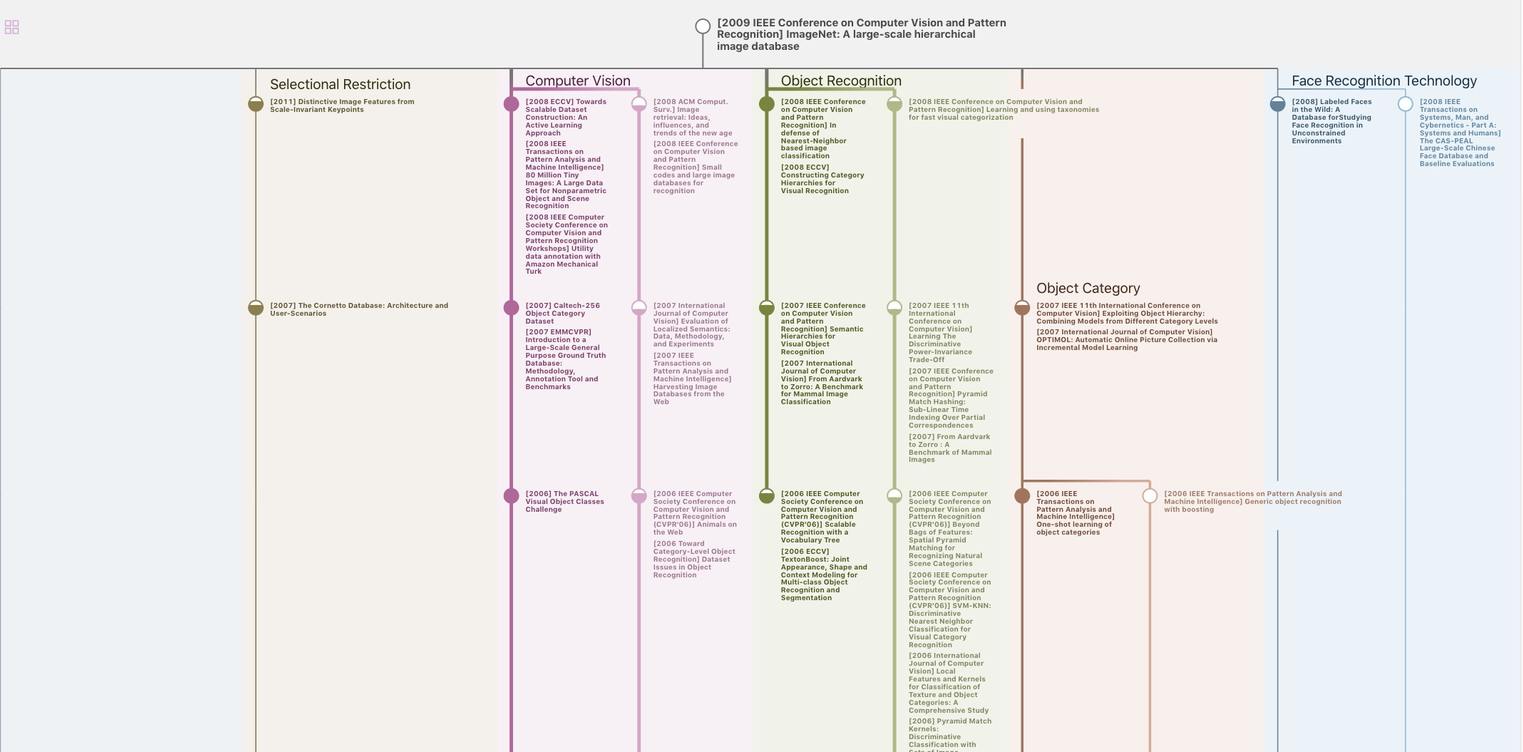
生成溯源树,研究论文发展脉络
Chat Paper
正在生成论文摘要