Incomplete Multi-view Clustering Based on Self-representation
NEURAL PROCESSING LETTERS(2023)
摘要
In recent years, multi-view spectral clustering has become a research hotspot of multi-view learning. However, it cannot directly handle incomplete multi-view data, while such data is very common in the real world. Besides, the adjacent graph is the key for multi-view spectral clustering and the artificial graph construction methods cannot obtain satisfactory performance. To solve these problems, this paper proposes a novel incomplete multi-view spectral clustering method called Incomplete Multi-view Clustering based on Self-representation (IMCS). To accurately represent the relation between data samples, IMCS constructs the adjacent graph of each view by self-representation. To obtain the consistency of multiple views, IMCS fuses the adjacent graphs from different views and utilizes the fused graph to perform spectral clustering. To reduce the negative effect of missing data, IMCS utilizes existing data to reconstruct incomplete data and integrates the reconstruction objective with self-representation based spectral clustering. IMCS is compared with several state-of-the-art multi-view clustering approaches on benchmark multi-view datasets. Experimental results demonstrate the superior effectiveness of IMCS.
更多查看译文
关键词
Multi-view clustering,Missing views,Spectral clustering,Self-representation
AI 理解论文
溯源树
样例
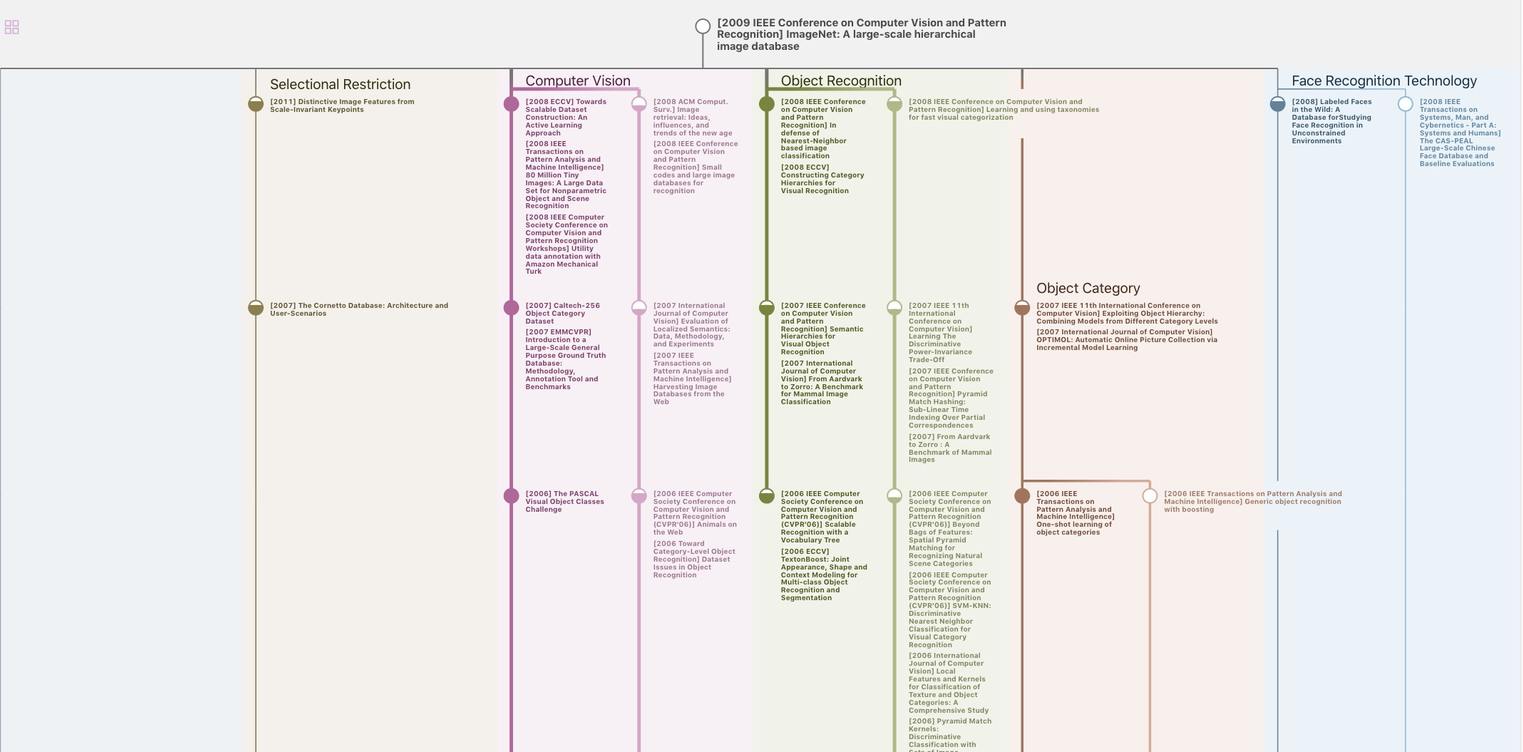
生成溯源树,研究论文发展脉络
Chat Paper
正在生成论文摘要