Deep Learning in Employee Selection: Evaluation of Algorithms to Automate the Scoring of Open-Ended Assessments
JOURNAL OF BUSINESS AND PSYCHOLOGY(2023)
摘要
This paper explores the application of deep learning in automating the scoring of open-ended candidate responses to pre-hire employment selection assessments. Using job applicant text data from pre-employment virtual assessment center exercises, three algorithmic approaches were compared: a traditional bag of words (BoW), long short-term memory (LSTM) models, and robustly optimized bidirectional encoder representations from transformers approach (RoBERTa). Measurement and assessment best practices were leveraged in the development of the candidate assessment items and human labels (subject matter experts’ (SME) ratings on job-relevant competencies), producing a rich set of data to train the algorithms on. The trained models were used to score the candidate textual responses on the given competencies, and the level of agreement with expert human raters was assessed. Using data from three companies hiring for three different occupations and across seven competencies, three algorithmic approaches to automatically score text were evaluated, showcasing correlations between SME and algorithmically scored competencies on holdout samples that were very strong (avg r = 0.84 for the best performing method, RoBERTa) and nearly identical to the inter-rater reliability achieved by multiple expert raters following consensus (avg r = 0.85). Criterion-related validity, subgroup differences, and decision accuracy are investigated for each algorithmic approach. Lastly, the impact of smaller sample sizes to train the algorithms is explored.
更多查看译文
关键词
Deep learning,Machine learning,Natural language processing,Measurement
AI 理解论文
溯源树
样例
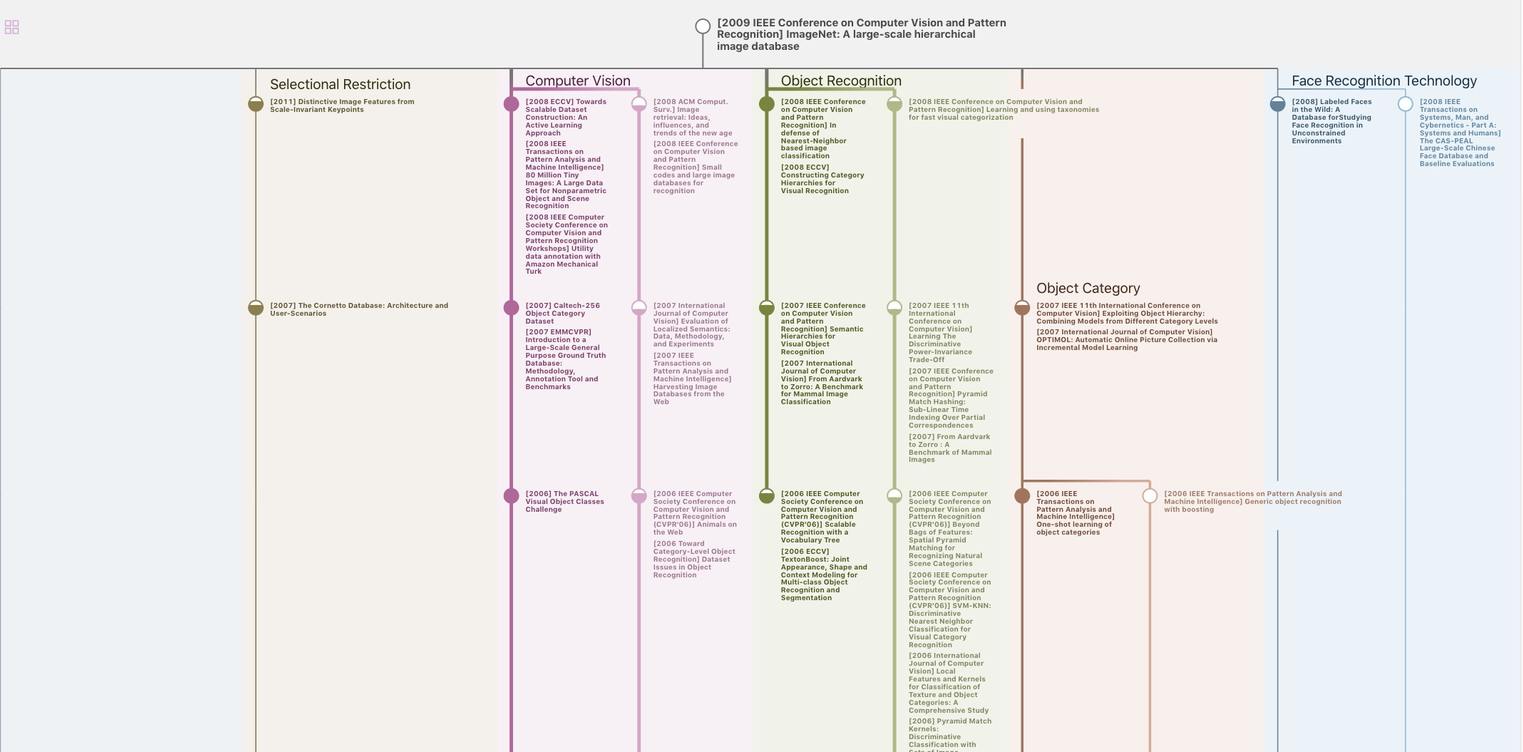
生成溯源树,研究论文发展脉络
Chat Paper
正在生成论文摘要