How to Be Irish in an Epidemic: A Dossier Article on HIV and AIDS in Ireland, Then and Now
MACHINE VISION AND APPLICATIONS(2023)
摘要
Person re-identification (ReID) is a computer vision-based autonomous process that re-identifies a query person from a set of gallery images captured by multiple non-overlapping cameras of a surveillance network. Since long, the convolution neural networks (CNNs) make strong baselines to solve vision problems. A limitation of CNN is the local neighborhood-dependent learning at each layer of the network, which prevents the learning of dependencies among distant regions of an image. However, these dependencies among distant parts of a person image play an imperative role in person ReID, especially in the cases of diverse inter-class and intra-class variations. In this study, we address this limitation of CNNs and provide a ubiquitous self-attention-based deep architecture for person re-identification, to learn the associations between all parts of an image, whether these are close by or far away. Secondly, in order to emphasize learning less attentive regions of the image, we expanded our work by providing an attention-based batch layoff module in our baseline model. The proposed unification of learning self-attentions among all parts of a person's image while keeping in line with the complete context resulted in the novel transformer-based ReID architecture. We evaluated the proposed ReID model on three public ReID benchmarks Market1501, DukeMTMC-ReID and MSMT-17 and computed the cumulative matching characteristics curve and mean average precision. The proposed ReID model consistently outperformed all CNN-based vanilla ReID models for all standard performance measures of person re-identification. The implementation and trained models are made publicly available at .
更多查看译文
关键词
Visual surveillance,Person re-identification,Self-attention,Image patches,Attention drop
AI 理解论文
溯源树
样例
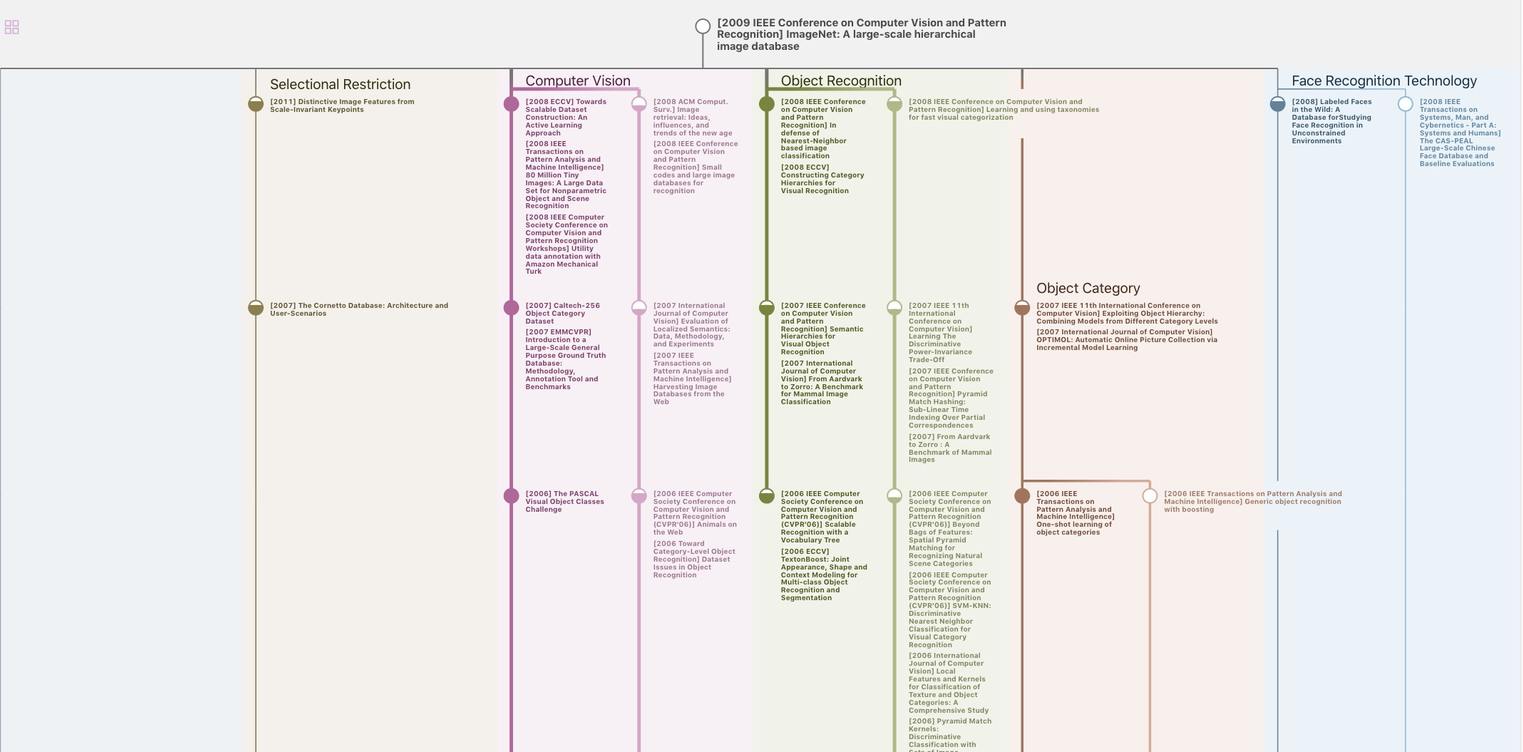
生成溯源树,研究论文发展脉络
Chat Paper
正在生成论文摘要