Multi-scale space-time transformer for driving behavior detection
MULTIMEDIA TOOLS AND APPLICATIONS(2023)
摘要
The advent of advanced in-vehicle sensors and communication technologies have facilitated the collection of large volume and almost real-time data on vehicles and drivers. Processing and analyzing this data provides unprecedented opportunities to offer remarkable insights and solutions for driving behavior detection. Characterizing driving behavior plays a key role in a variety of research areas such as traffic safety, the development of autonomous driving, and risk assessment. In this research, a novel framework, Multi-scale Space-time TRansformer (MSTR) is proposed for driving behavior detection using multi-modal data, i.e. front view video frames and vehicle signals. In particular, a multi-patch architecture is explored to capture driving scene features generated from different scales. Meanwhile, a Multi-patch Space-time Attention (MSA) module is designed for MSTR to model multi-scale features and capture spatial-temporal correlation simultaneously. Moreover, the extracted vehicle dynamics features are used as auxiliary to improve the robustness of detection, and a customized Cross-Modal Fusion (CMF) module is introduced to integrate these two different modality features efficiently. Finally, we experimentally validate the efficiency of our approach on a naturalistic driving data set containing over 2800 maneuvers recorded. The MSTR achieves state-of-the-art results with a low inference cost when compared to 3D convolutional networks, and it performs superior to a number of Transformer-based models and other advanced detection methods.
更多查看译文
关键词
Driving behavior detection,MSTR,Multi-modal data,Multi-patch space-time attention
AI 理解论文
溯源树
样例
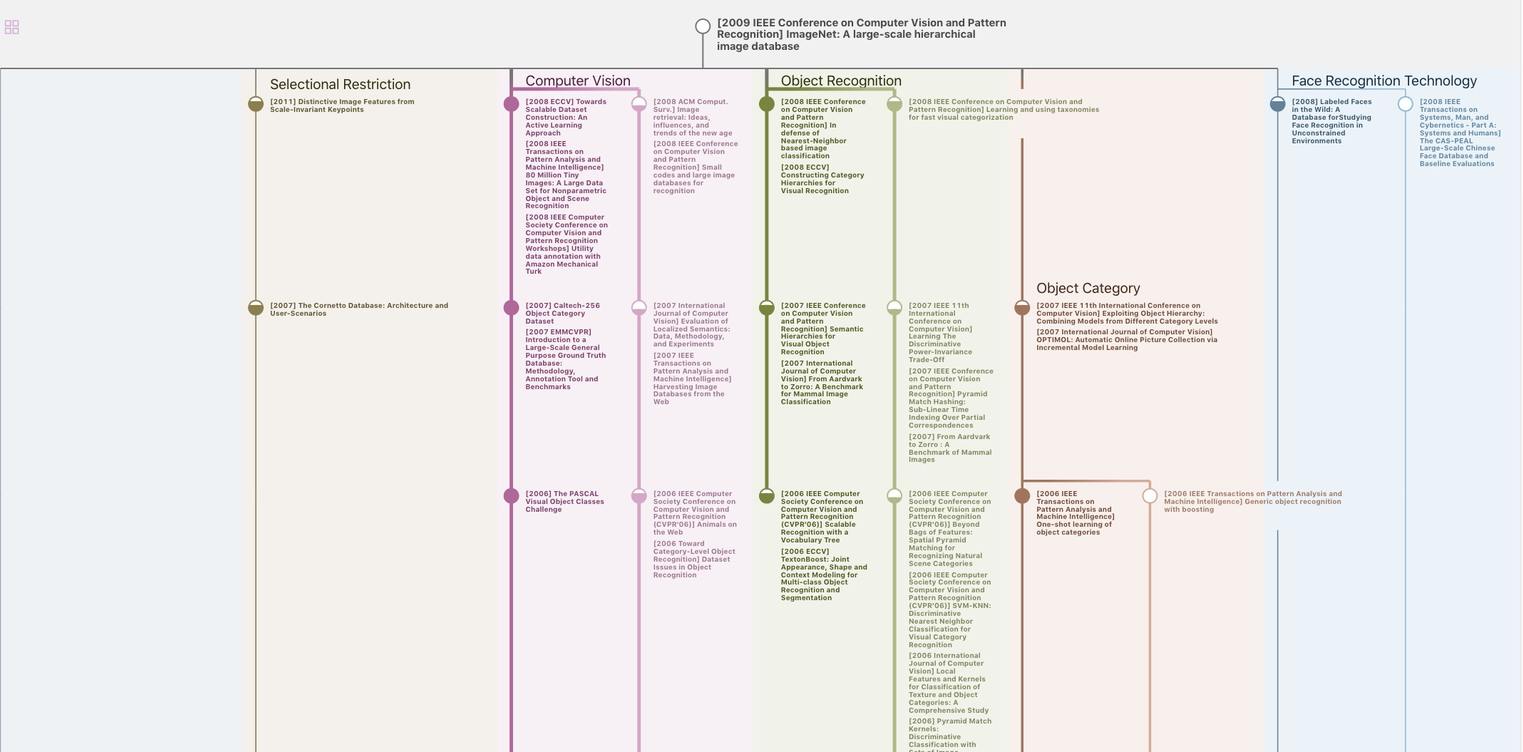
生成溯源树,研究论文发展脉络
Chat Paper
正在生成论文摘要