SA-SGRU: Combining Improved Self-Attention and Skip-GRU for Text Classification
APPLIED SCIENCES-BASEL(2023)
摘要
When reading texts for text classification tasks, a large number of words are irrelevant, and in text classification tasks, the traditional self-attention mechanism has the problem of weight distribution limitations. Therefore, a text classification model that combines an improved self-attention mechanism with a Skip-GRU (Skip-grate recurrent unit) network (SA-SGRU) is proposed in this paper. Firstly, Skip-GRU, the enhanced model of GRU (Grate Recurrent Unit), is used to skip the content that is not important for text classification when reading texts and only capture effective global information. Then, the improved self-attention mechanism is introduced to redistribute the weight of the deep text sequences. Secondly, the optimized CNN (convolutional neural network) is combined to bring up the local features of texts. Finally, a Softmax classifier is used to obtain the classification results of sample labels. Experimental results show that the proposed method can achieve better performance on three public datasets compared with other baseline methods. The ablation experiments also demonstrate the effectiveness of each module in the proposed model.
更多查看译文
关键词
text classification,skip gated recurrent unit,self-attention mechanism,convolutional neural network
AI 理解论文
溯源树
样例
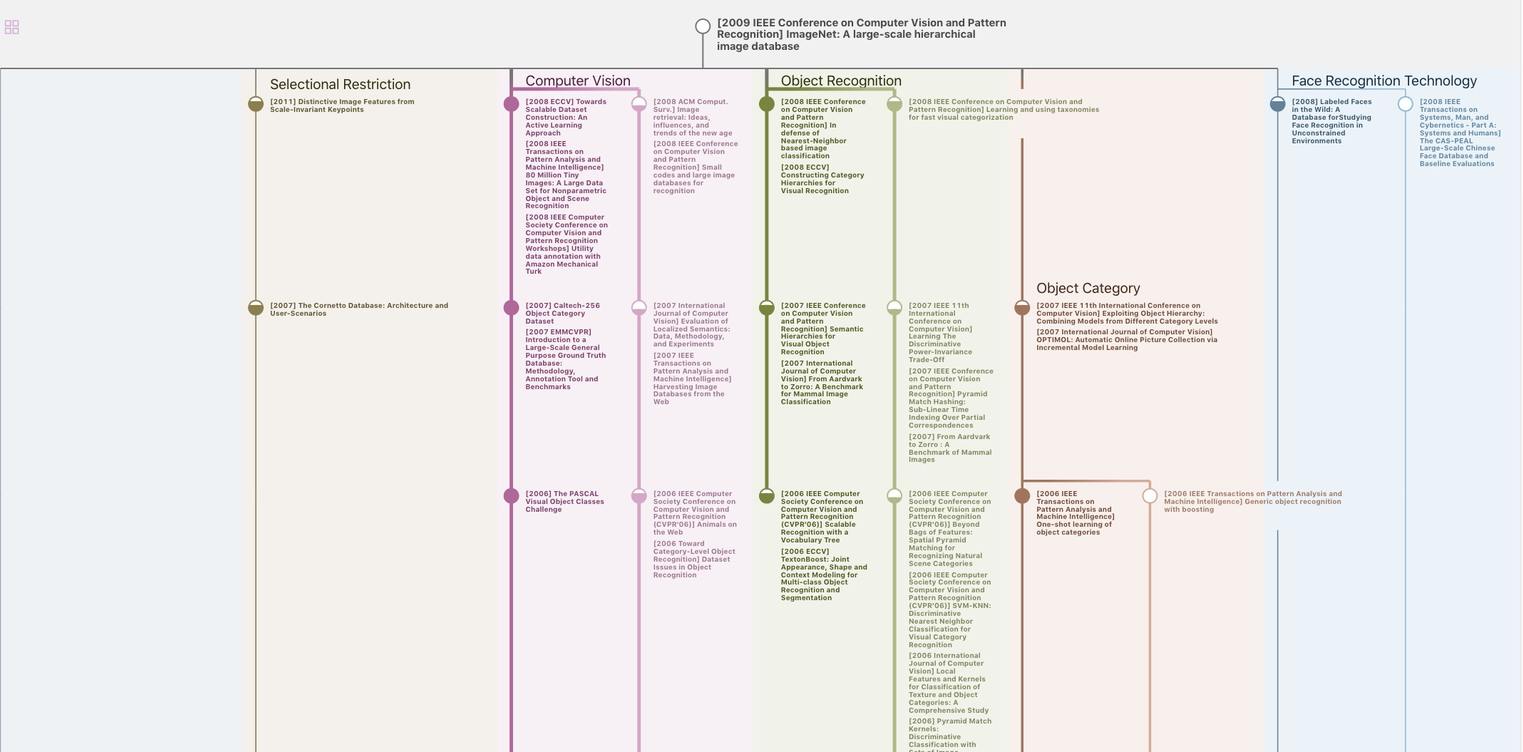
生成溯源树,研究论文发展脉络
Chat Paper
正在生成论文摘要