Parallel dual-channel multi-label feature selection
Soft Computing(2023)
摘要
In the process of multi-label learning, feature selection methods are often adopted to solve the high-dimensionality problem in feature spaces. Most existing multi-label feature selection algorithms focus on exploring the correlation between features and labels and then obtain the target feature subset by importance ranking. These algorithms commonly use single-channel structure to obtain important features, which induces the excessive reliance on the ranking results and causes the loss of important features. However, the correlation between label-specific feature and label-instance is ignored. Therefore, this paper proposes Parallel Dual-channel Multi-label Feature Selection algorithm (PDMFS). We first introduce the concept of dual channel and design the algorithm model as two independent modules. The algorithm obtained different feature correlation sequences, thus avoided relevant feature loss. And then, the proposed algorithm uses the subspace model to select the feature subset with the maximum correlation and minimum redundancy for each sequence, thus obtaining feature subsets under respective correlations. Finally, the subsets are cross-merged to reduce the important feature loss caused by the serial structure processing single feature correlation. The experimental results on eight datasets and statistical hypothesis testing indicate that the proposed algorithm is effective.
更多查看译文
关键词
Multi-label learning,Feature selection,Parallel dual-channel,Information entropy,Cross-merging
AI 理解论文
溯源树
样例
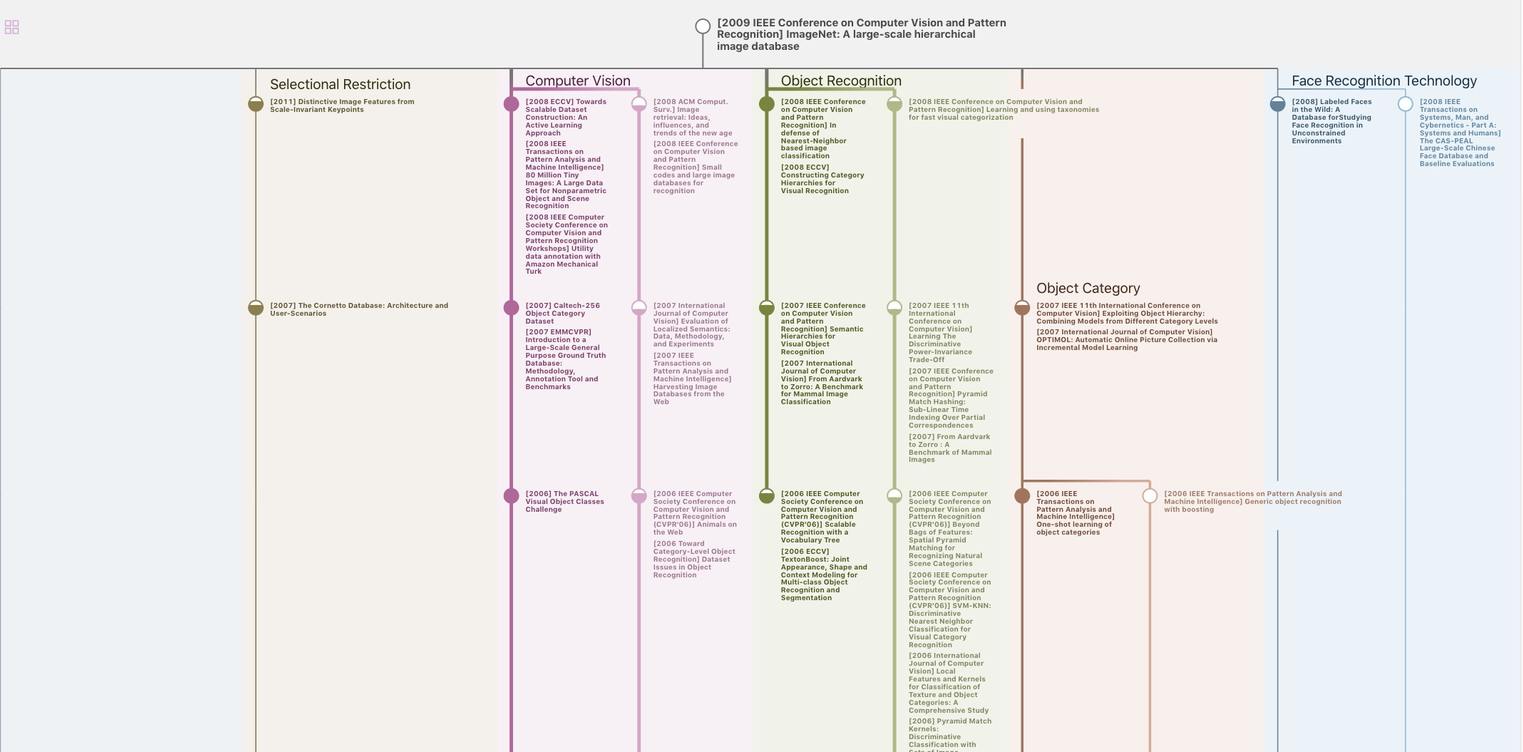
生成溯源树,研究论文发展脉络
Chat Paper
正在生成论文摘要