Achieving Personalized Precision Education Using the Catboost Model during the COVID-19 Lockdown Period in Pakistan
SUSTAINABILITY(2023)
摘要
With the emergence of the COVID-19 pandemic, access to physical education on campus became difficult for everyone. Therefore, students and universities have been compelled to transition from in-person to online education. During this pandemic, online education, the use of unfamiliar digital learning tools, the lack of internet access, and the communication barriers between teachers and students made precision education more difficult. Customizing models from previous studies that only consider a single course in order to make a prediction reduces the predictive power of the model because it only considers a small subset of the attributes of each possible course. Due to a lack of data for each course, overfitting often occurs. It is challenging to obtain a comprehensive understanding of the student's participation during the semester system or in a broader context. In this paper, a model that is flexible and more generalizable is developed to address these issues. This model resolves the problem of generalized models and overfitting by using a large number of responses from college and university students as a dataset that considered a broader range of attributes, regardless of course differences. CatBoost, an advanced type of gradient boosting algorithm, was used to conduct this research, and enabled the developed model to perform effectively and produce accurate results. The model achieved a 96.8% degree of accuracy. Finally, a comparison was made with other related work to demonstrate the concept, and the experimental results proved that the Catboost model is a viable, accurate predictor of students' performance.
更多查看译文
关键词
precision education,personalized learning,machine learning,early prediction,educational data mining,learning analytics,digital learning platforms,COVID-19
AI 理解论文
溯源树
样例
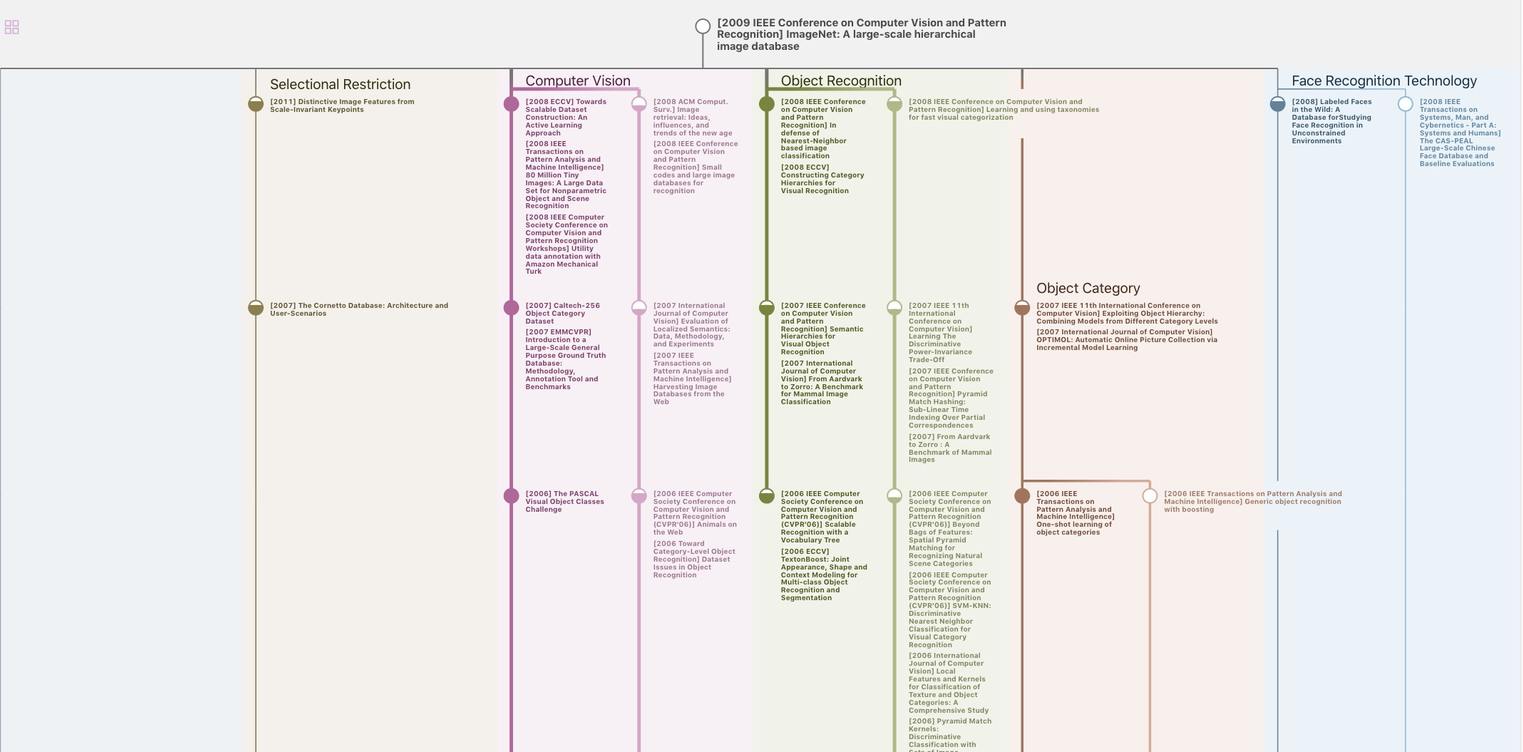
生成溯源树,研究论文发展脉络
Chat Paper
正在生成论文摘要