Empirical mode decomposition-based multi-scale spectral graph convolution network for abnormal electricity consumption detection
NEURAL COMPUTING & APPLICATIONS(2023)
Abstract
Abnormal electricity consumption detection is of great significance for maintaining the safe and stable operation of the power grids, or for reducing the irregular electricity consumption and economic losses. However, not enough attention has been paid to comprehensively explore the multi-scale characteristics of the consumption series, and to discover the intra-series relationships among different timestamps. To tackle such problems, this paper proposes an empirical mode decomposition-based multi-scale spectral graph convolution network for detecting the abnormal electricity consumptions. Firstly, the electricity consumption data are decomposed into subcomponents by the empirical mode decomposition to generate the multi-scale time characteristics, and then, the entropy feature vectors are extracted from such subcomponents and the original data. Furthermore, the feature fusion for the entropy feature vectors and the original data is realized by the multi-scale convolutional layers. Then, the spectral graph convolution network (SGCN) is employed to detect the abnormal electricity consumption and to output specific electricity consumption case. In this SGCN, both the inter-series and the intra-series relationships can be tackled by the graph Fourier transform and the graph convolution operator. Finally, detailed experiments and comparisons are done. Experimental results demonstrate that our proposed method has satisfactory accuracy and adaptability, and has much better detection performance than some popular deep and shallow methods, e.g., the CNN, LSTM, ELM and SVM.
MoreTranslated text
Key words
Abnormal electricity consumption detection,Empirical mode decomposition,Spectral graph convolution network
AI Read Science
Must-Reading Tree
Example
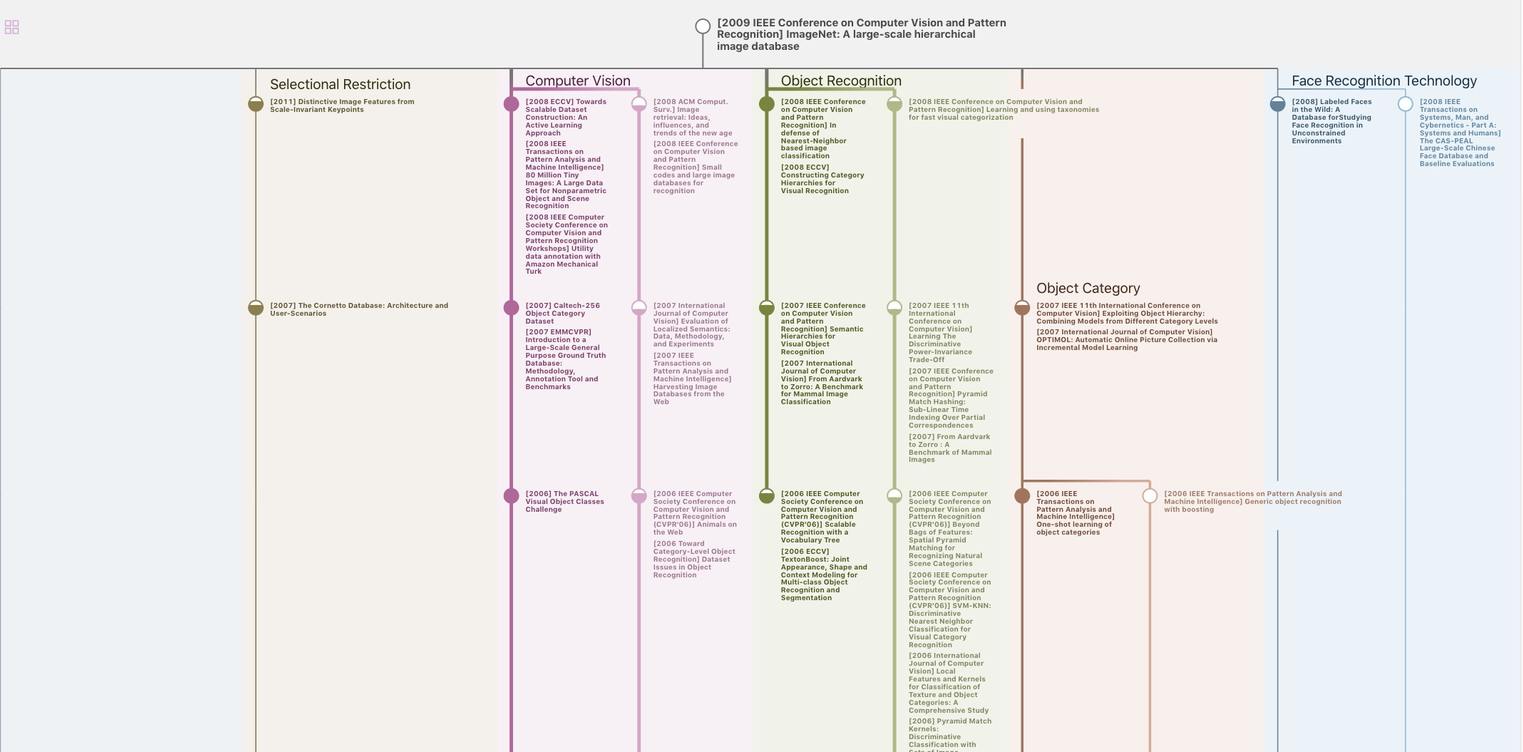
Generate MRT to find the research sequence of this paper
Chat Paper
Summary is being generated by the instructions you defined