Machine-Learning-Assisted Screening of Interface Passivation Materials for Perovskite Solar Cells
ACS ENERGY LETTERS(2023)
Abstract
Interface passivation using an ammonium salt can effectively improve the power conversion efficiency (PCE) of perovskite solar cells (PSCs). Despite significant PCE improve-ment achieved in previous studies, the selection criteria for ammonium salts are not fully understood. Here we apply a machine-learning (ML) method to investigate the relationship between the molecular features of ammonium salts and the PCE improvement of PSCs. We establish an ML model using an experimental data set of 19 salts to predict the PCE improve-ment after passivation. Three molecular features (hydrogen bond donor, hydrogen atom, and octane-water partition coefficient) are identified as the most important features of selecting an ammonium salt for passivation. The ML model is further used to screen ammonium salts from a pool of 112 salts in the PubChem database. FAMACs and FAMA-based PSCs fabricated with a model-recommended salt (2-phenylpropane-1-aminium iodide) achieve PCEs of 22.36% and 24.47%, respectively.
MoreTranslated text
Key words
interface passivation materials,solar cells,machine-learning-assisted machine-learning-assisted
AI Read Science
Must-Reading Tree
Example
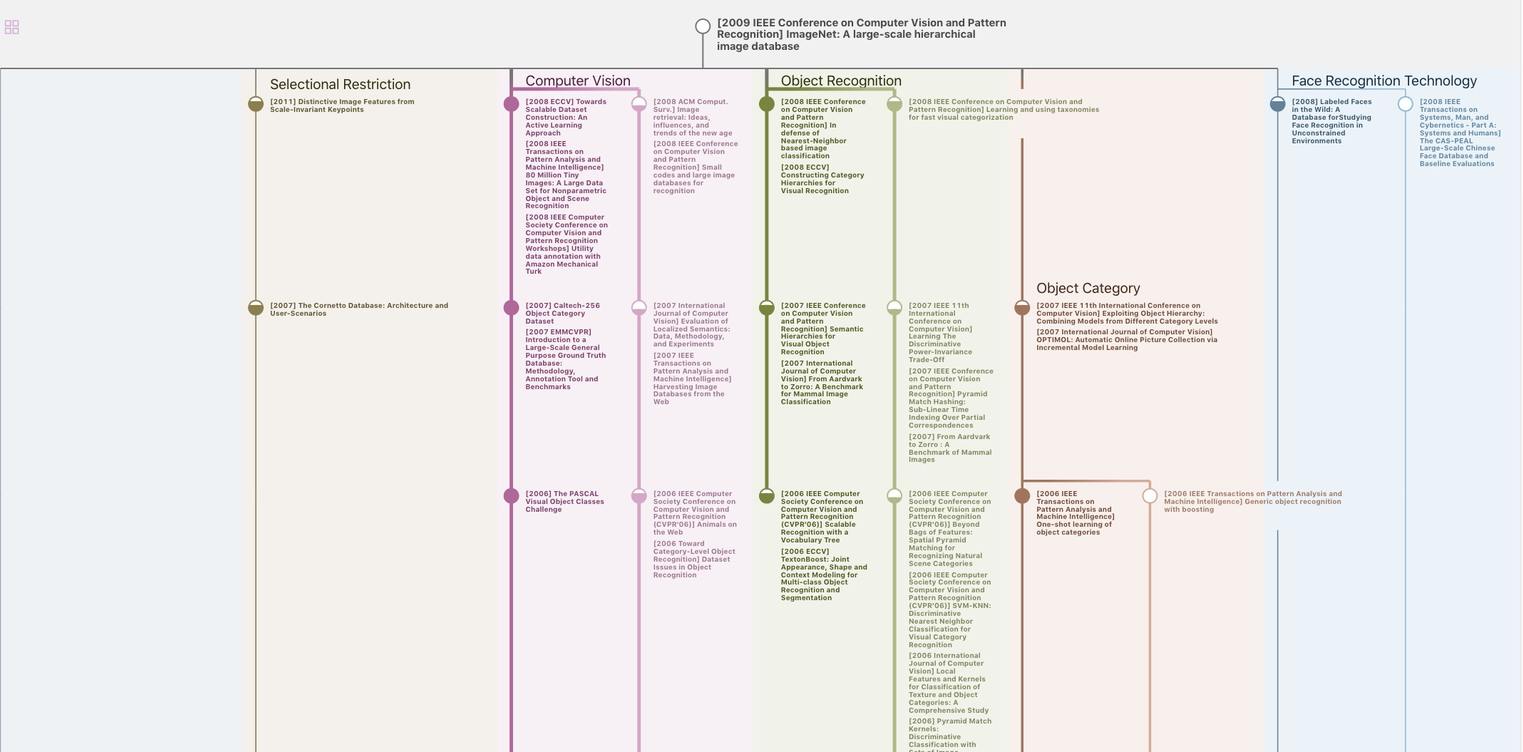
Generate MRT to find the research sequence of this paper
Chat Paper
Summary is being generated by the instructions you defined