A bi-level ensemble learning approach to complex time series forecasting: Taking exchange rates as an example
JOURNAL OF FORECASTING(2023)
摘要
Forecasting complex time series faces a huge challenge due to its high volatility. To improve the accuracy and robustness of prediction, this paper proposes a bi-level ensemble learning approach by combining decomposition-ensemble forecasting and resample strategies. The bi-level ensemble approach consists of four steps: data decomposition via singular spectrum analysis (SSA), resampling by employing a bagging algorithm, individual forecasting utilizing extreme learning machine (ELM), and introducing sorting-pruning and simple addition ensemble strategies for integrating the inner and outer results, respectively. To verify the effectiveness of the established forecasting approach, three exchange rate time series are selected as samples. The results reveal that the proposed model is significantly better than the other benchmarks at different lead times, which indicates that it can be regarded as an effective and promising tool for complex time series forecasting.
更多查看译文
关键词
bagging,bi-level ensemble forecasting,exchange rates forecasting,machine learning,singular spectrum analysis
AI 理解论文
溯源树
样例
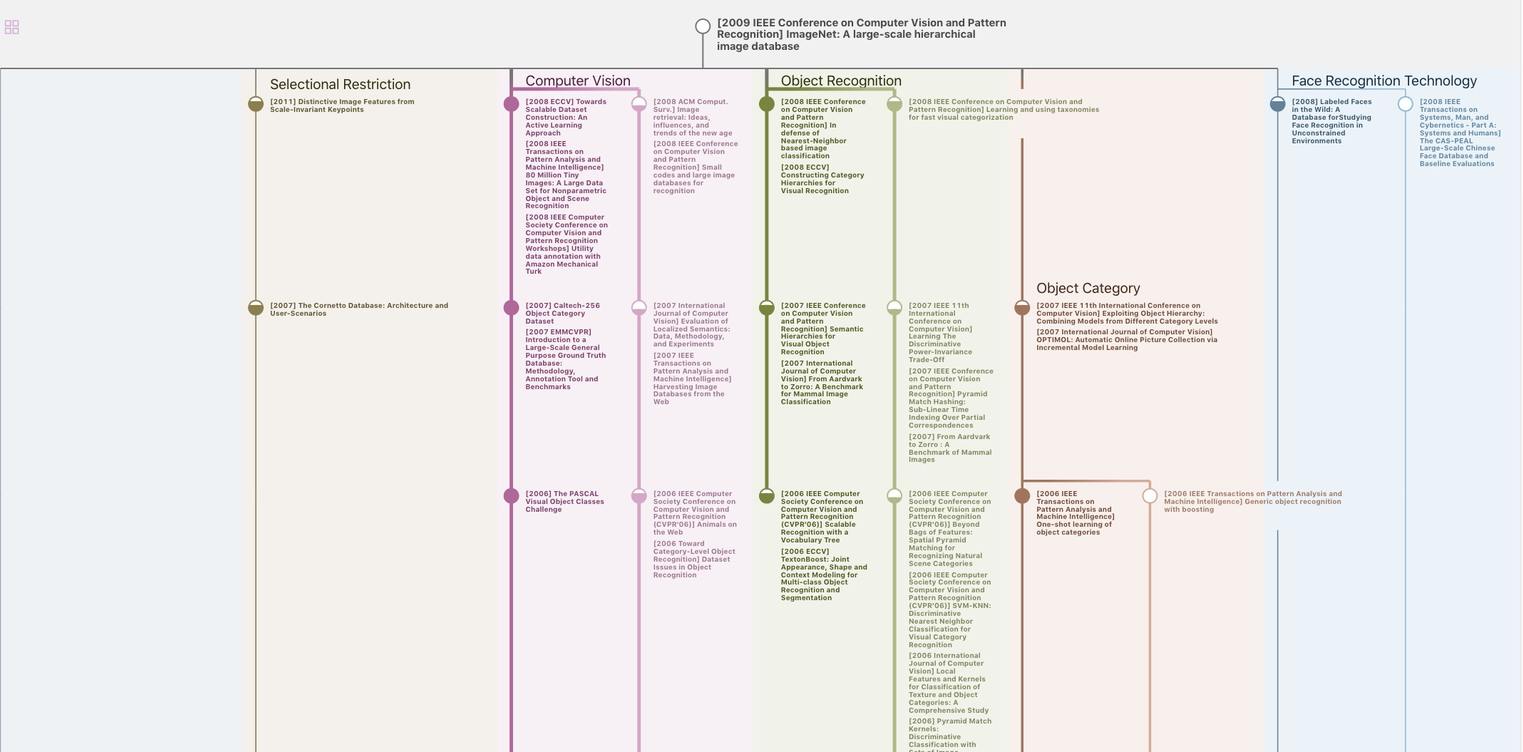
生成溯源树,研究论文发展脉络
Chat Paper
正在生成论文摘要