Improved milling stability analysis for chatter-free machining parameters planning using a multi-fidelity surrogate model and transfer learning with limited experimental data
INTERNATIONAL JOURNAL OF PRODUCTION RESEARCH(2024)
摘要
Decision-making on chatter-free machining parameters is essential for process planning since chatter significantly affects production quality and efficiency. Stability lobe diagram (SLD) is commonly used for selecting chatter-free machining parameters, but its analytical prediction often has poor accuracy and experiment-based prediction is time-consuming. This paper proposes a multi-fidelity (MF) surrogate model and transfer learning-based method to improve the milling stability analysis. Firstly, an analytical stability model is constructed to predict low-fidelity (LF) SLDs for key combinations of radial cutting width (a(e)) and feed rate per tooth (f(t)). A few spindle speeds (n(s)) are selected from each key LF SLD to detect high-fidelity (HF) stability limits (a(p)(lim)) through milling experiments. Subsequently, sufficient LF and limited HF combinations of n(s), a(e), f(t), and a(p)(lim) are taken to construct additive scaling function-based MF stability models. Predicted MF combinations of n(s), a(e), f(t), and a(p)(lim) are combined with limited HF combinations to construct more accurate stability models through transfer learning. Then, a neural network is ultimately trained to predict a(p)(lim) values for arbitrary combinations of n(s), a(e), and f(t). A detailed experimental validation indicates that the proposed method can provide more accurate lobe boundaries for machining parameters selection by introducing fewer experimental samples.
更多查看译文
关键词
Stability lobe diagram,machining parameters planning,multi-fidelity surrogate model,transfer learning,limited experimental information
AI 理解论文
溯源树
样例
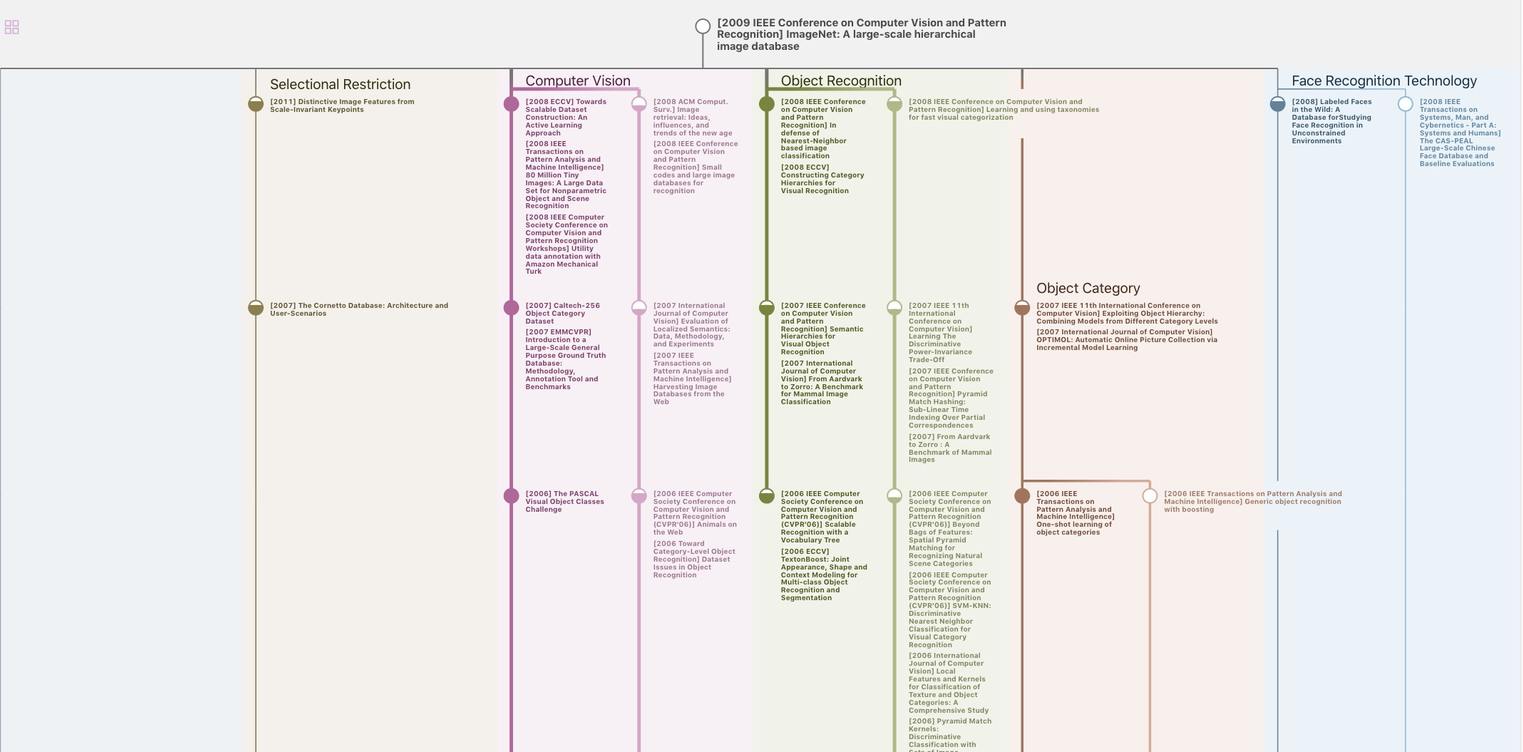
生成溯源树,研究论文发展脉络
Chat Paper
正在生成论文摘要