Short-Term Occupancy Forecasting for a Smart Home Using Optimized Weight Updates Based on GA and PSO Algorithms for an LSTM Network
ENERGIES(2023)
摘要
In this work, we provide a smart home occupancy prediction technique based on environ-mental variables such as CO2, noise, and relative temperature via our machine learning method and forecasting strategy. The proposed algorithms enhance the energy management system through the optimal use of the electric heating system. The Long Short-Term Memory (LSTM) neural network is a special deep learning strategy for processing time series prediction that has shown promising prediction results in recent years. To improve the performance of the LSTM algorithm, particularly for autocorrelation prediction, we will focus on optimizing weight updates using various approaches such as Genetic Algorithm (GA) and Particle Swarm Optimization (PSO). The performances of the proposed methods are evaluated using real available datasets. Test results reveal that the GA and the PSO can forecast the parameters with higher prediction fidelity compared to the LSTM networks. Indeed, all experimental predictions reached a range in their correlation coefficients between 99.16% and 99.97%, which proves the efficiency of the proposed approaches.
更多查看译文
关键词
deep neural networks,LSTM,time series prediction,optimisation,GA,PSO
AI 理解论文
溯源树
样例
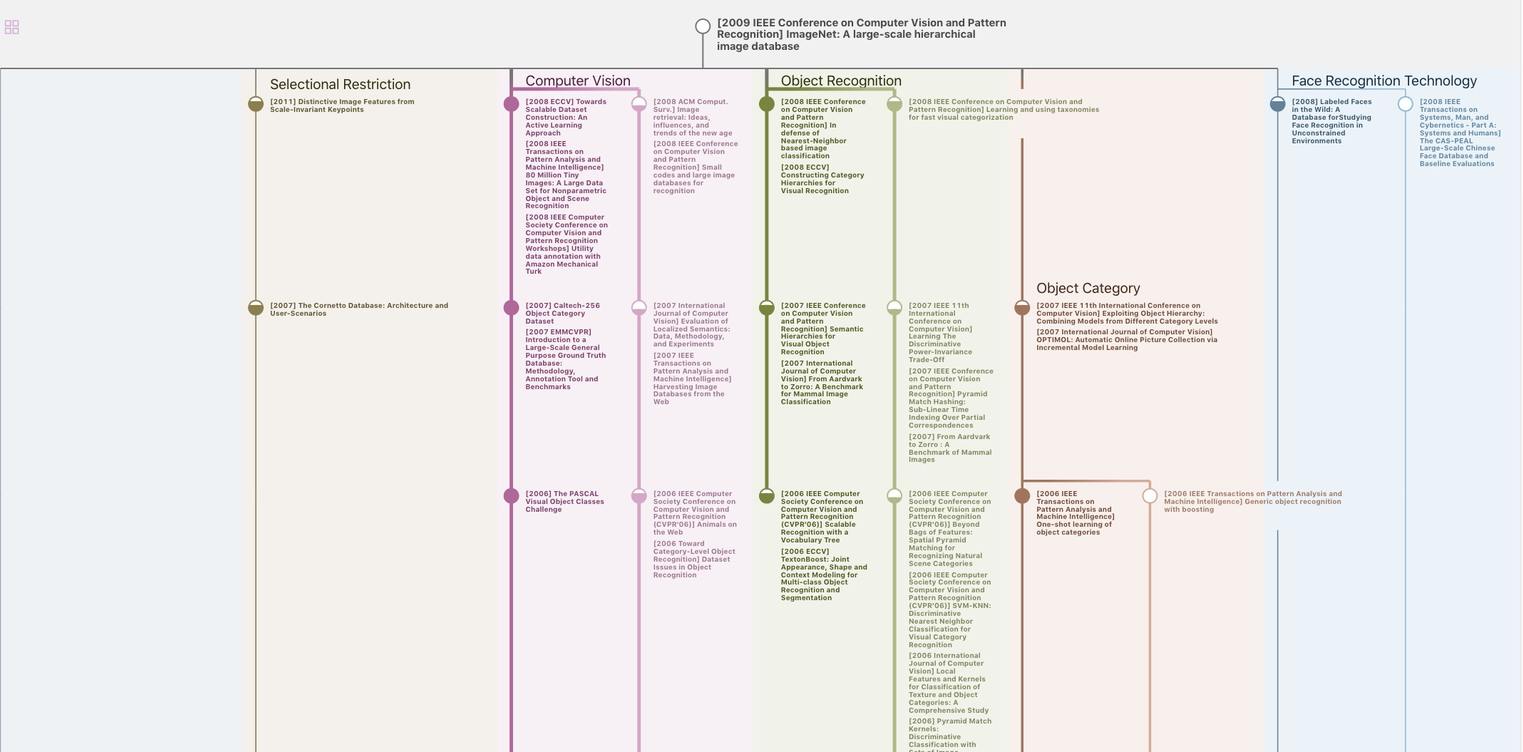
生成溯源树,研究论文发展脉络
Chat Paper
正在生成论文摘要