A Hybrid Neural Network Model for Short-Term Wind Speed Forecasting
ENERGIES(2023)
摘要
This study proposes an effective wind speed forecasting model combining a data processing strategy, neural network predictor, and parameter optimization method. (a) Variational mode decomposition (VMD) is adopted to decompose the wind speed data into multiple subseries where each subseries contains unique local characteristics, and all the subseries are converted into two-dimensional samples. (b) A gated recurrent unit (GRU) is sequentially modeled based on the obtained samples and makes the predictions for future wind speed. (c) The grid search with rolling cross-validation (GSRCV) is designed to simultaneously optimize the key parameters of VMD and GRU. To evaluate the effectiveness of the proposed VMD-GRU-GSRCV model, comparative experiments based on hourly wind speed data collected from the National Renewable Energy Laboratory are implemented. Numerical results show that the root mean square error, mean absolute error, mean absolute percentage error, and symmetric mean absolute percentage error of this proposed model reach 0.2047, 0.1435, 3.77%, and 3.74%, respectively, which outperform the benchmark predictions using popular parameter optimization methods, data processing techniques, and hybrid neural network forecasting models.
更多查看译文
关键词
wind speed forecasting,variational mode decomposition,gated recurrent unit,grid search,rolling cross-validation
AI 理解论文
溯源树
样例
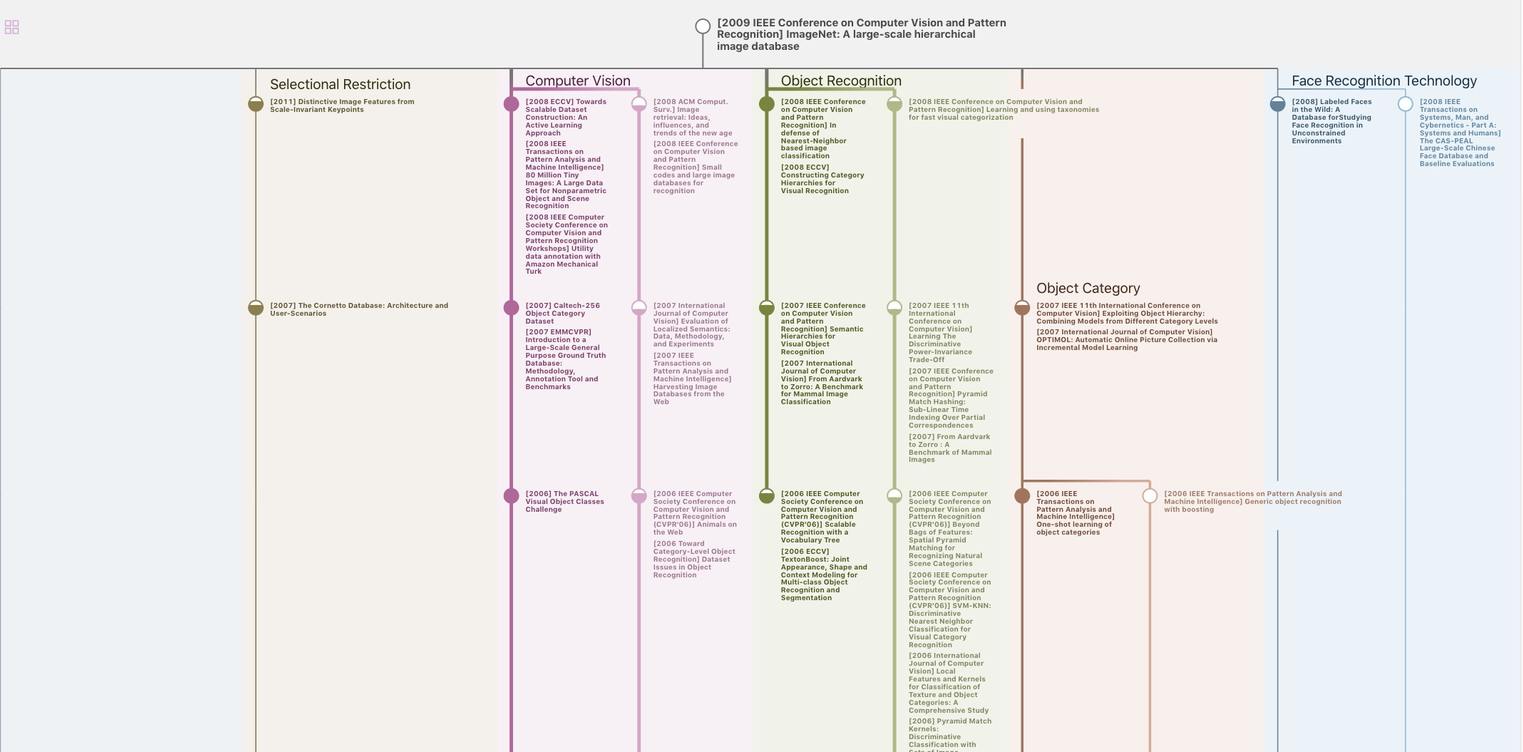
生成溯源树,研究论文发展脉络
Chat Paper
正在生成论文摘要