Semi-Supervised Land Cover Classification of Remote Sensing Imagery Using CycleGAN and EfficientNet
KSCE JOURNAL OF CIVIL ENGINEERING(2023)
摘要
Image classification of very high resolution (VHR) images is a fundamental task in the remote sensing domain for various applications, such as land cover mapping, vegetation mapping, and urban planning. Recently, deep learning-based semantic segmentation networks demonstrated the promising performance for pixel-level image classification. However, deep learning-based approaches are generally limited by the requirement of a sufficient amount of labeled data to obtain stable accuracy, and acquiring reference labels of remotely-sensed VHR images is very labor-extensive and expensive. Hence, this paper applied a semi-supervised learning-based CycleGAN and EfficientNet for VHR remote sensing image classification to overcome this problem. The proposed method achieved the highest accuracy than the other benchmarks. The largest increase in accuracy was observed in a test site containing complex objects due to the regularization effect of the semi-supervised method using unlabeled data. Moreover, results indicated that a relatively sufficient amount of unlabeled data compared with labeled data are required to increase the classification accuracy by controlling the amount of labeled and unlabeled data. Finally, we verified that the semi-supervised method returned significantly improved results irrespective of the three classification network structures, displaying the applicability of the method for semi-supervised image classification on remotely-sensed VHR images.
更多查看译文
关键词
CycleGAN,EfficientNet,Land cover classification,Remote sensing,Semi-supervised learning,VHR image classification
AI 理解论文
溯源树
样例
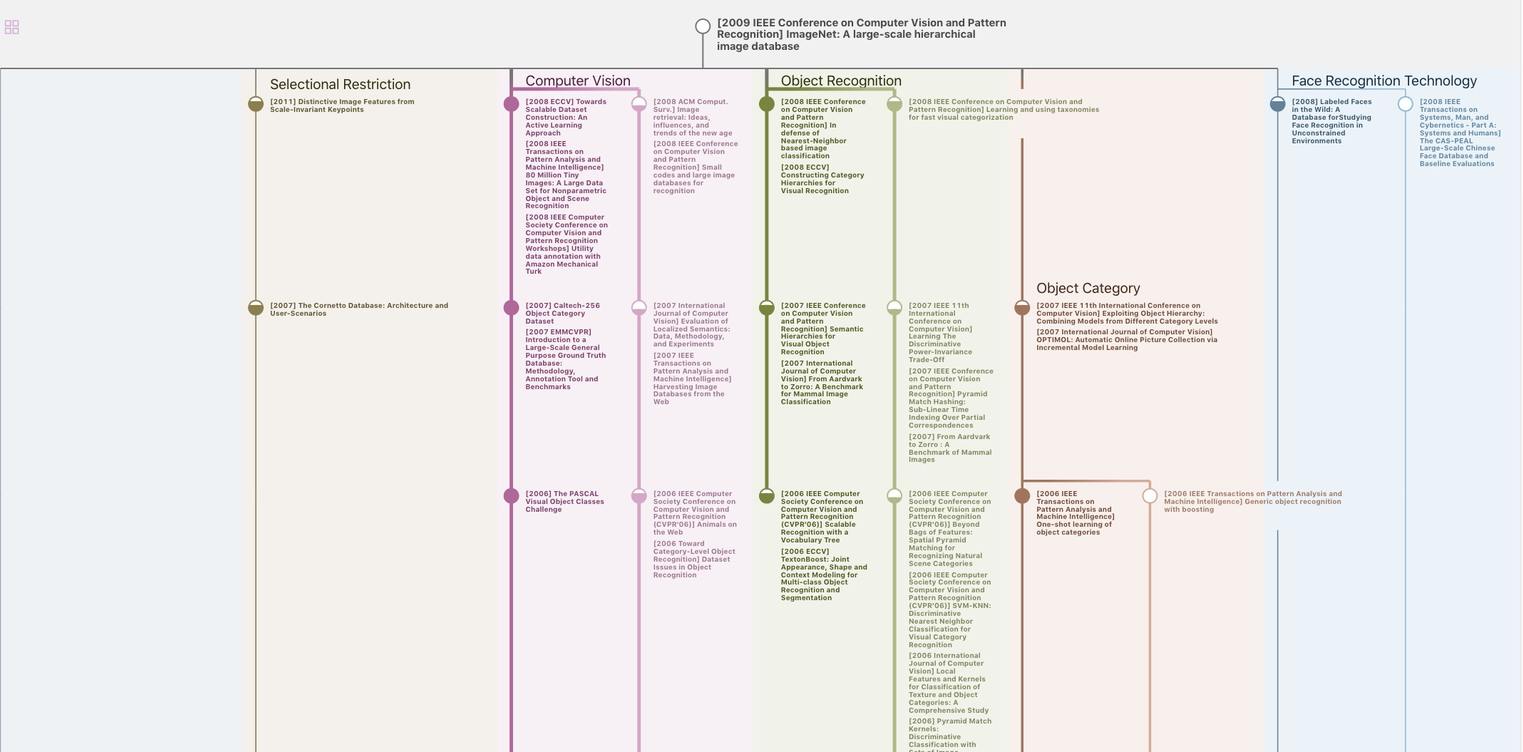
生成溯源树,研究论文发展脉络
Chat Paper
正在生成论文摘要