Semi-Supervised Adversarial Transfer Networks for Cross-Domain Intelligent Fault Diagnosis of Rolling Bearings
APPLIED SCIENCES-BASEL(2023)
摘要
In recent advances, deep learning-based methods have been broadly applied in fault diagnosis, while most existing studies assume that source domain and target domain data follow the same distribution. As differences in operating conditions lead to the deterioration of diagnosis performance, domain adaptation technology has been introduced to bridge the distribution gap. However, most existing approaches generally assume that source domain labels are available under all health conditions during training, which is incompatible with the actual industrial situation. To this end, this paper proposes a semi-supervised adversarial transfer networks for cross-domain intelligent fault diagnosis of rolling bearings. Firstly, the Gramian Angular Field method is introduced to convert time domain vibration signals into images. Secondly, a semi-supervised learning-based label generating module is designed to generate artificial labels for unlabeled images. Finally, the dynamic adversarial transfer network is proposed to extract the domain-invariant features of all signal images and provide reliable diagnosis results. Two case studies were conducted on public rolling bearing datasets to evaluate the diagnostic performance. An experiment under variable operating conditions and an experiment with different numbers of source domain labels were carried out to verify the generalization and robustness of the proposed approach, respectively. Experiment results demonstrate that the proposed method can achieve high diagnosis accuracy when dealing with cross-domain tasks with deficient source domain labels, which may be more feasible in engineering applications than conventional methodologies.
更多查看译文
关键词
intelligent fault diagnosis,domain adaptation,semi-supervised learning,adversarial transfer network,rolling bearings
AI 理解论文
溯源树
样例
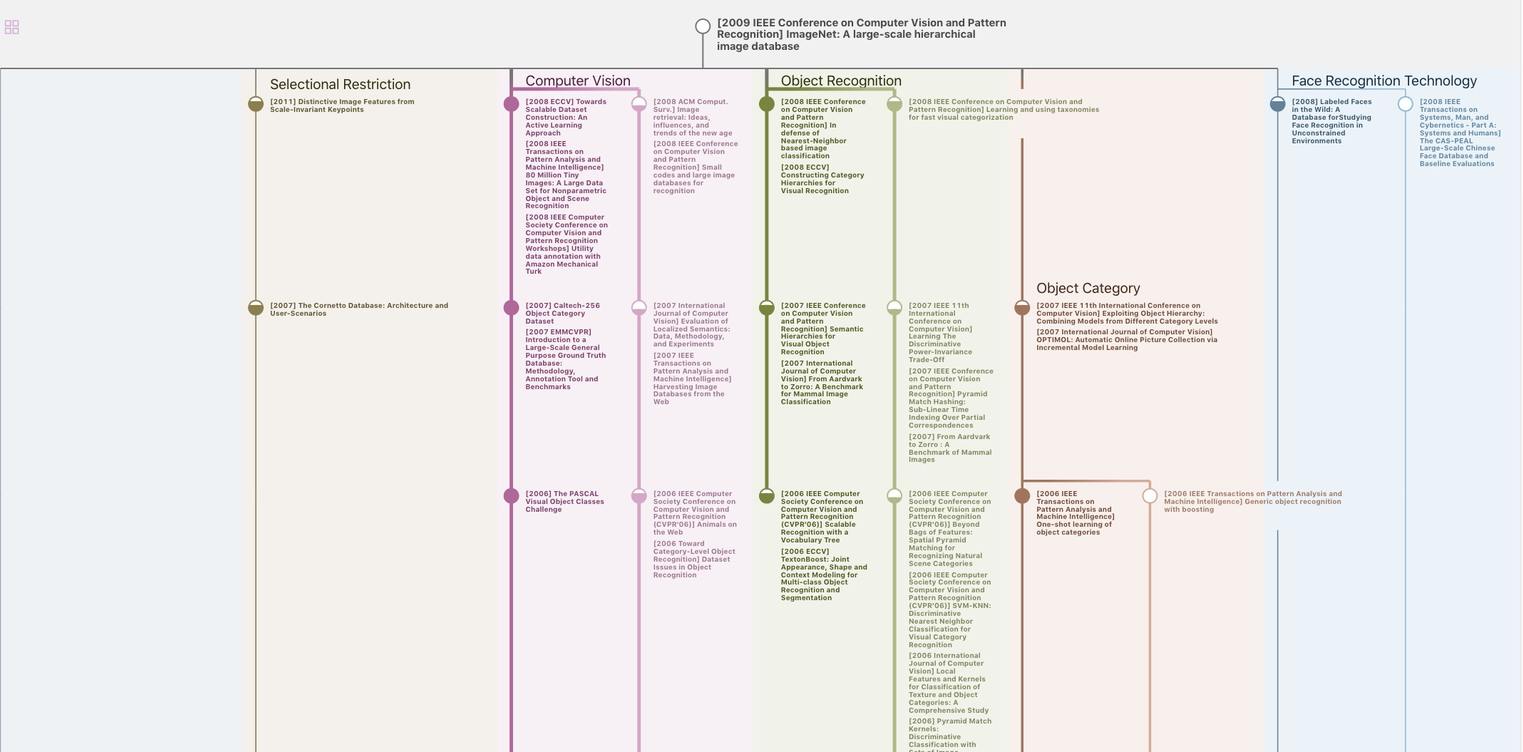
生成溯源树,研究论文发展脉络
Chat Paper
正在生成论文摘要