Data quality enhancement for field experiments in atmospheric chemistry via sequential Monte Carlo filters
ATMOSPHERIC MEASUREMENT TECHNIQUES(2023)
摘要
In this study, we explore the applications and limitations of sequential Monte Carlo (SMC) filters to field experiments in atmospheric chemistry. The proposed algorithm is simple, fast, versatile and returns a complete probability distribution. It combines information from measurements with known system dynamics to decrease the uncertainty of measured variables. The method shows high potential to increase data coverage, precision and even possibilities to infer unmeasured variables. We extend the original SMC algorithm with an activity variable that gates the proposed reactions. This extension makes the algorithm more robust when dynamical processes not considered in the calculation dominate and the information provided via measurements is limited. The activity variable also provides a quantitative measure of the dominant processes. Free parameters of the algorithm and their effect on the SMC result are analyzed. The algorithm reacts very sensitively to the estimated speed of stochastic variation. We provide a scheme to choose this value appropriately. In a simulation study, O-3, NO, NO2 and j(NO2) are tested for interpolation and de-noising using measurement data of a field campaign. Generally, the SMC method performs well under most conditions, with some dependence on the particular variable being analyzed.
更多查看译文
关键词
atmospheric chemistry,data quality enhancement,field experiments
AI 理解论文
溯源树
样例
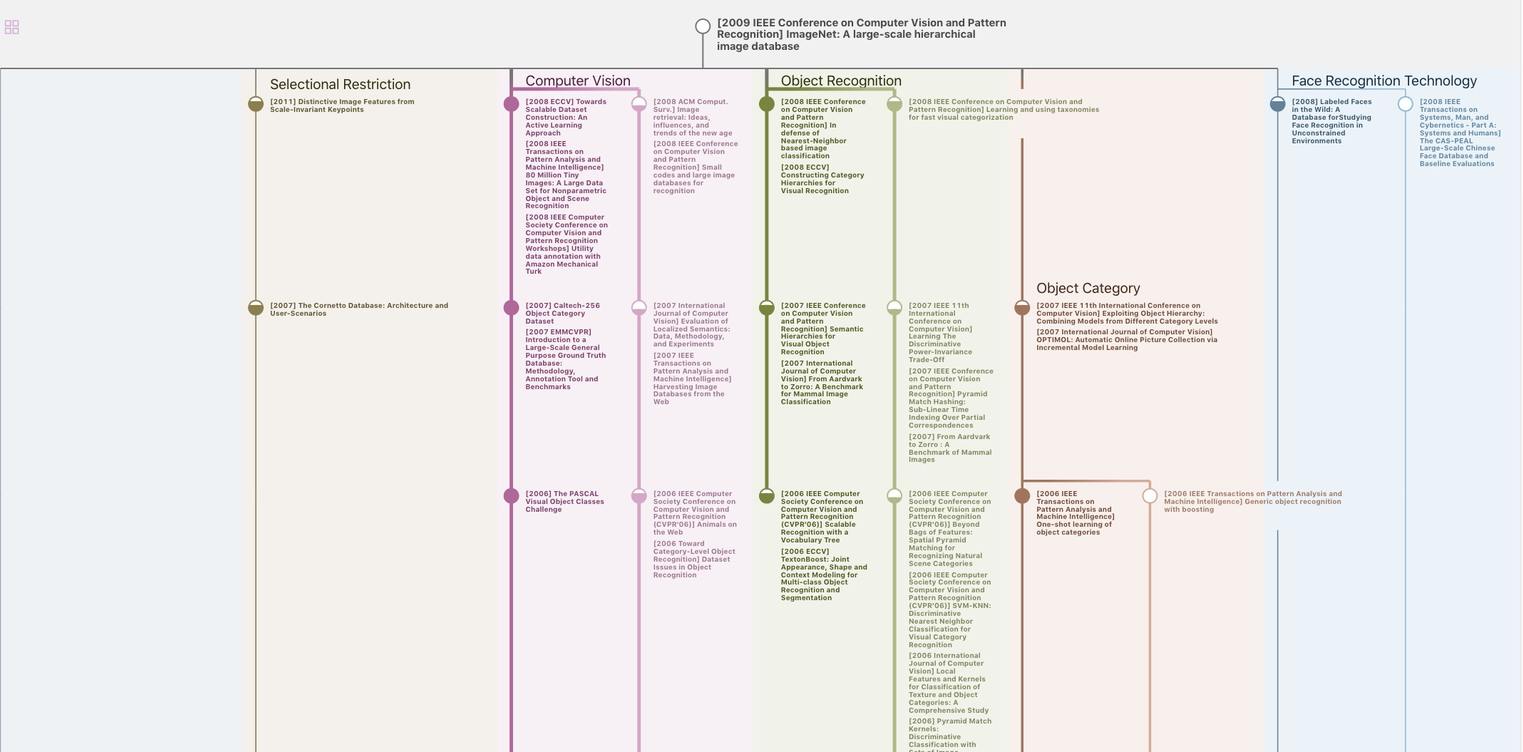
生成溯源树,研究论文发展脉络
Chat Paper
正在生成论文摘要