OISVM: Optimal Incremental Support Vector Machine-based EEG Classification for Brain-computer Interface Model
COGNITIVE COMPUTATION(2023)
摘要
The brain-computer interface (BCI) is a field of computer science where users can interact with devices in terms of brain signals. The brain signals are mimicked from the motor cortex without straining those muscles and without visualizing motor activity. By inserting various electrodes in the subject’s head, electroencephalography (EEG) is a technique used to capture the electrical patterns produced by the brain. The primary function of the EEG-based BCI is to track neural activity in the brain and convert it into signals or decisions. This paper presents a capuchin search algorithm (CSA)-optimized incremental support vector machine (ISVM) for the interpretation and classification of EEG-based BCI. The CSA is mainly incorporated to optimize the penalty and kernel parameters of the ISVM algorithm. The main aim of this paper is to aid in improving the interaction of stroke patients via computers by monitoring their thoughts. The features (electric signals), which contribute more to the output variable (hand movements), are identified via the sparse principal component analysis (SPCA) method. The proposed model is mainly trained and tested using the Berlin brain-computer interface (BCI) competition III datasets 4a and BCI competition IV datasets. The efficiency of the proposed model in analyzing the cortical sources is identified via different performance metrics such as accuracy, precision, F-score, etc. The proposed model offers accuracy, F1-score, and recall values of 92.24, 93.12, and 93.35%, respectively, which shows its efficiency in identifying hand, foot, or tongue movements.
更多查看译文
关键词
Brain-computer interface,Motor imagery,Sensory motor rhythm,Capuchin search algorithm,Incremental support vector machine,Sparse principal component analysis
AI 理解论文
溯源树
样例
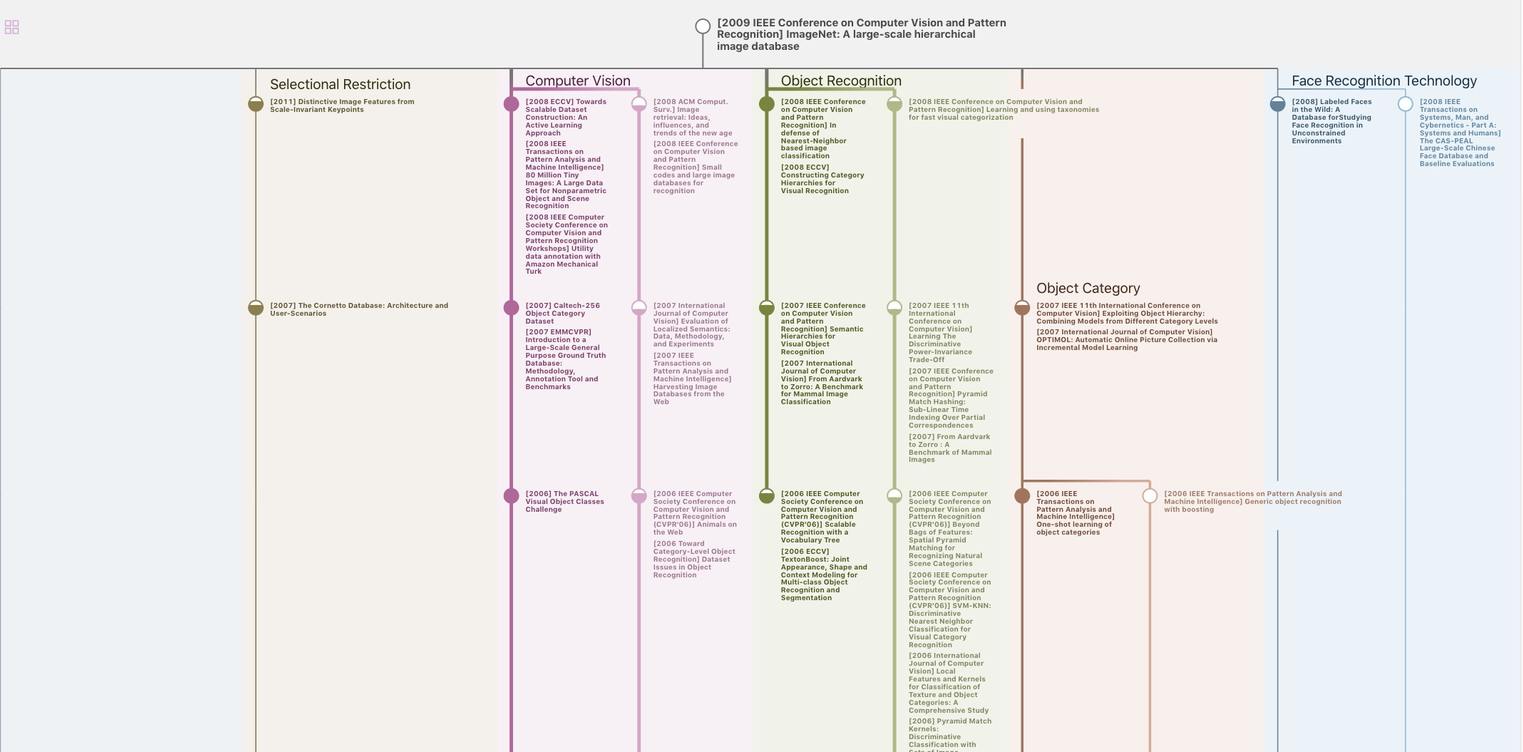
生成溯源树,研究论文发展脉络
Chat Paper
正在生成论文摘要