Machine learning approach to residential valuation: a convolutional neural network model for geographic variation
The Annals of Regional Science(2024)
摘要
Geographic location and neighbourhood attributes are major contributors to residential property values. Automated valuation models (AVM) often use hedonic pricing with location and neighbourhood attributes in the form of numeric and categorical variables. This paper proposed a novel approach to automated property valuation using a machine learning model with a convolutional neural network (CNN), fully connected neural network layers with numeric and categorical variables. In this study we compare the results of a fused model, which treat geographical data as an input with the performance of the baseline neural network model with only numerically or categorically represented data. Furthermore, the residential valuation by the proposed fused model was tested with actual sold price data in Greater Sydney, Australia. The study found that the fused model produced valuations with a significantly lower mean absolute percentage error (MAPE) (8.71%) than the MAPE of the baseline model (11.59%). The results show that the fused model with CNN significantly improves the accuracy for residential valuation, reducing spatial information loss by data manipulation and distance calibration.
更多查看译文
关键词
C31,O18,C31,R32
AI 理解论文
溯源树
样例
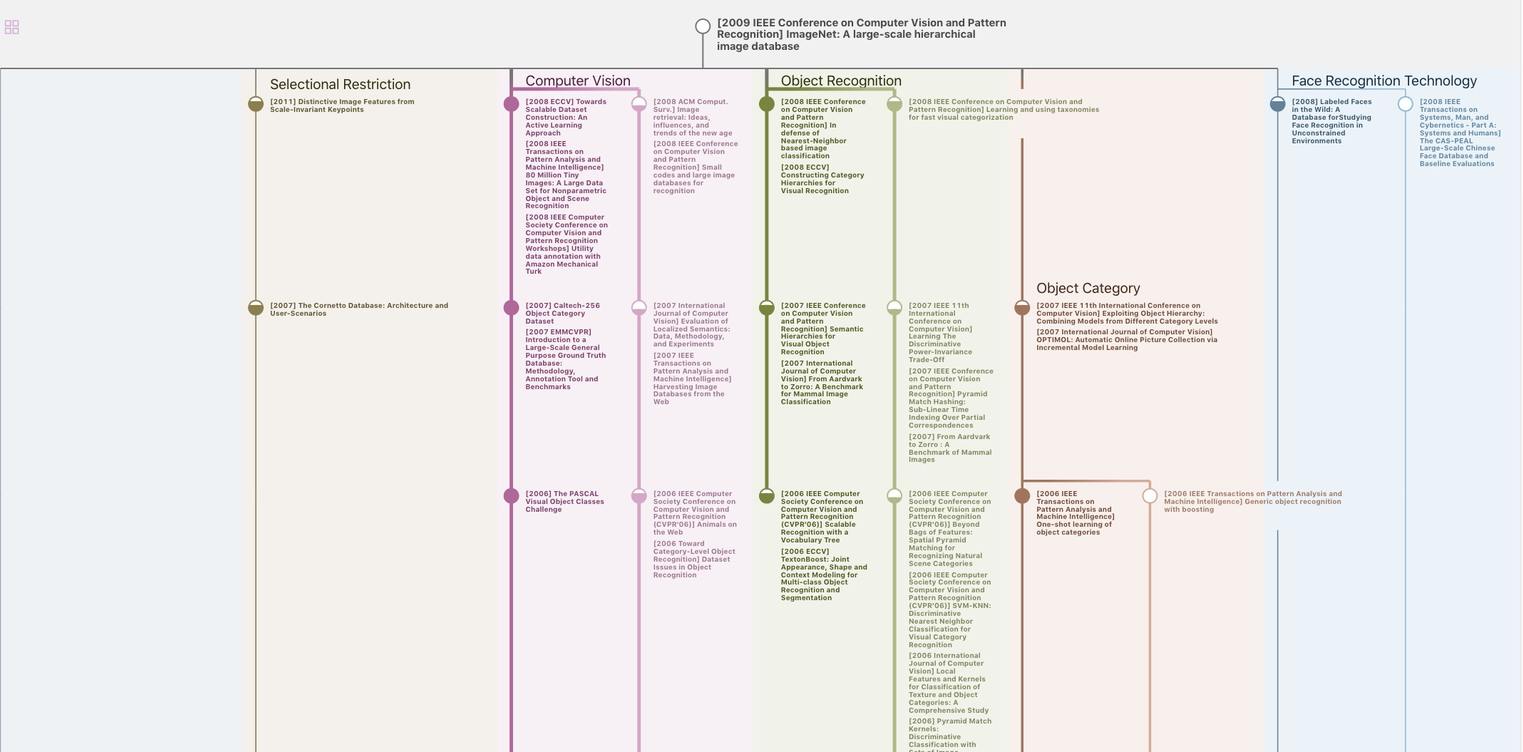
生成溯源树,研究论文发展脉络
Chat Paper
正在生成论文摘要