Neural coordination through spider monkey optimization-guided weight synchronization
MULTIMEDIA TOOLS AND APPLICATIONS(2023)
摘要
This paper proposes a Spider Monkey-based neural weight optimization approach for quicker neural synchronization. To exchange the session key across a public channel, two Artificial Neural Networks (ANNs) are synchronized through reciprocating learning. The most crucial feature of ANN coordination is evaluating how effectively sender and receiver’s ANNs coordinate without the other’s weights. Traditional algorithms have a latency in assessing coordination, which endangers neuronal coordination’s confidentiality. This research proposes a collaborative learning technique for analyzing the total synchronization of ANNs efficiently and decisively. The frequency at which the two ANNs got the same outcome in previous iterations determines synchronization. The hash is being used to evaluate if all ANNs are better aligned when a certain threshold is met. The presented technique uses the hash value of the synaptic weights to achieve complete synchronization among two communicating parties. This approach offers a number of benefits, namely (1) the usage of a Spider Monkey-based PRNG optimization to obtain the optimal weight vector. (2) The suggested method, in contrast to earlier methodologies, allows two communicating entities to discover complete collaboration more rapidly. (3) The probability of success is lowered geometrically. (4) The proposed TLTPM coordination periods are significantly quicker than the present CVTPM, VVTPM, and TPM-FPGA approaches. The suggested method improves the security of the neural key exchange protocol. The proposed approach is tested, and the findings reveal that it outperforms similar techniques currently in use.
更多查看译文
关键词
Spider Monkey Optimization (SMO),Neural synchronization,Hash Function,Session Key
AI 理解论文
溯源树
样例
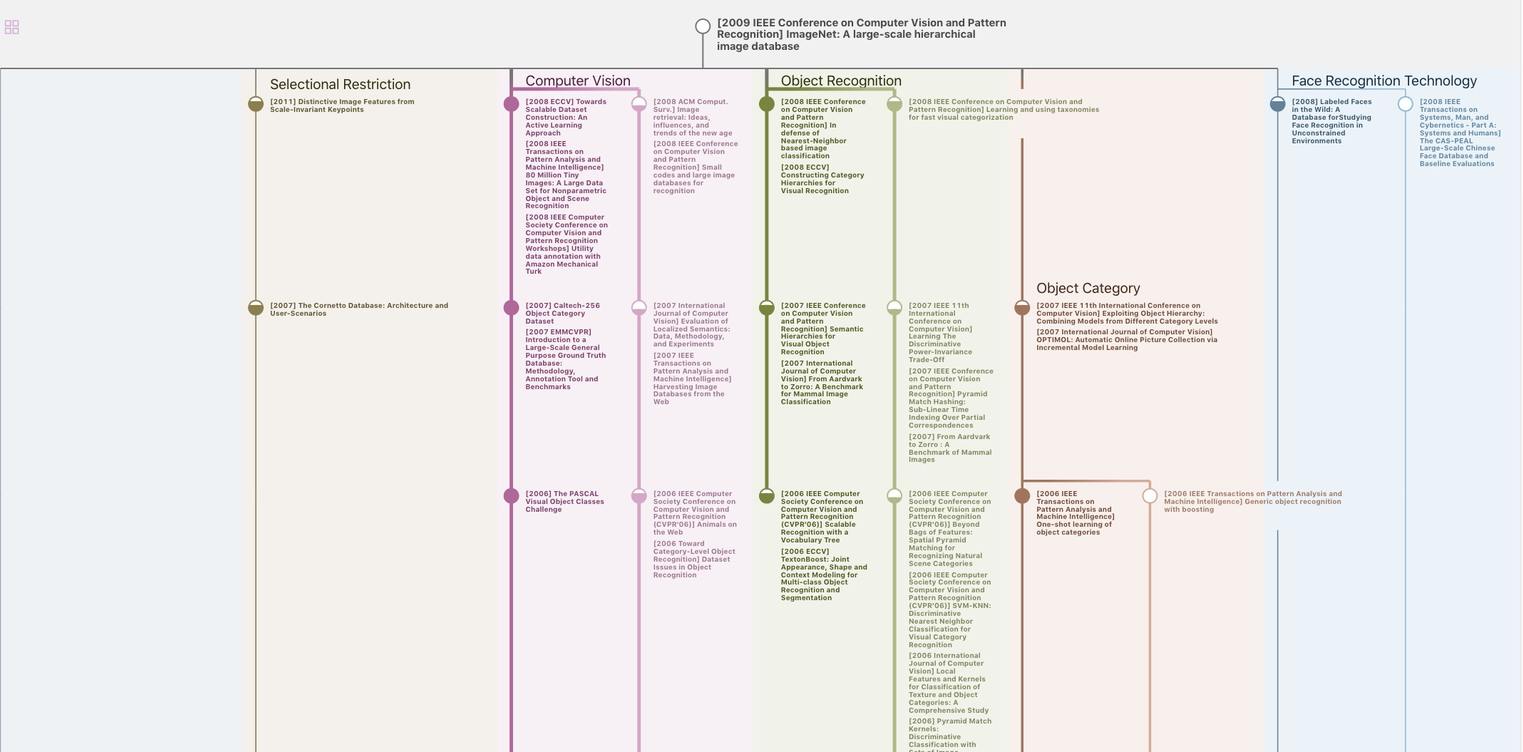
生成溯源树,研究论文发展脉络
Chat Paper
正在生成论文摘要