Landslide susceptibility mapping using automatically constructed CNN architectures with pre-slide topographic DEM of deep-seated catastrophic landslides caused by Typhoon Talas
NATURAL HAZARDS(2023)
摘要
There has been an increasing demand for detailed and accurate landslide maps and inventories in disaster-prone areas of subtropical and temperate zones, particularly in Asia as they can mitigate the impacts of landslides on social infrastructure and economic losses. Hence, in this study, models using automatically constructed high-performing convolutional neural network (CNN) architectures for landslide detection were applied and their outcomes were compared for landslide susceptibility mapping at the Kii peninsula, Japan. First, a total of 38 landslide and 63 non-landslide points were identified and divided into 70% and 30% of training and validation datasets, respectively. Eight landslide influence factors were used: slope angle, eigenvalue ratio, curvature, underground openness, overground openness, topographic witness index, wavelet, and elevation. These factors were selected using a 1-m DEM, which is easy to acquire and process data. Experimental results of model evaluation using receiver operating characteristics (ROC), area under the curve (AUC), and accuracy showed that the optimal models (ROC = 96.0%, accuracy = 88.7%) were more accurate than initial models (ROC = 91.1%, accuracy = 80.7%) in predicting landslides spatially. Furthermore, the landslide susceptibility mapping is consistent with the trends in the distribution of gentle slopes and knick lines unique to the study area and can be used as a powerful method for predicting landslides in future.
更多查看译文
关键词
Convolutional neural network,Landslide susceptibility map,Automatically constructed model,Landslide,Deep-seated gravitational slope deformation,Eigenvalue ratio
AI 理解论文
溯源树
样例
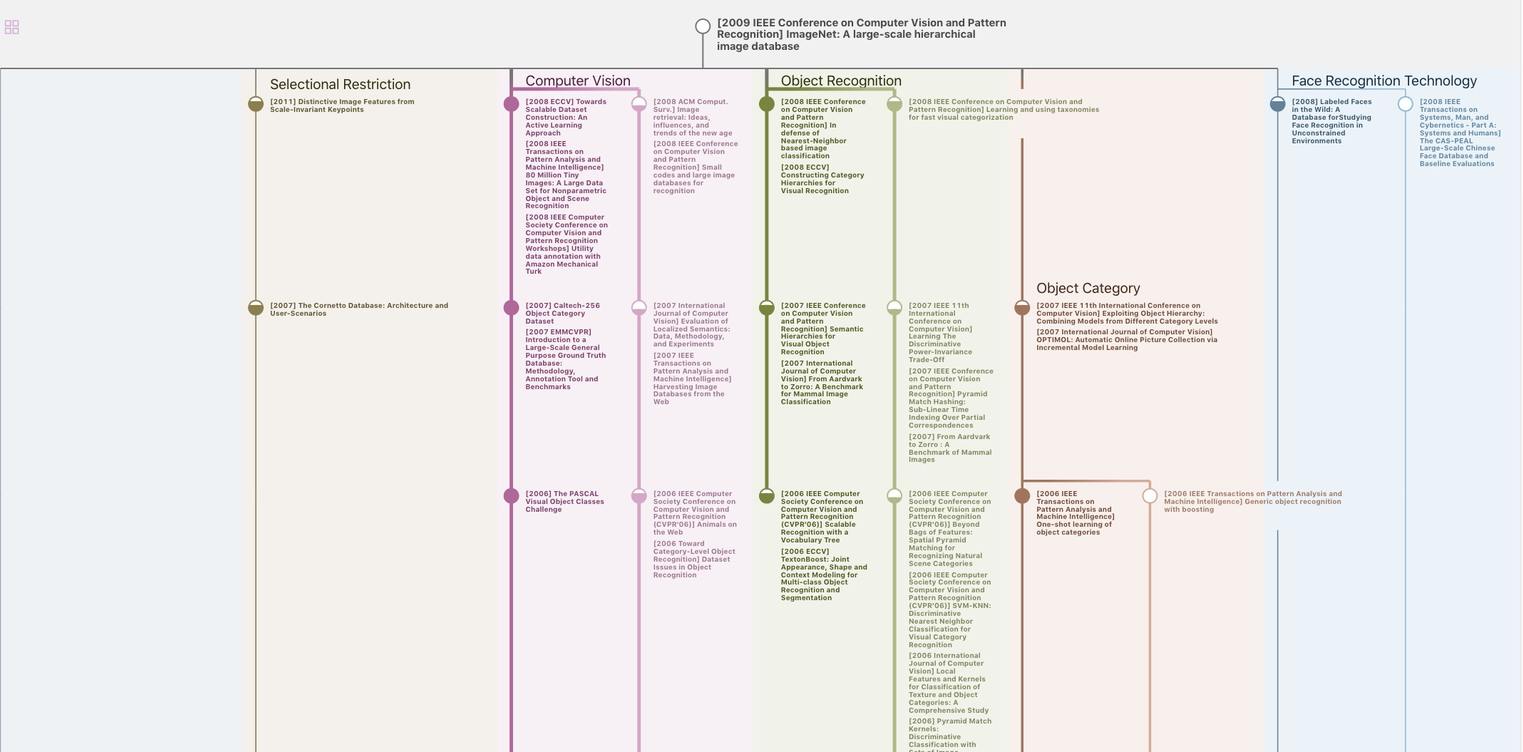
生成溯源树,研究论文发展脉络
Chat Paper
正在生成论文摘要