A Modified Gray Wolf Optimizer-Based Negative Selection Algorithm for Network Anomaly Detection
INTERNATIONAL JOURNAL OF INTELLIGENT SYSTEMS(2023)
摘要
Intrusion detection systems are crucial in fighting against various network attacks. By monitoring the network behavior in real time, possible attack attempts can be detected and acted upon. However, with the development of openness and flexibility of networks, artificial immunity-based network anomaly detection methods lack continuous adaptability and hence have poor detection performance. Thus, a novel framework for network anomaly detection with adaptive regulation is built in this paper. First, a heuristic dimensionality reduction algorithm based on unsupervised clustering is proposed. This algorithm uses the correlation between features to select the best subset. Then, a hybrid partitioning strategy is introduced in the negative selection algorithm (NSA), which divides the feature space into a grid based on the sample distribution density and generates specific candidate detectors in the boundary grid to effectively mitigate the holes caused by boundary diversity. Finally, the NSA is improved by self-set clustering and a novel gray wolf optimizer to achieve adaptive adjustment of the detector radius and position. The results show that the proposed NSA algorithm based on mixed hierarchical division and gray wolf optimization (MDGWO-NSA) achieves a higher detection rate, lower false alarm rate, and better generation quality than other network anomaly detection algorithms.
更多查看译文
关键词
negative selection algorithm,network anomaly detection,anomaly detection,optimizer-based
AI 理解论文
溯源树
样例
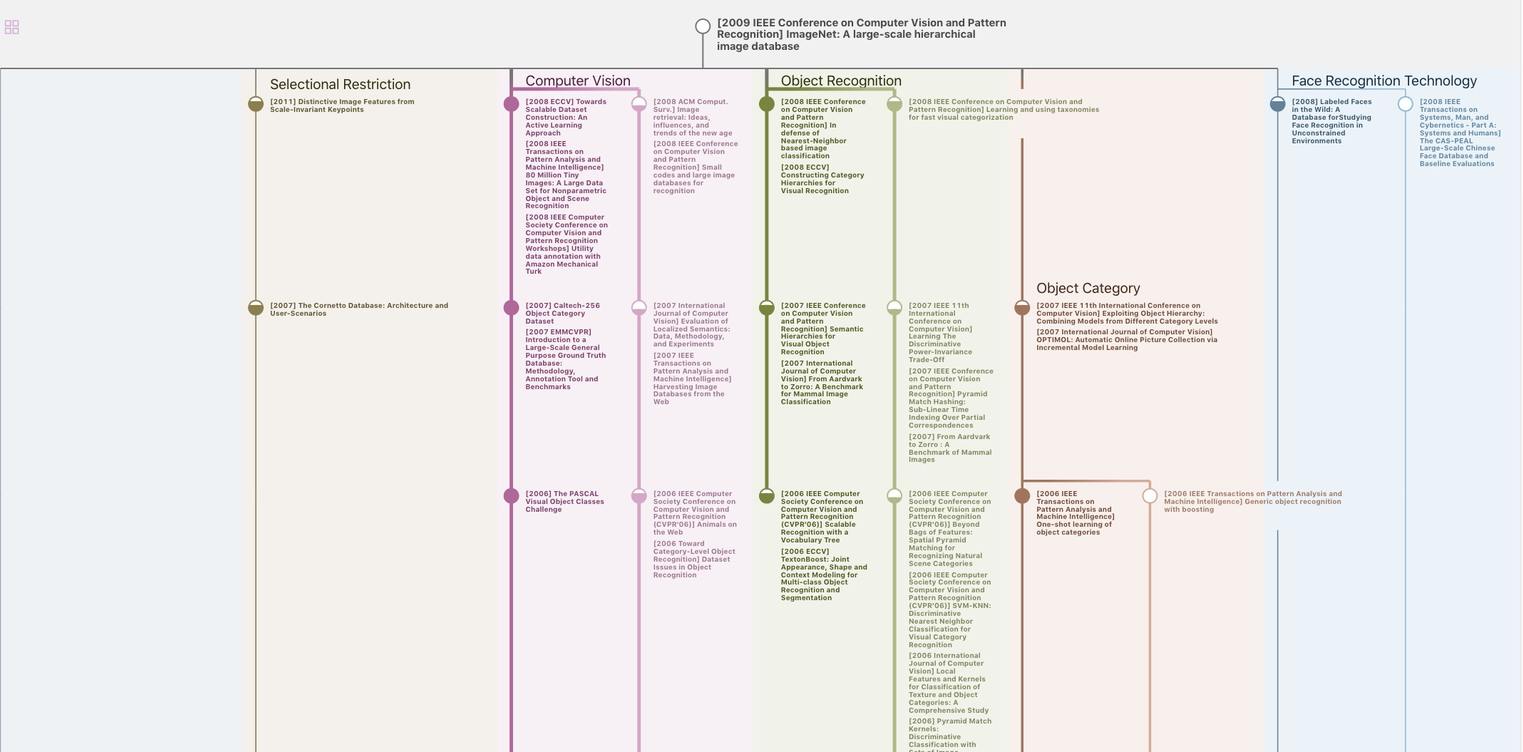
生成溯源树,研究论文发展脉络
Chat Paper
正在生成论文摘要