An Efficient Algorithm for Influence Blocking Maximization based on Community Detection
2019 5th International Conference on Web Research (ICWR)(2019)
摘要
Popularity of online social network services makes it a suitable platform for rapid information diffusion ranging from positive to negatives information. Although the positive diffused information may welcomed by people, the negative information such as rumor, hate and misinformation content should be blocked. However, blocking inappropriate, unwanted and contamination diffusion are not trivial. In particular, in this paper, we study the notion of competing negative and positive campaigns in a social network by addressing the influence blocking maximization (IBM) problem to minimize the bad effect of misinformation. IBM problem can be defined as finding a subset of nodes to promote the positive influence under Multi-campaign Independent Cascade Model as diffusion model to minimize the number of nodes that adopt the negative influence at the end of both propagation processes. In this regard, we proposed a community based algorithm called FC_IBM algorithm using fuzzy clustering and centrality measures for finding a good candidate subset of nodes for diffusion of positive information in order to minimizing the IBM problem. The experimental results on well-known network datasets showed that the proposed algorithm not only outperforms the baseline algorithms with respect to efficiency but also with respect to the final number of positive nodes.
更多查看译文
关键词
social network analysis,influence blocking maximization,community detection,fuzzy clustering,centrality measures
AI 理解论文
溯源树
样例
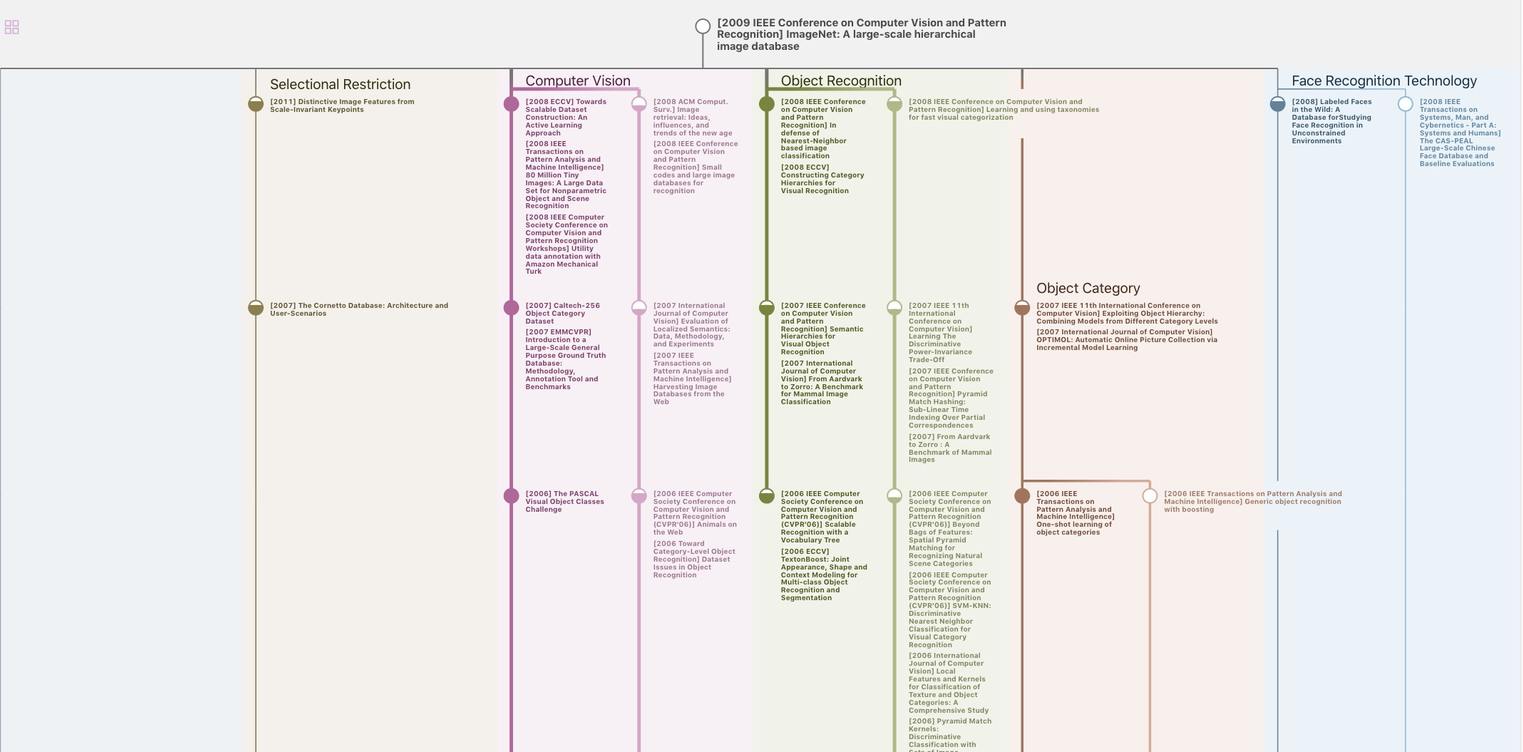
生成溯源树,研究论文发展脉络
Chat Paper
正在生成论文摘要