Mapping seagrass habitats of potential suitability using a hybrid machine learning model
FRONTIERS IN ECOLOGY AND EVOLUTION(2023)
摘要
Seagrass meadows provide essential ecosystem services globally in the context of climate change. However, seagrass is being degraded at an accelerated rate globally due to ocean warming, ocean acidification, aquaculture, and human activities. The need for more information on seagrasses' spatial distribution and health status is a serious impediment to their conservation and management. Therefore, we propose a new hybrid machine learning model (RF-SWOA) that integrates the sinusoidal chaos map whale optimization algorithm (SWOA) with a random forest (RF) model to accurately model the suitable habitat of potential seagrasses. This study combines in situ sampling data with multivariate remote sensing data to train and validate hybrid machine learning models. It shows that RF-SWOA can predict potential seagrass habitat suitability more accurately and efficiently than RF. It also shows that the two most important factors affecting the potential seagrass habitat suitability on Hainan Island in China are distance to land (38.2%) and depth to sea (25.9%). This paper not only demonstrates the effectiveness of a hybrid machine learning model but also provides a more accurate machine learning model approach for predicting the potential suitability distribution of seagrasses. This research can help identify seagrass suitability distribution areas and thus develop conservation strategies to restore healthy seagrass ecosystems.
更多查看译文
关键词
seagrass,machine learning,species distribution model,hybrid model,habitat suitability,niches
AI 理解论文
溯源树
样例
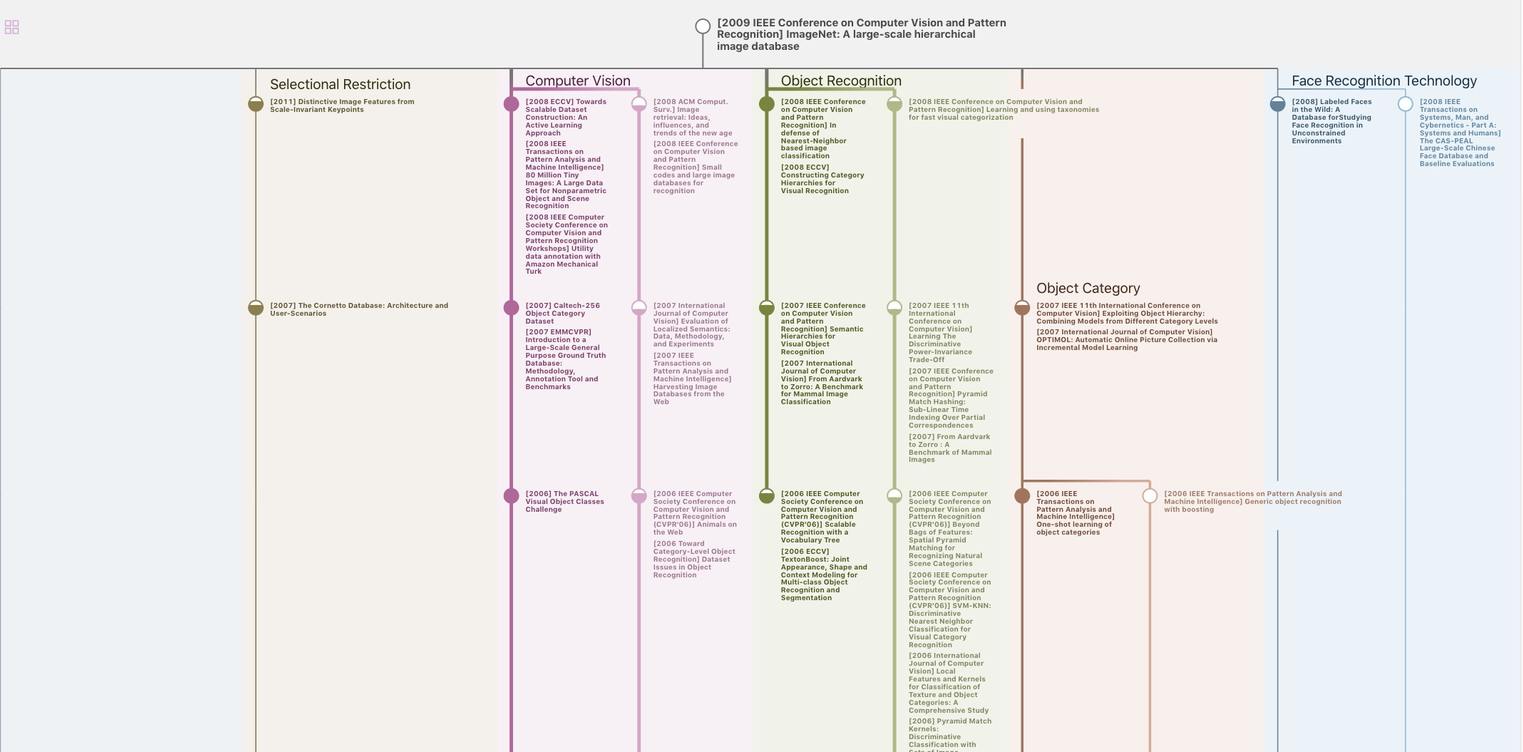
生成溯源树,研究论文发展脉络
Chat Paper
正在生成论文摘要