Multiplierless low-cost implementation of Hindmarsh-Rose neuron model in case of large-scale realization
INTERNATIONAL JOURNAL OF CIRCUIT THEORY AND APPLICATIONS(2023)
摘要
Implementation of neural networks in case of hardware helps us to understand the different parts of the human brain operation, using artificial intelligence (AI). This paper presents a new model of the Hindmarsh-Rose (HR) Neuron that is based on basic polynomial functions called Nyquist-look up table-Hindmarsh-Rose (N-LUT-HR) based on an accurate sampling of the original model. The proposed approach is investigated in terms of its digital realization feasibility. According to high matching between the original and proposed terms, it is showed that the new modified model can follow all spiking patterns of primary model with low-error computations. In hardware case, the proposed and original models are implemented on Xilinx FPGA XC2VP30 chip to validate different aspects of the simulation results. Hardware results demonstrate that our model regenerates the desired patterns in low-cost and high-frequency (speed-up) in comparison with the other similar works. Overall saving in FPGA resources show that this new model is capable of being used in large-scale networks in case of minimum required resources (FPGA costs). In addition, the analysis of hardware indicates that the new circuits can work in a maximum frequency of 123 MHz with 98.25% saving in FPGA costs (resources utilization of FPGA).
更多查看译文
关键词
CNS FPGA,HR,neuron
AI 理解论文
溯源树
样例
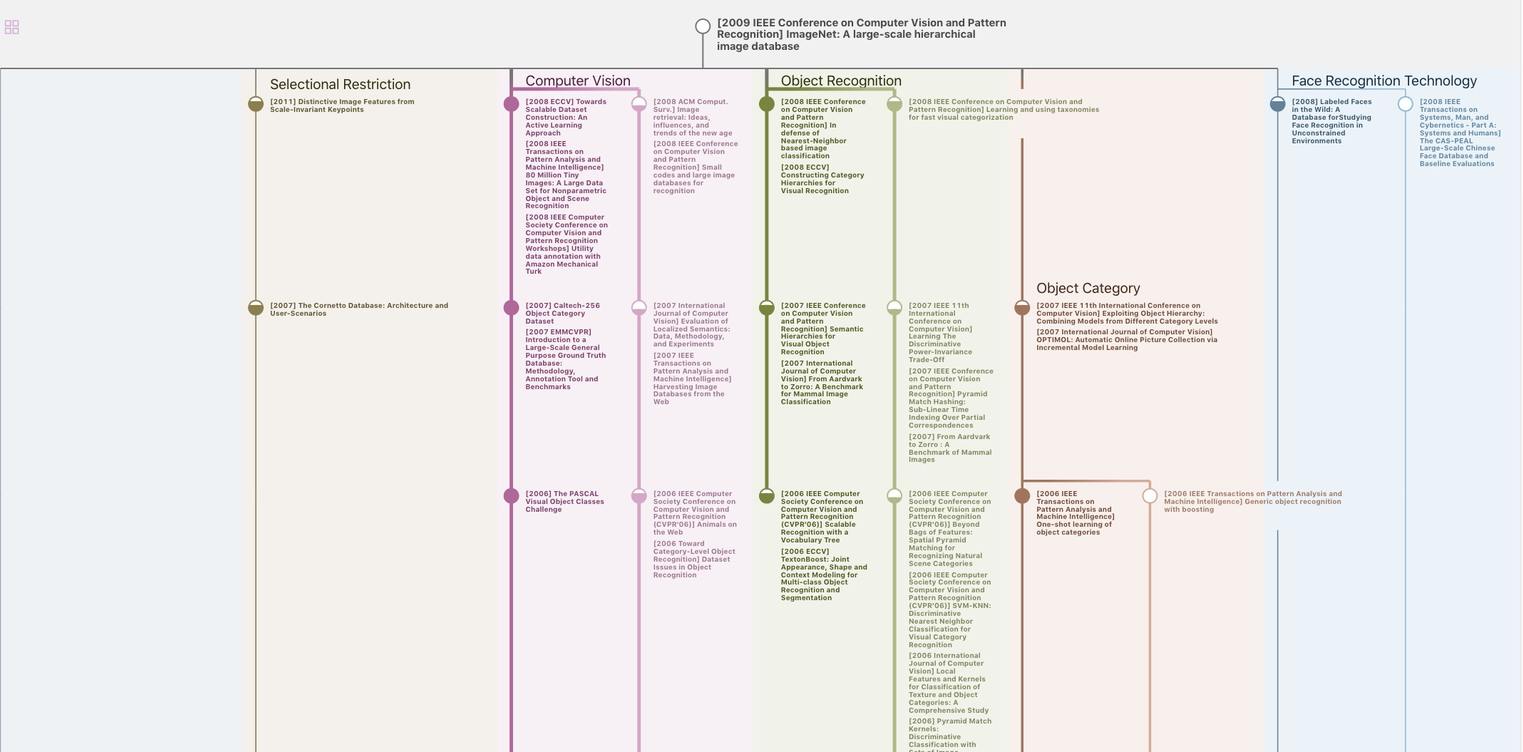
生成溯源树,研究论文发展脉络
Chat Paper
正在生成论文摘要