Flexible few-shot class-incremental learning with prototype container
NEURAL COMPUTING & APPLICATIONS(2023)
Abstract
In the few-shot class-incremental learning, new class samples are utilized to learn the characteristics of new classes, while old class exemplars are used to avoid old knowledge forgetting. The limited number of new class samples is more likely to cause overfitting during incremental training. Moreover, mass stored old exemplars mean large storage space consumption. To solve the above difficulties, in this paper we propose a novel flexible few-shot class-incremental framework to make the incremental process efficient and convenient. We enhance the expression ability of extracted features through multistage pre-training. Then, we set up a prototype container to store each class prototype to retain old knowledge. When new classes flow in, we calculate the new class prototypes and update the prototype container. Finally, we get the prediction result through similarity weighting. The entire framework only need to train the base class classifier and does not require further training during the incremental process. It avoids the overfitting of novel classes and saves time for further training. Besides, storing prototypes can save more storage space than original image data. Overall, the entire framework has the advantage of flexibility. We conduct extensive experiments on three standard few-shot class-incremental datasets and achieve state-of-the-art results. Especially, to verify the flexibility of the framework, we discuss the special federated few-shot class-incremental scenarios in addition. No further training and less storage consumption provide the possibility for applications in more complex scenarios.
MoreTranslated text
Key words
Few-shot class-incremental learning,Few-shot learning,Incremental learning
AI Read Science
Must-Reading Tree
Example
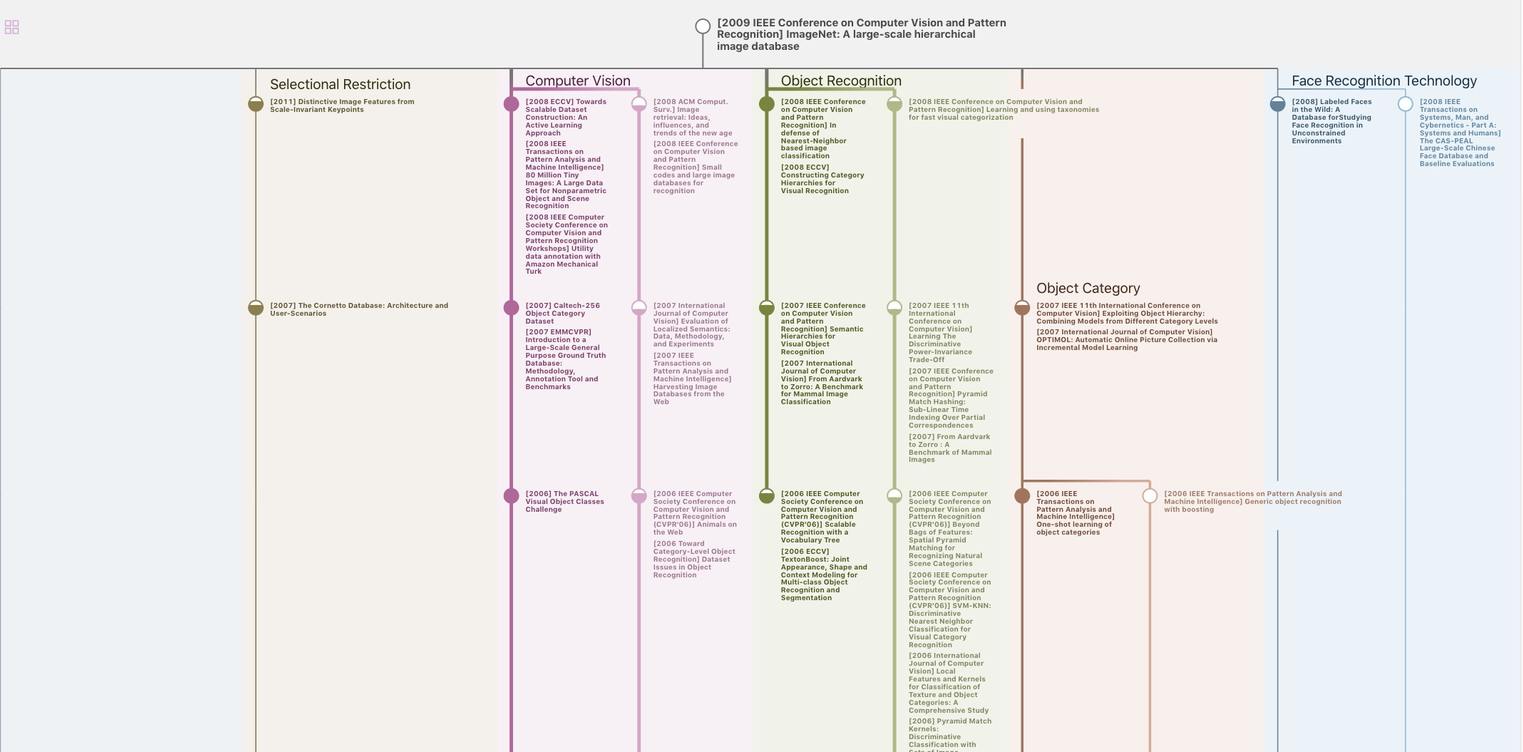
Generate MRT to find the research sequence of this paper
Chat Paper
Summary is being generated by the instructions you defined