Brain Cognition-Inspired Dual-Pathway CNN Architecture for Image Classification
IEEE TRANSACTIONS ON NEURAL NETWORKS AND LEARNING SYSTEMS(2023)
摘要
Inspired by the global-local information processing mechanism in the human visual system, we propose a novel convolutional neural network (CNN) architecture named cognition-inspired network (CogNet) that consists of a global pathway, a local pathway, and a top-down modulator. We first use a common CNN block to form the local pathway that aims to extract fine local features of the input image. Then, we use a transformer encoder to form the global pathway to capture global structural and contextual information among local parts in the input image. Finally, we construct the learnable top-down modulator where fine local features of the local pathway are modulated by global representations of the global pathway. For ease of use, we encapsulate the dual-pathway computation and modulation process into a building block, called the global-local block (GL block), and a CogNet of any depth can be constructed by stacking a necessary number of GL blocks one after another. Extensive experimental evaluations have revealed that the proposed CogNets have achieved the state-of-the-art performance accuracies on all the six benchmark datasets and are very effective for overcoming the "texture bias " and the "semantic confusion " problems faced by many CNN models.
更多查看译文
关键词
Visualization,Feature extraction,Convolutional neural networks,Modulation,Semantics,Transformers,Shape,Cognition inspired,cognition-inspired network (CogNet),convolutional neural network (CNN),dual pathway,semantic confusion (SC),texture bias (TB),transformer
AI 理解论文
溯源树
样例
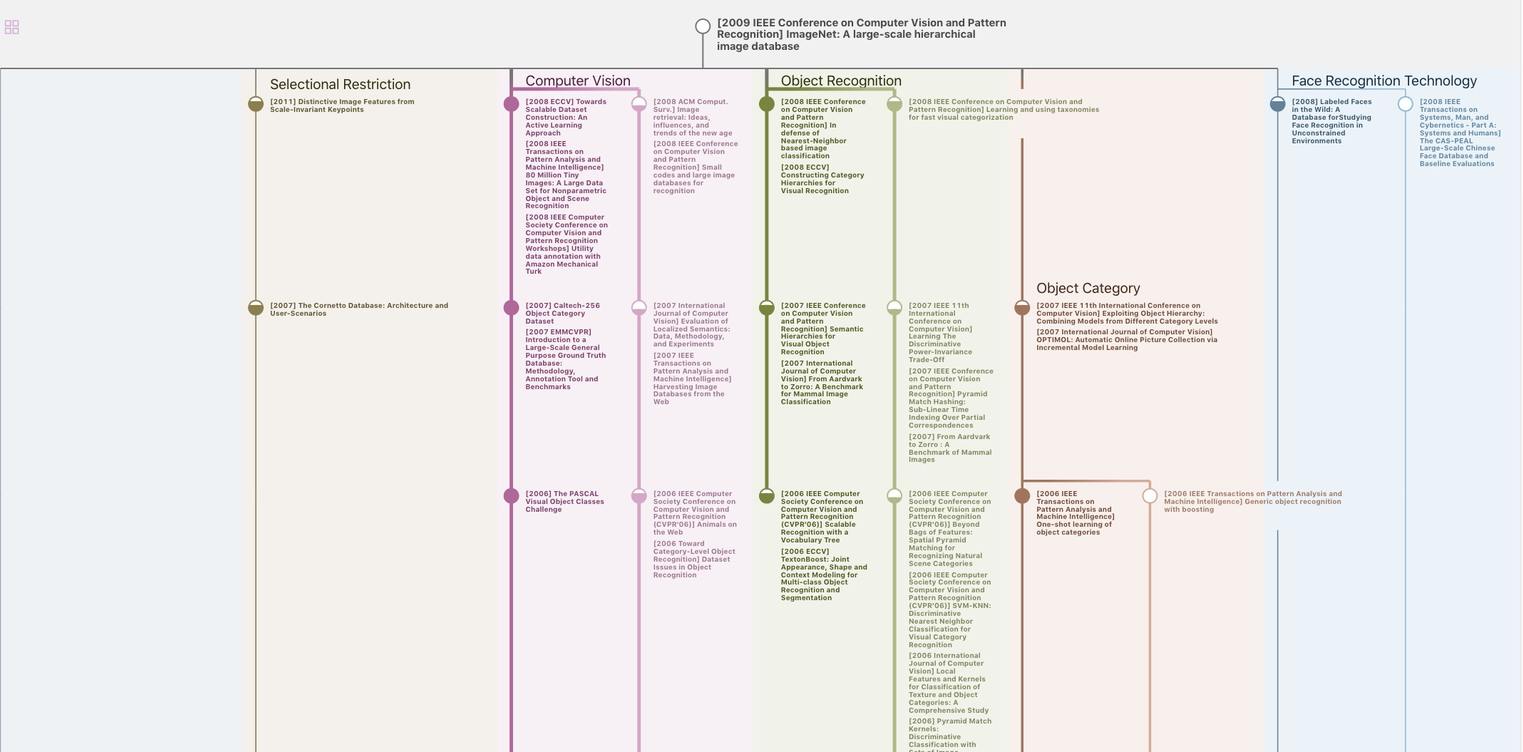
生成溯源树,研究论文发展脉络
Chat Paper
正在生成论文摘要