Deep Learning-Based PM2.5 Long Time-Series Prediction by Fusing Multisource Data-A Case Study of Beijing
ATMOSPHERE(2023)
Abstract
Accurate air quality prediction is of great significance for pollution prevention and disaster prevention. Effective and reliable prediction models are needed not only for short time prediction, but are even more important for long time-series future predictions. In the long time series, most of the current models might not function as accurately as in the short period and thus a new model is required. In this paper, the new PM2.5 predictor is proposed to achieve accurate long time series PM2.5 prediction in Beijing. The predictor simplifies the input parameters through Spearman correlation analysis and implements the long time series prediction through Informer. The results show that AQI, CO, NO2, and PM10 concentrations are selected from the air quality data, and Dew Point Temperature (DEWP) and wind speed are incorporated from two meteorological data to better improve the prediction efficiency by almost 27%. By comparing with LSTM and attention-LSTM models, the model constructed in this paper performs well in different prediction time periods, with at least 21%, 19%, 28%, and 35% improvement in accuracy in four prediction time series: 48 h, 7 days, 14 days, and 30 days. In conclusion, the proposed model is proved to solve the problem of predicting long time series PM2.5 concentrations in the future, which can make up for the shortcomings of the currently existing models and have good application value.
MoreTranslated text
Key words
PM2 5,prediction,deep learning,correlation
AI Read Science
Must-Reading Tree
Example
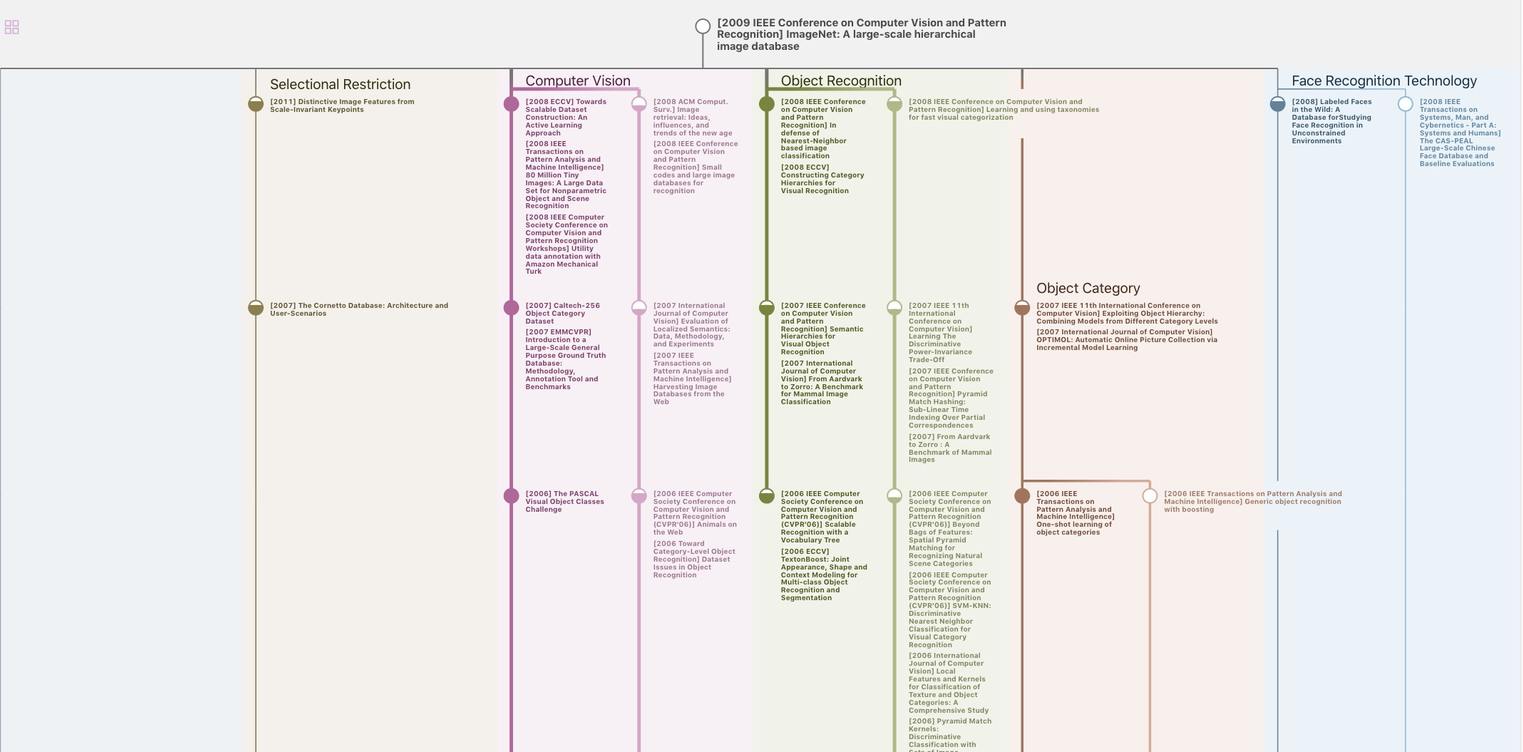
Generate MRT to find the research sequence of this paper
Chat Paper
Summary is being generated by the instructions you defined