Modified Conditional Restricted Boltzmann Machines for Query Recommendation in Digital Archives
APPLIED SCIENCES-BASEL(2023)
摘要
Digital archives (DAs) usually store diverse expert-level materials. Nowadays, access to DAs is increasing for non-expert users, However, they might have difficulties formulating appropriate search queries to find the necessary information. In response to this problem, we propose a query log-based query recommendation algorithm that provides expert knowledge to non-expert users, thus supporting their information seeking in DAs. The use case considered is one where after users enter some general queries, they will be recommended semantically similar expert-level queries in the query logs. The proposed modified conditional restricted Boltzmann machines (M-CRBMs) are capable of utilizing the rich metadata in DAs, thereby alleviating the sparsity problem that conventional restricted Boltzmann machines (RBMs) will face. Additionally, compared with other CRBM models, we drop a large number of model weights. In the experiments, the M-CRBMs outperform the conventional RBMs when using appropriate metadata, and we find that the recommendation results are relevant to the metadata fields that are used in M-CRBMs. Through experiments on the Europeana dataset, we also demonstrate the versatility and scalability of our proposed model.
更多查看译文
关键词
query recommendation,digital archives,conditional restricted Boltzmann machines,machine learning
AI 理解论文
溯源树
样例
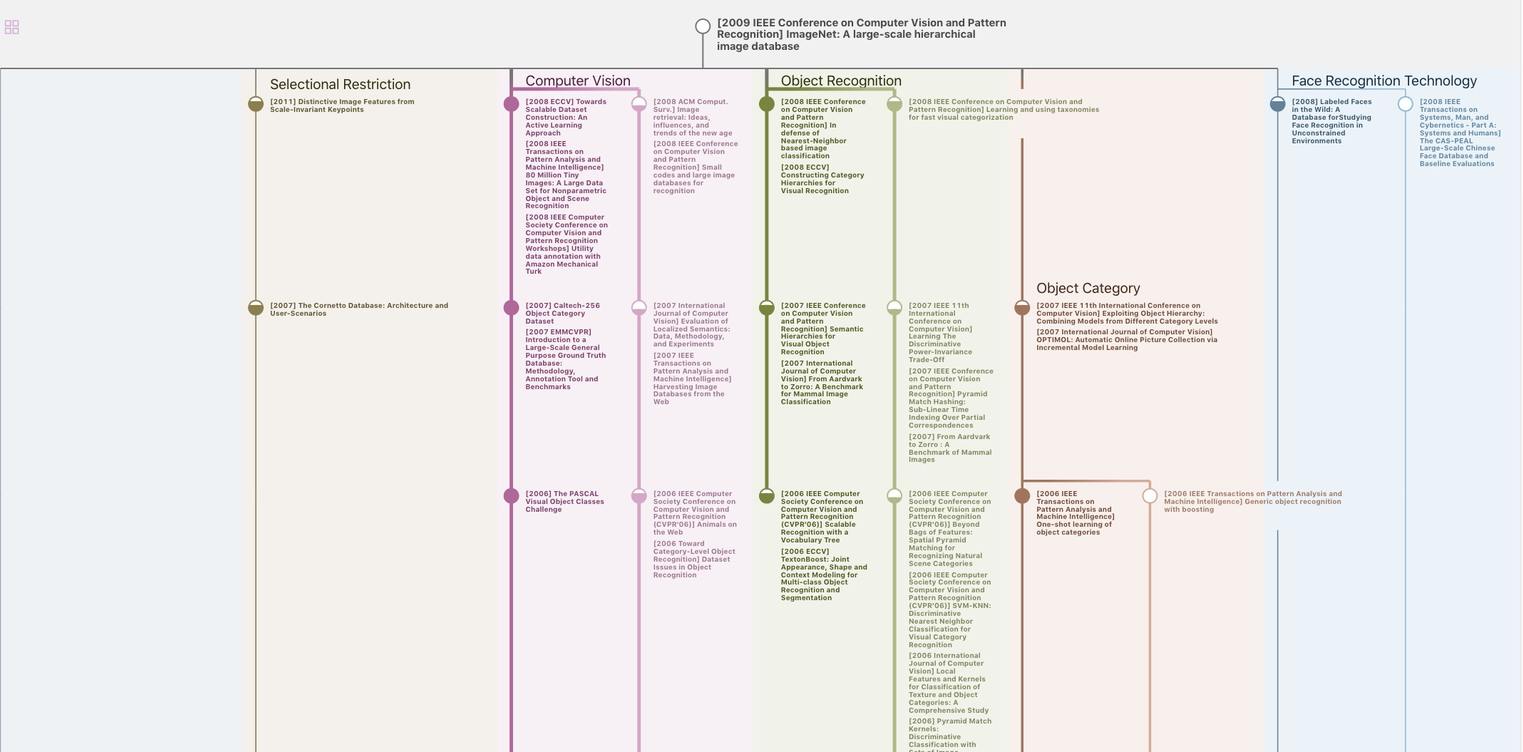
生成溯源树,研究论文发展脉络
Chat Paper
正在生成论文摘要