A gated multi-hierarchical feature fusion network for recognizing steel plate surface defects
MULTIMEDIA SYSTEMS(2023)
Abstract
Recognizing defects on the steel plate surface has great application potential in the steel manufacturing process. However, it is still challenging to accurately recognize surface defects since most defects only occupy a small area of the whole image and have high similarities to the surrounding backgrounds. To solve the above issues, we propose an attention multi-hierarchical feature fusion network (AMHNet) to recognize defects. First, to better fuse the features from different levels, we propose a skipping attention module to selectively transfer informative features in low-level layers into high-level layers based on the convolutional block attention mechanism. Second, to dynamically fuse multi-hierarchical features, we propose a feature dynamic aggregation gate by gating mechanism to enhance defect-relevant features and suppress useless features. Finally, to verify the effectiveness and advantages of our model, we also collect a new challenging defect recognition dataset called NPU-DRD. Extensive experiments on dataset NPU-DRD show that our AMHNet achieves an accuracy of 97.58% and an AUC score of 97.23%, which are the new state-of-the-art results among existing methods. Our new dataset and source codes are available at https://github.com/Heisenberg828/AMHNet .
MoreTranslated text
Key words
Steel defect recognition,Feature aggregation,Attentional mechanism,Multi-hierarchical feature
AI Read Science
Must-Reading Tree
Example
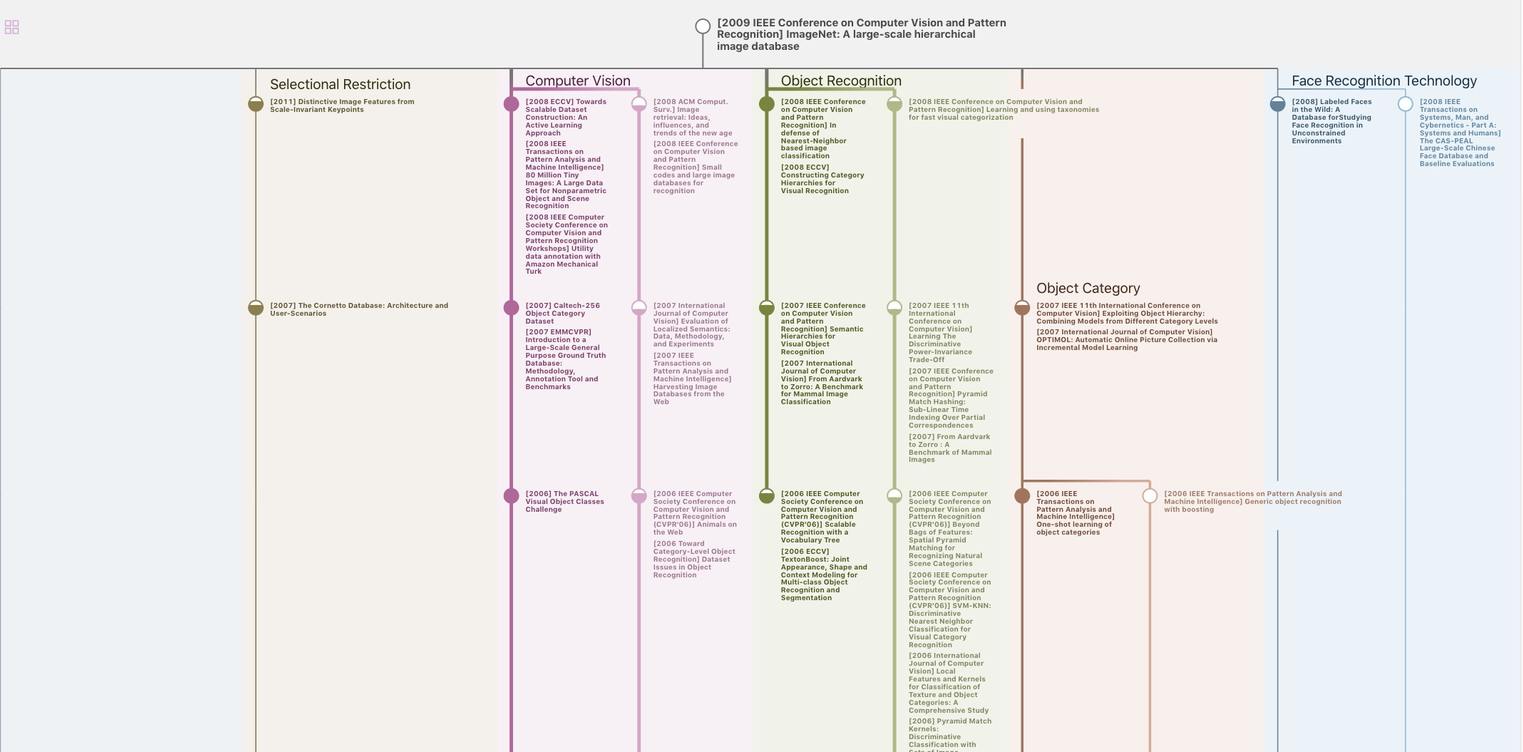
Generate MRT to find the research sequence of this paper
Chat Paper
Summary is being generated by the instructions you defined