Machine Learning Application for Output Capacitance Accuracy Improvement: A Case Study for Combinational Cells
ACS APPLIED ELECTRONIC MATERIALS(2023)
摘要
The lack of physical information at the early gatelevel Netlist makes timing accuracy a significant challenge. Advancing in the physical design flow reduces the timing inaccuracy gap in the cell's delay estimation, where the physical layout information is no longer estimated. This paper aims to improve the cell delay accuracy of Oasys-RTL Synthesis by predicting the cell's output capacitance using Machine Learning algorithms. We trained and tested various ML Regression algorithms to predict the Oasys-RTL cell's output capacitance using several 16 nm designs. With the help of a state-of-art tool, APRISA P&R by Siemens EDA, we derived accurate output capacitance values and compared the inaccuracy and error margins between postplaced APRISA and postplaced Oasys-RTL Netlists before and after applying ML models. We reached the highest accuracy using the Random Forest model. The RF model has achieved a total average accuracy of 93.04% and has reduced the RMSE from 2.537 to 0.655.
更多查看译文
关键词
delay estimation,output capacitance,timing accuracy,machine learning,regression
AI 理解论文
溯源树
样例
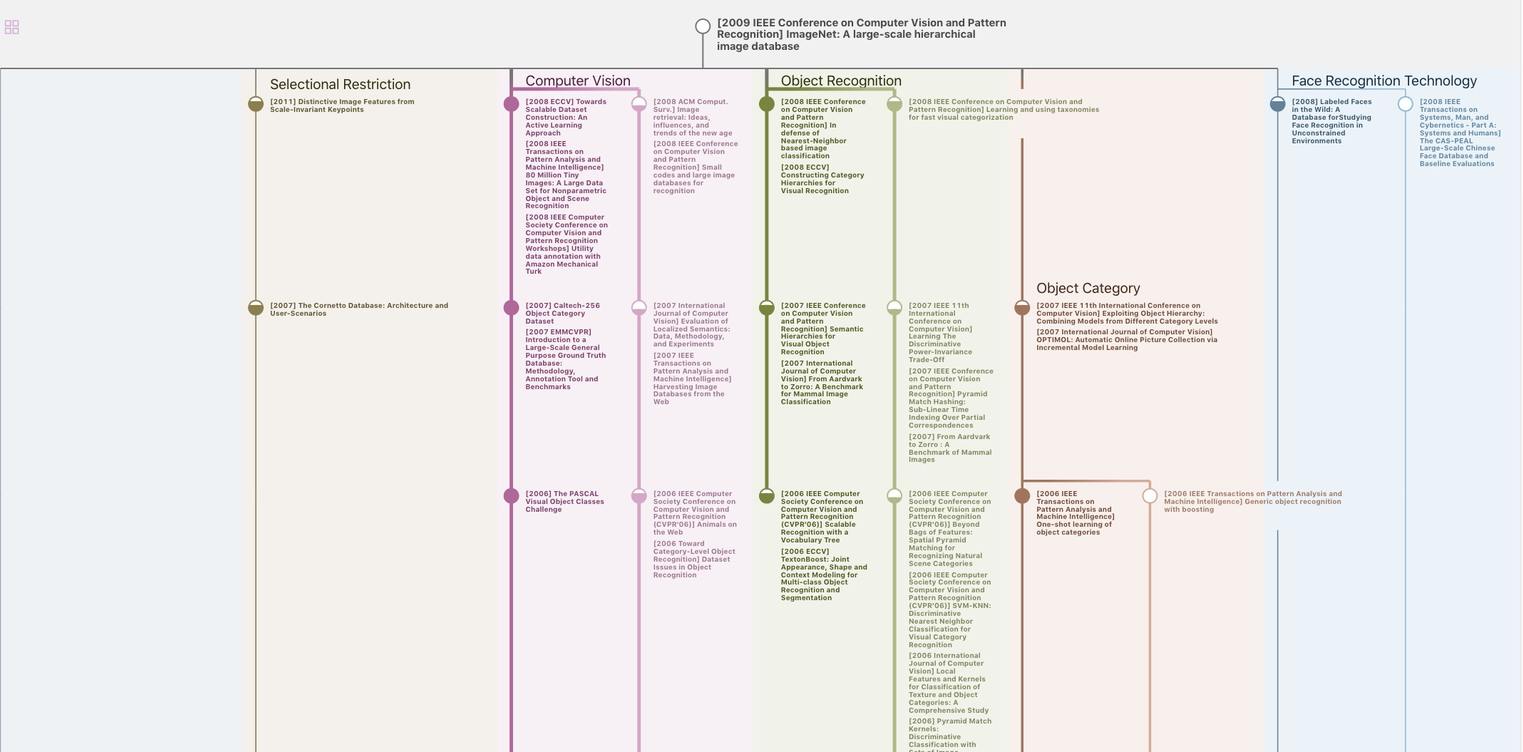
生成溯源树,研究论文发展脉络
Chat Paper
正在生成论文摘要