Geographically weighted regression (GWR) and Prediction-area (P-A) plot to generate enhanced geochemical signatures for mineral exploration targeting
APPLIED GEOCHEMISTRY(2023)
摘要
The geochemical exploration provides an effective and direct spatial evidence for identifying undiscovered de-posits. Geochemical data are spatial data with many attributes, such as closure effect, collinearity, multivariate, spatial correlation and heterogeneity. The data processing method considering the attributes of geochemical data is very important for accurately identification of the undiscovered deposits. In this paper, a data-driven mineral exploration targeting method was proposed, first log-ratio transformation was performed to eliminate the closure effect, and then central standardization was applied to avoid collinearity. Then, the geographically weighted regression (GWR) was carried out with rock-forming oxides and immobile elements as dependent variables and ore-forming elements as independent variables, which considering the spatial attributes of geochemical data, and the residuals were regarded as the geochemical evidences. Afterwards, associated elements for mineralization were selected and the P-A plot was used to evaluate the relative importance of ore-forming elements (residual errors) and associated elements to indicate the locations of the deposits of the type sought, and finally an enhanced data-driven multi-element geochemical signature was generated for mineral exploration targeting. The geochemical anomalies extraction effect was significantly enhanced compared with traditional method. The method considering the multi-attribute characteristics of geochemical data provided an effective geochemical proxy for mineral exploration targeting, which was of great significance for improving the geochemical explo-ration theory and prospecting practice.
更多查看译文
关键词
Geochemical exploration,GWR,P-A plot,Ore-induced anomalies,Mineral exploration targeting
AI 理解论文
溯源树
样例
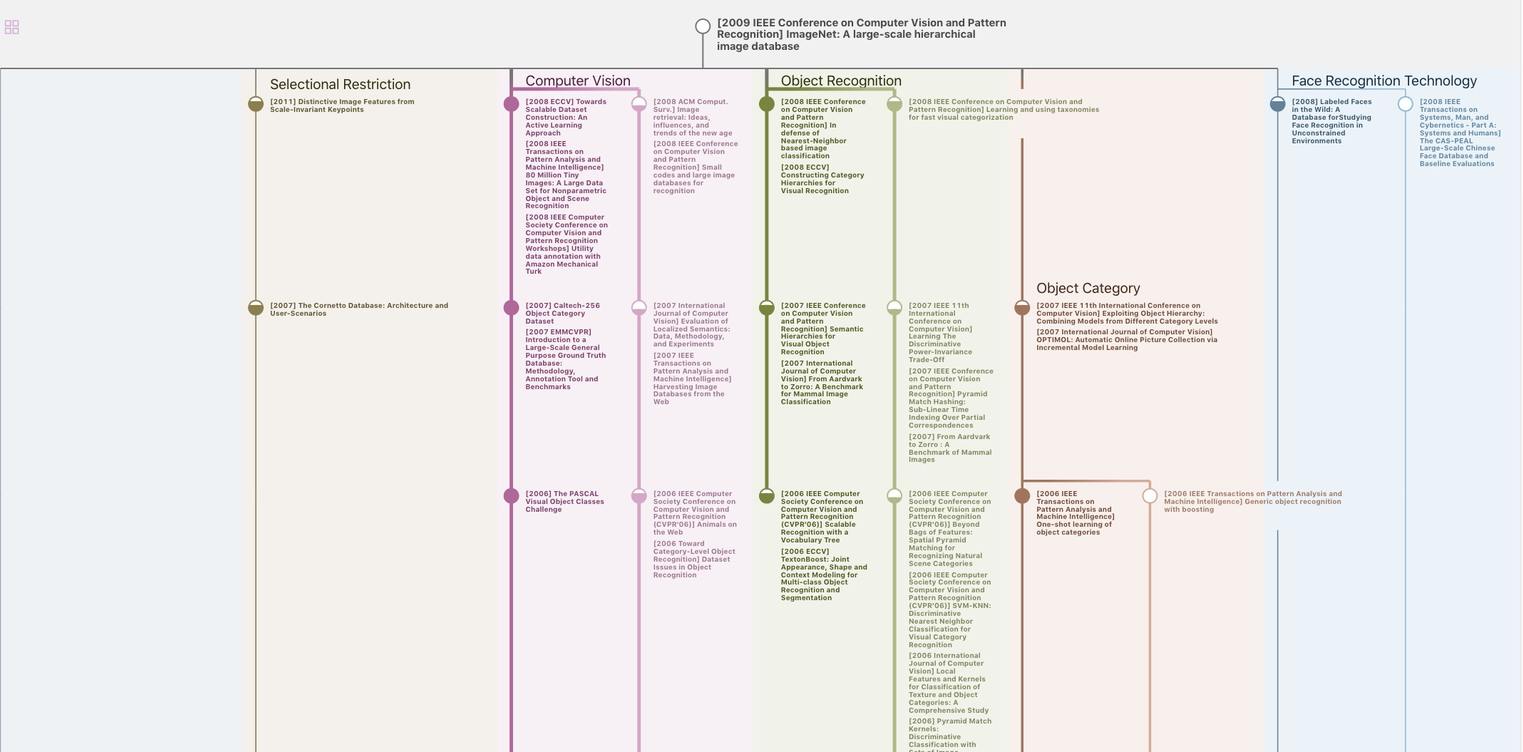
生成溯源树,研究论文发展脉络
Chat Paper
正在生成论文摘要