Variable Selection Using Deep Variational Information Bottleneck with Drop-Out-One Loss
APPLIED SCIENCES-BASEL(2023)
摘要
The information bottleneck (IB) model aims to find the optimal representations of input variables with respect to the response variable. While it has been widely used in the machine-learning community, research from the perspective of the information-theoretic method has been rarely reported regarding variable selection. In this paper, we investigate DNNs for variable selection through an information-theoretic lens. To be specific, we first state the rationality of variable selection with IB and then propose a new statistic to measure the variable importance. On this basis, a new algorithm based on a deep variational information bottleneck is developed to calculate the statistic, in which we consider the Gaussian distribution and the exponential distribution to estimate the Kullback-Leibler divergence. Empirical evaluations on simulated and real-world data show that the proposed method performs better than classical variable-selection methods. This confirms the feasibility of the variable selection from the perspective of IB.
更多查看译文
关键词
information bottleneck,drop-out-one loss,variable selection,deep learning
AI 理解论文
溯源树
样例
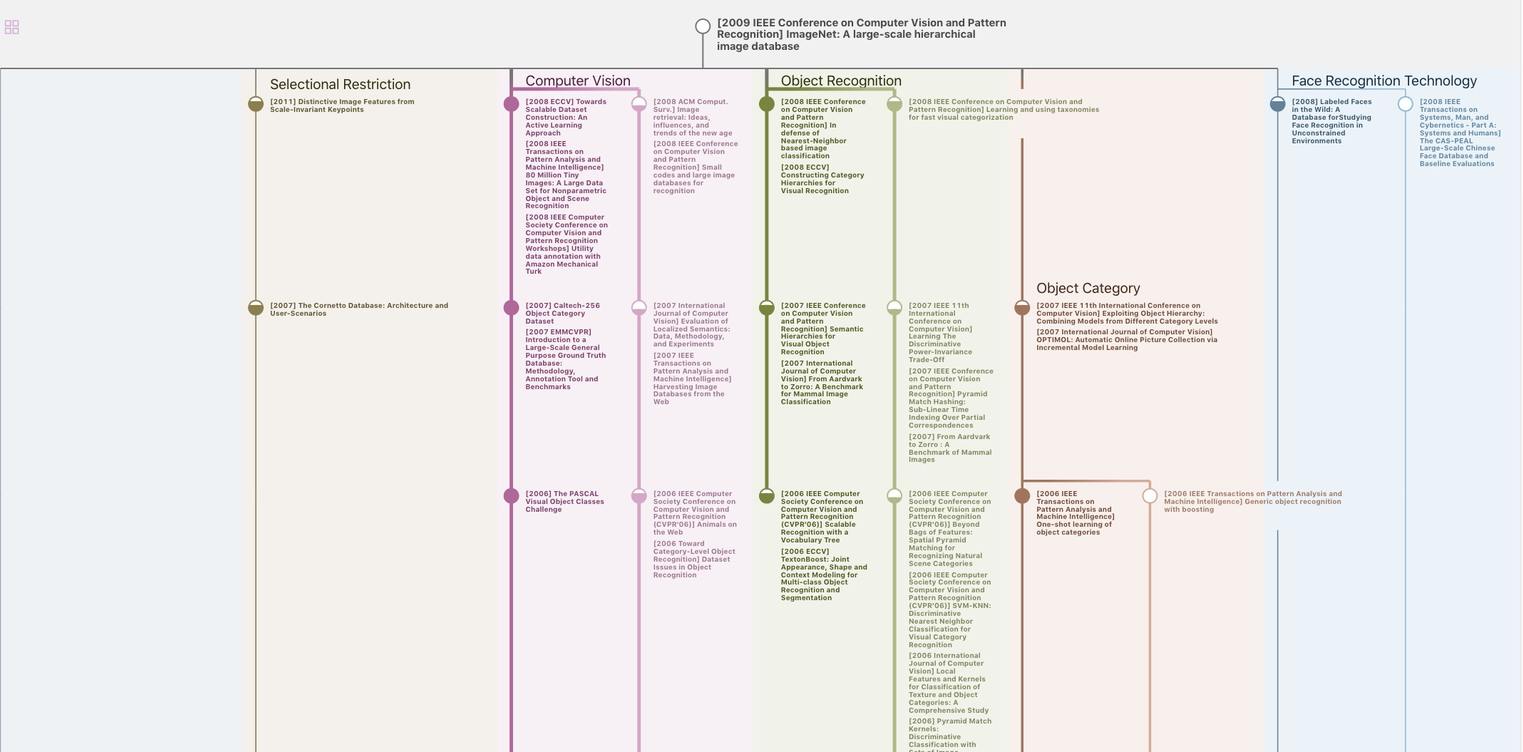
生成溯源树,研究论文发展脉络
Chat Paper
正在生成论文摘要