An Overview of Advanced Deep Graph Node Clustering
IEEE TRANSACTIONS ON COMPUTATIONAL SOCIAL SYSTEMS(2024)
摘要
Graph data have become increasingly important, and graph node clustering has emerged as a fundamental task in data analysis. In recent years, graph node clustering has gradually moved from traditional shallow methods to deep neural networks due to the powerful representation capabilities of deep learning. In this article, we review some representatives of the latest graph node clustering methods, which are classified into three categories depending on their principles. Extensive experiments are conducted on real-world graph datasets to evaluate the performance of these methods. Four mainstream evaluation performance metrics are used, including clustering accuracy, normalized mutual information, adjusted rand index, and F1-score. Based on the experimental results, several potential research challenges and directions in the field of deep graph node clustering are pointed out. This work is expected to facilitate researchers interested in this field to provide some insights and further promote the development of deep graph node clustering.
更多查看译文
关键词
Clustering methods,Clustering algorithms,Task analysis,Deep learning,Neural networks,Feature extraction,Computational modeling,Deep clustering,deep learning,graph node clustering,neural networks,unsupervised learning
AI 理解论文
溯源树
样例
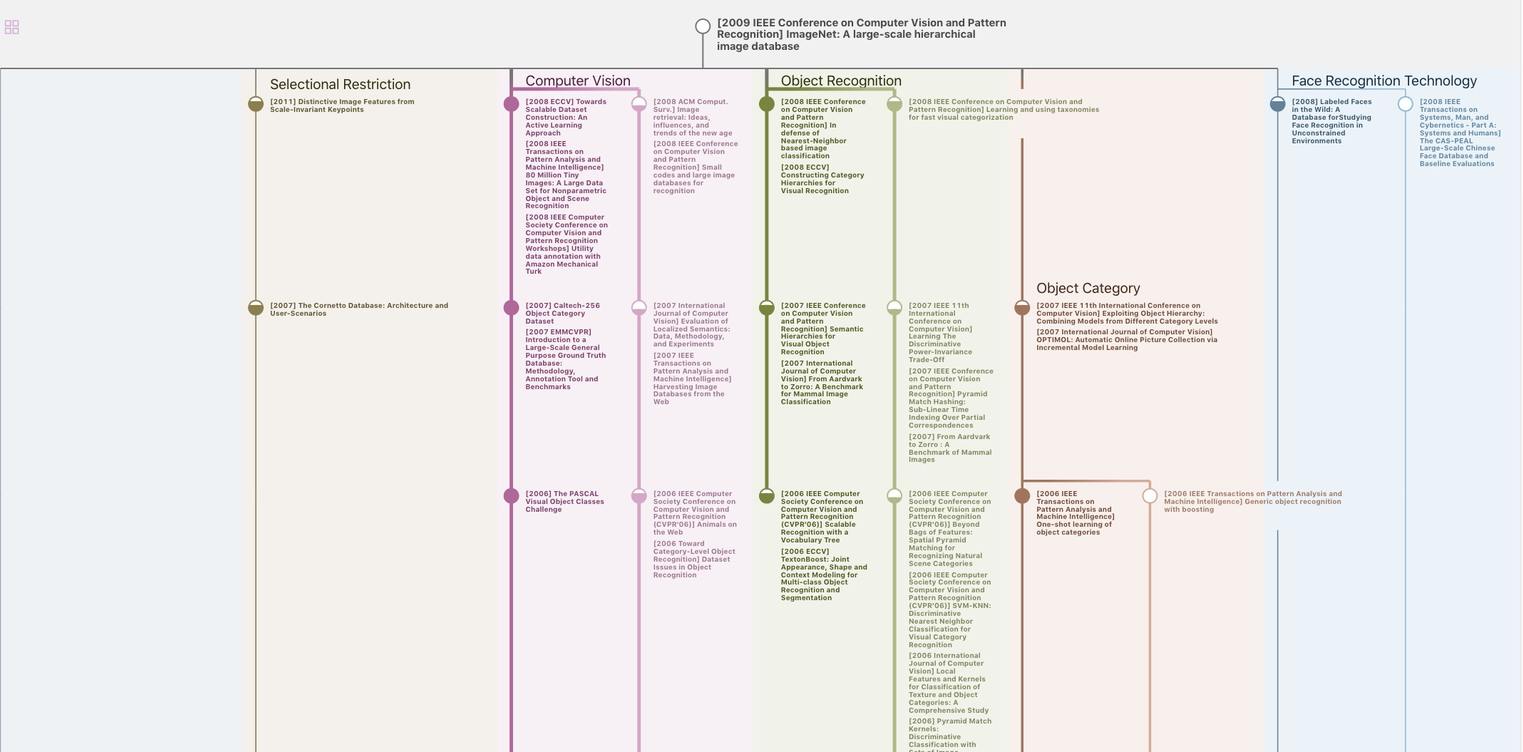
生成溯源树,研究论文发展脉络
Chat Paper
正在生成论文摘要