DDNSR: a dual-input degradation network for real-world super-resolution
PATTERN ANALYSIS AND APPLICATIONS(2023)
摘要
Recently, Real-World Super-Resolution has become one of the most popular research fields in the scope of Single Image Super-Resolution, as it focuses on real-world applications. Due to the lack of paired training data, developing real-world super-resolution is considered a more challenging problem. Previous works intended to model the real image degradation process so that paired training images could be obtained. Specifically, some methods attempt to explicitly estimate degradation kernels and noise patterns, while others introduce degradation networks to learn maps from high-resolutions (HRs) to low-resolutions (LRs), which is a more direct and practical way. However, previous degradation networks take only one HR image as an input and therefore can hardly learn the real sensor noise contained in LR samples. In this paper, we propose a novel dual-input degradation network that takes a real LR image as an additional input to better learn the real sensor noise. Furthermore, we propose an effective self-supervised learning method to synchronously train the degradation network along with the reconstruction network. Extensive experiments showed that our dual-input degradation network can better simulate the real degradation process, thereby indicating that the reconstruction network outperforms state-of-the-art methods. Original codes and most of the testing data can be found on our website.
更多查看译文
关键词
Real-world super-resolution,Degradation network,Self-supervised learning,Deep learning
AI 理解论文
溯源树
样例
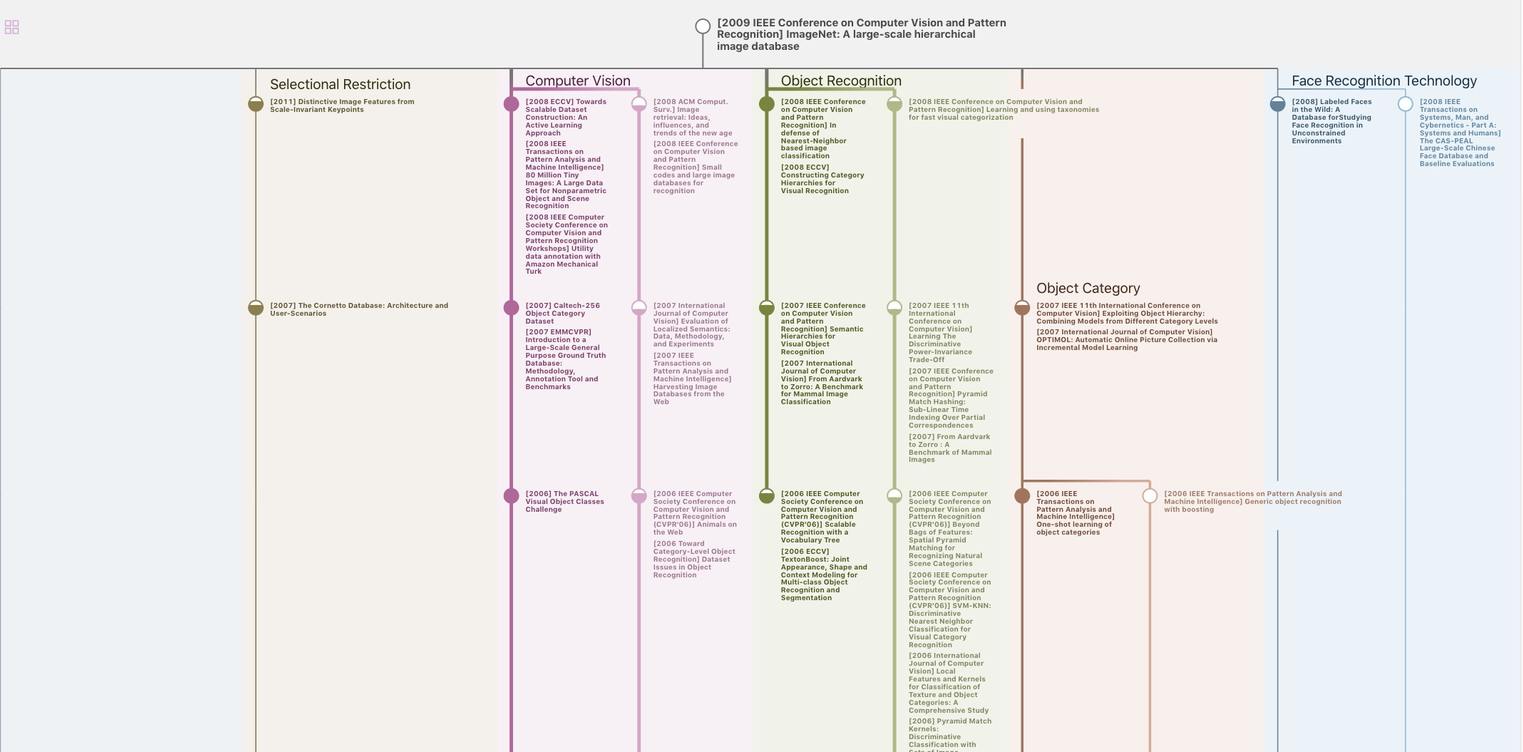
生成溯源树,研究论文发展脉络
Chat Paper
正在生成论文摘要