On exploring weakly supervised domain adaptation strategies for semantic segmentation using synthetic data
MULTIMEDIA TOOLS AND APPLICATIONS(2023)
摘要
Pixel-wise image segmentation is key for many Computer Vision applications. The training of deep neural networks for this task has expensive pixel-level annotation requirements, thus, motivating a growing interest on synthetic data to provide unlimited data and its annotations. In this paper, we focus on the generation and application of synthetic data as representative training corpuses for semantic segmentation of urban scenes. First, we propose a synthetic data generation protocol, which identifies key features affecting performance and provides datasets with variable complexity. Second, we adapt two popular weakly supervised domain adaptation approaches (combined training, fine-tuning) to employ synthetic and real data. Moreover, we analyze several backbone models, real/synthetic datasets and their proportions when combined. Third, we propose a new curriculum learning strategy to employ several synthetic and real datasets. Our major findings suggest the high performance impact of pace and order of synthetic and real data presentation, achieving state of the art results for well-known models. The results by training with the proposed dataset outperform popular alternatives, thus demonstrating the effectiveness of the proposed protocol. Our code and dataset are available at http://www-vpu.eps.uam.es/publications/WSDA_semantic/
更多查看译文
关键词
Synthetic data,Semantic segmentation,Domain adaptation,Weakly supervised domain adaptation
AI 理解论文
溯源树
样例
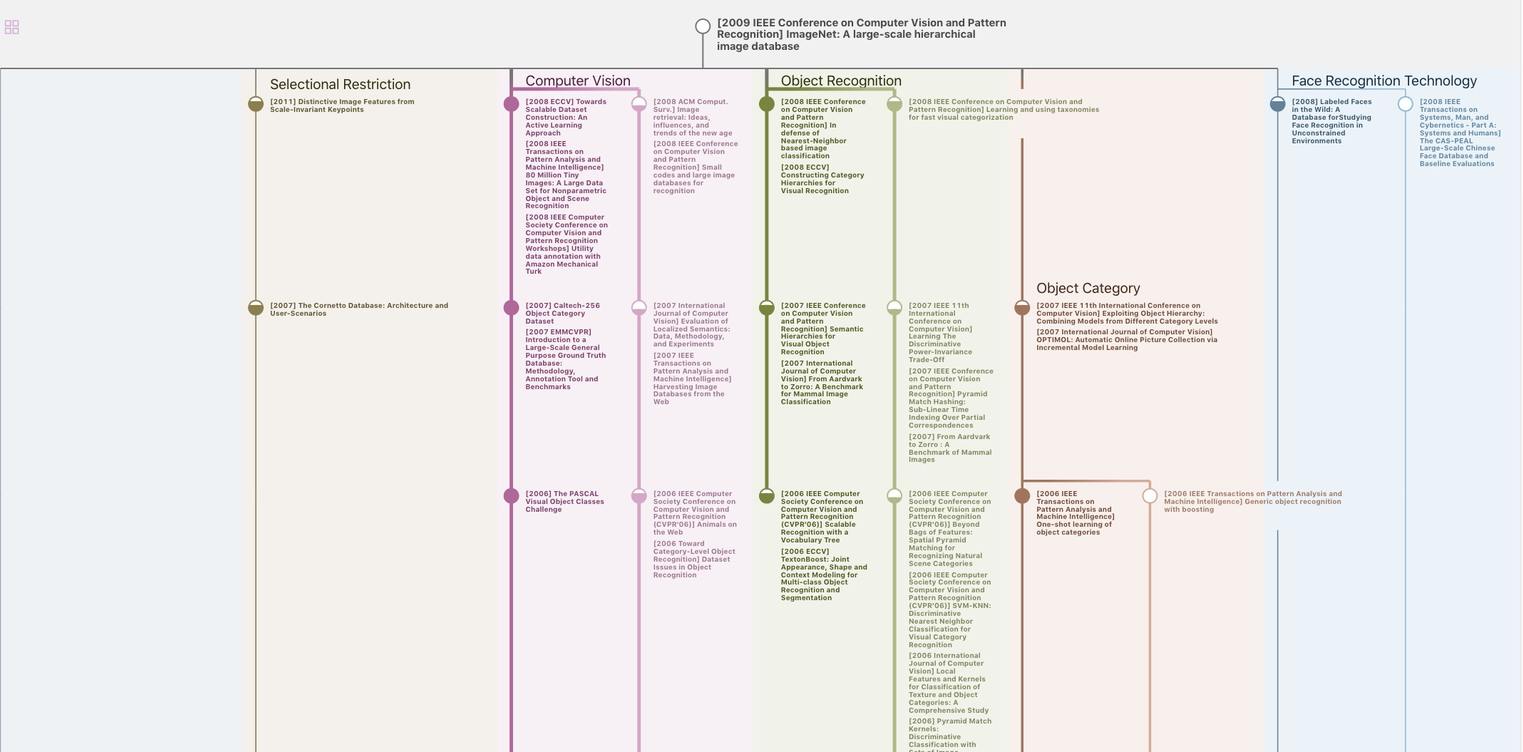
生成溯源树,研究论文发展脉络
Chat Paper
正在生成论文摘要