Unsupervised Deep Embedded Clustering for High-Dimensional Visual Features of Fashion Images
APPLIED SCIENCES-BASEL(2023)
摘要
Fashion image clustering is the key to fashion retrieval, forecasting, and recommendation applications. Manual labeling-based clustering is both time-consuming and less accurate. Currently, popular methods for extracting features from data use deep learning techniques, such as a Convolutional Neural Network (CNN). These methods can generate high-dimensional feature vectors, which are effective for image clustering. However, high dimensions can lead to the curse of dimensionality, which makes subsequent clustering difficult. The fashion images-oriented deep clustering method (FIDC) is proposed in this paper. This method uses CNN to generate a 4096-dimensional feature vector for each fashion image through migration learning, then performs dimensionality reduction through a deep-stacked auto-encoder model, and finally performs clustering on these low-dimensional vectors. High-dimensional vectors can represent images, and dimensionality reduction avoids the curse of dimensionality during clustering tasks. A particular point in the method is the joint learning and optimization of the dimensionality reduction process and the clustering task. The optimization process is performed using two algorithms: back-propagation and stochastic gradient descent. The experimental findings show that the proposed method, called FIDC, has achieved state-of-the-art performance.
更多查看译文
关键词
clustering algorithm,dimensionality reduction,fashion images,deep embedding,stacked autoencoder
AI 理解论文
溯源树
样例
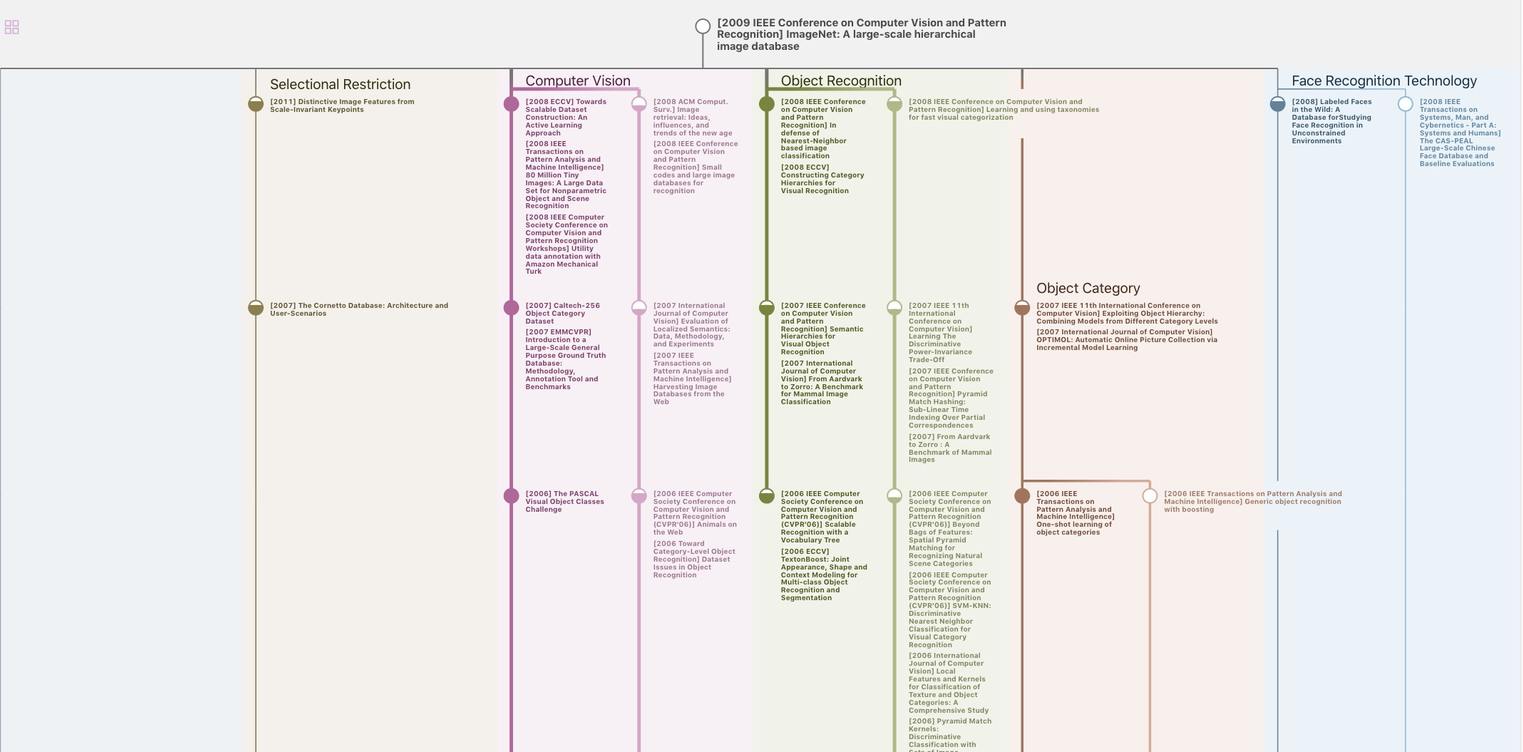
生成溯源树,研究论文发展脉络
Chat Paper
正在生成论文摘要