Handling Missing Values in Surveys With Complex Study Design: A Simulation Study
JOURNAL OF SURVEY STATISTICS AND METHODOLOGY(2024)
摘要
The inverse probability weighting (IPW) method is commonly used to deal with missing-at-random outcome (response) data collected by surveys with complex sampling designs. However, IPW methods generally assume that fully observed predictor variables are available for all sampled units, and it is unclear how to appropriately implement these methods when one or more independent variables are subject to missing values. Multiple imputation (MI) methods are well suited for a variety of missingness patterns but are not as easily adapted to complex sampling designs. In this case study, we consider the National Survey of Morbidity and Risk Factors (EMENO), a multistage probability sample survey. To understand the strengths and limitations of using either missing data treatment method for the EMENO, we present an extensive simulation study modeled on the EMENO health survey, with the target analysis being the estimation of population prevalence of hypertension as well as the association between hypertension and income. Both variables are subject to missingness. We test a variety of IPW and MI methods in simulation and on empirical data from the survey, assessing robustness by varying missingness mechanisms, proportions of missingness, and strengths of fitted response propensity models.
更多查看译文
关键词
Inverse probability weighting,Missing data,Multiple imputation,Survey
AI 理解论文
溯源树
样例
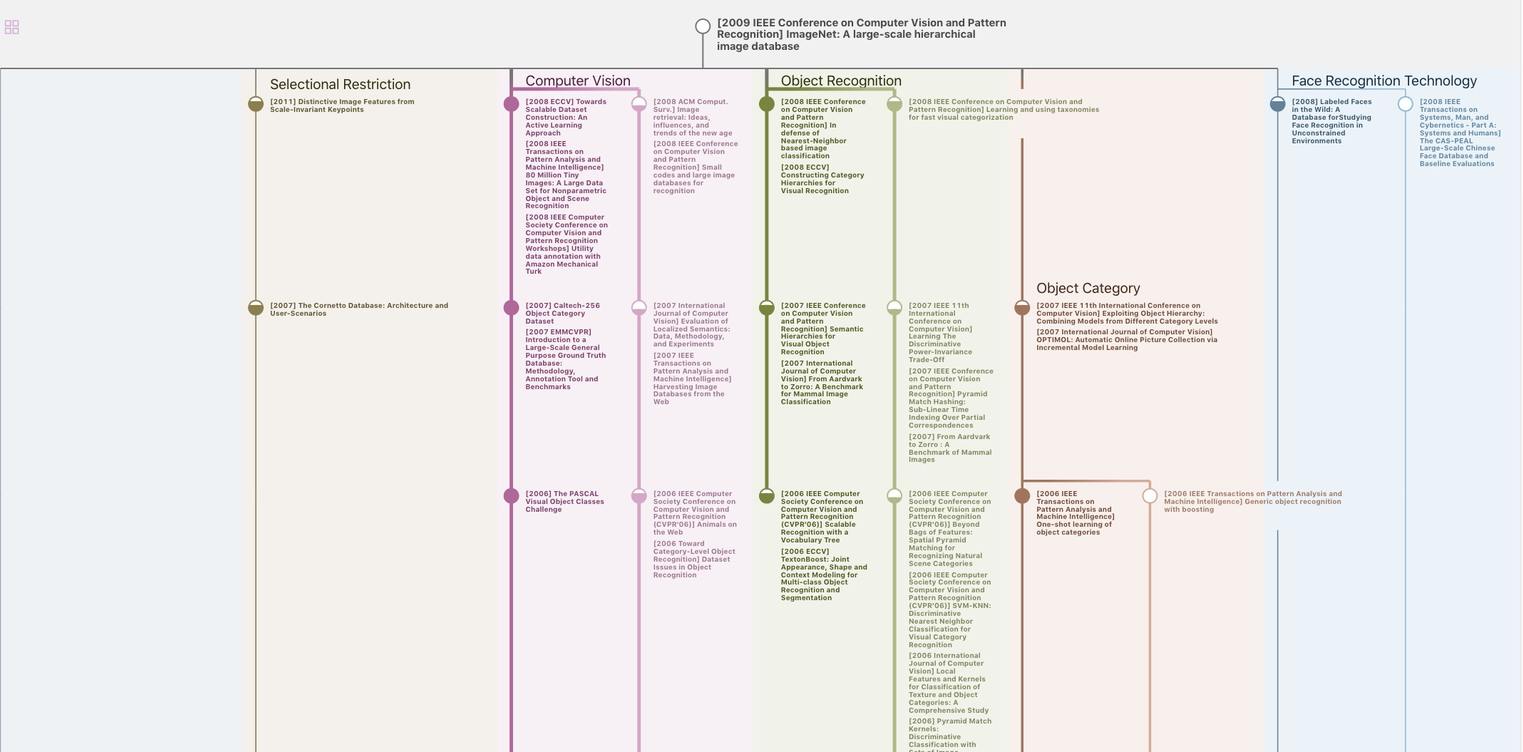
生成溯源树,研究论文发展脉络
Chat Paper
正在生成论文摘要