Global Pose Initialization Based on Gridded Gaussian Distribution With Wasserstein Distance
IEEE TRANSACTIONS ON INTELLIGENT TRANSPORTATION SYSTEMS(2023)
Abstract
Feature descriptors, as abstraction of the critical information in the lidar point clouds, are often used in global pose initialization in large-area to provide a pose reference for intelligent driving system. The current state-of-the-art method Scan Context descriptor is generated based on points' maximum height and, therefore, designed especially for outdoor scenarios without a ceiling. This study proposes a generic descriptor for both outdoor and indoor scenarios based on the point cloud's Gridded Gaussian Distribution (GGD). Wasserstein distance is introduced to this field to evaluate the proposed GGD descriptors' matching performance because it not only has a solid mathematical foundation in comparing two Gaussian distributions but also shows excellent time efficiency via a straightforward analytical solution without enumeration or iteration. We construct a multi-step error function to initialize the vehicle pose using conventional cosine similarity, and Wasserstein distance. Two experiments are designed for this study. The first experiment compares the pose initialization performances under various multi-frame superimposition distance in space to find an efficient GGD descriptor extraction setting. The second experiment verifies that the proposed method achieves a better pose initialization success rate than the mainstream methods.
MoreTranslated text
Key words
Point cloud compression,Gaussian distribution,Task analysis,Location awareness,Pose estimation,Laser radar,Three-dimensional displays,Global pose initialization,point cloud descriptors,gridded Gaussian distribution,Wasserstein distance,indoor scenarios
AI Read Science
Must-Reading Tree
Example
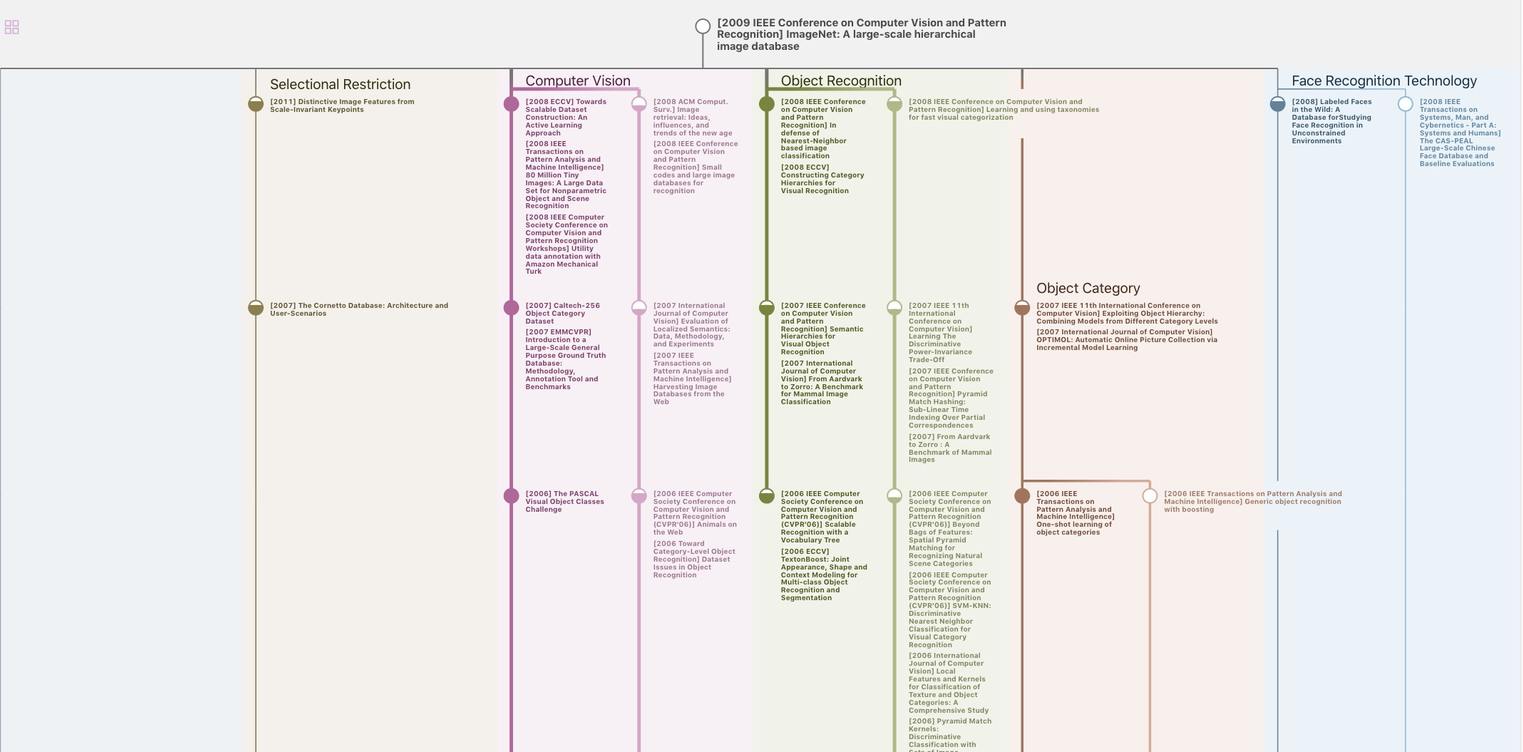
Generate MRT to find the research sequence of this paper
Chat Paper
Summary is being generated by the instructions you defined