Deep learning for exploring ultra-thin ferroelectrics with highly improved sensitivity of piezoresponse force microscopy
NPJ COMPUTATIONAL MATERIALS(2023)
Abstract
Hafnium oxide-based ferroelectrics have been extensively studied because of their existing ferroelectricity, even in ultra-thin film form. However, studying the weak response from ultra-thin film requires improved measurement sensitivity. In general, resonance-enhanced piezoresponse force microscopy (PFM) has been used to characterize ferroelectricity by fitting a simple harmonic oscillation model with the resonance spectrum. However, an iterative approach, such as traditional least squares (LS) fitting, is sensitive to noise and can result in the misunderstanding of weak responses. In this study, we developed the deep neural network (DNN) hybrid with deep denoising autoencoder (DDA) and principal component analysis (PCA) to extract resonance information. The DDA/PCA-DNN improves the PFM sensitivity down to 0.3 pm, allowing measurement of weak piezoresponse with low excitation voltage in 10-nm-thick Hf 0.5 Zr 0.5 O 2 thin films. Our hybrid approaches could provide more chances to explore the low piezoresponse of the ultra-thin ferroelectrics and could be applied to other microscopic techniques.
MoreTranslated text
Key words
piezoresponse force microscopy,deep learning,ultra-thin
AI Read Science
Must-Reading Tree
Example
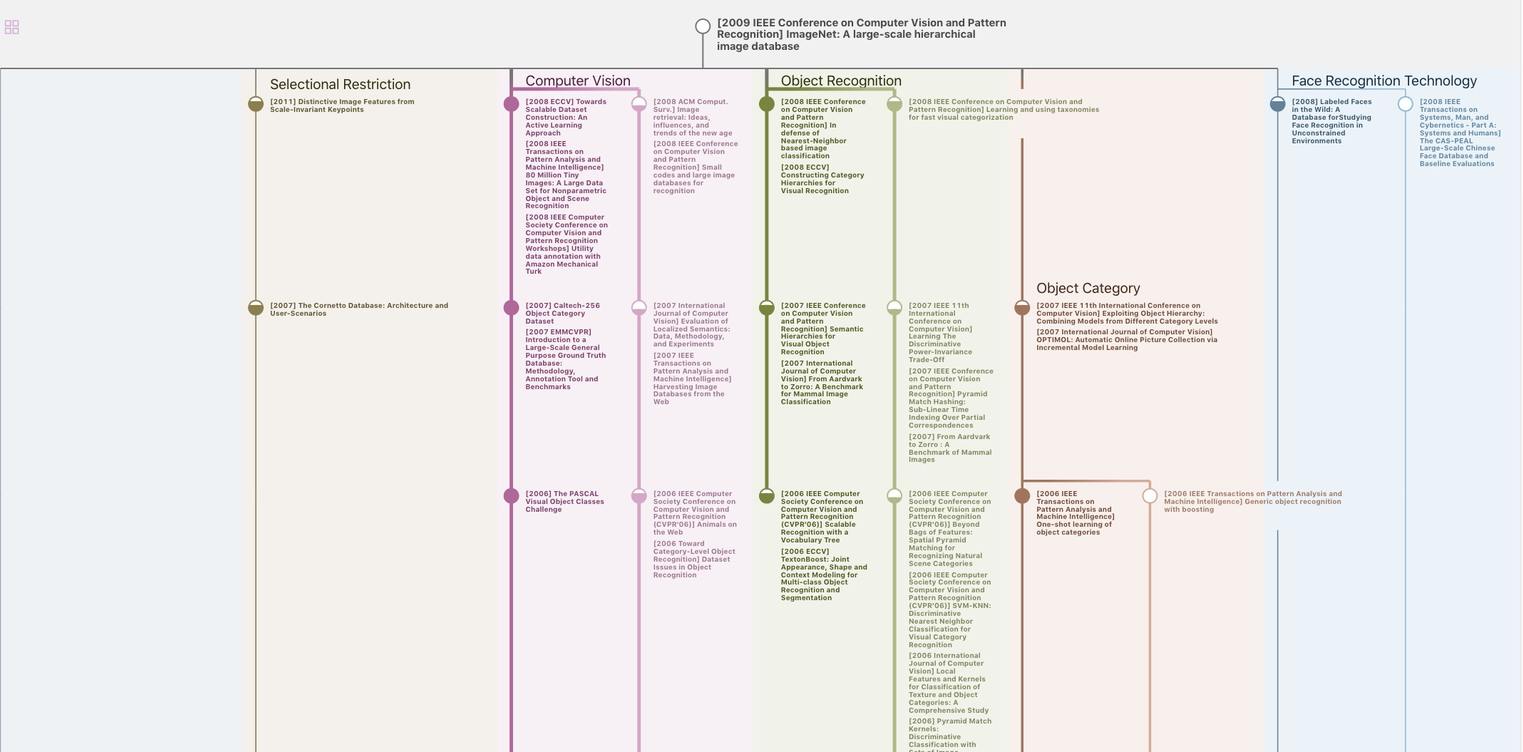
Generate MRT to find the research sequence of this paper
Chat Paper
Summary is being generated by the instructions you defined