Joint Use of Far-Infrared and Mid-Infrared Observation for Sounding Retrievals: Learning From the Past for Upcoming Far-Infrared Missions
EARTH AND SPACE SCIENCE(2023)
摘要
Atmosphere and surface properties are routinely retrieved from satellite measurements and extensively used in weather forecast and climate analysis. Satellite missions dedicated to fill the gap of far-infrared (far-IR) observations are scheduled to be launched this decade. To explore mid-infrared (mid-IR) and far-IR joint retrievals for the future far-IR satellite missions, this study uses an optimal-estimation-based method to retrieve atmospheric specific humidity and temperature profiles, surface skin temperature, and surface spectral emissivity from the Infrared Interferometer Sounder-D (IRIS-D) measurements in 1970, the only existing spaceborne far-IR spectral observations with global coverage. Based on a set of criteria, two cases in the Arctic, which are most likely under clear-sky conditions, are chosen for the retrieval experiments. Information content analysis suggests that the retrieved surface skin temperature and the mid-IR surface spectral emissivity are highly sensitive to the true values while the retrieval estimates of far-IR surface emissivity are subject to the variation of water vapor abundance. Results show that radiances based on the retrieved state variables are more consistent with the IRIS-D observations compared to those based on the reanalysis data. Retrieval estimates of the state variables along with retrieval uncertainties generally fall within reasonable ranges. The relative uncertainties of retrieved state variables decrease compared to the a priori relative uncertainties. In addition, the necessity to retrieve surface emissivity is corroborated by a parallel retrieval experiment assuming a blackbody surface emissivity that has revealed significant distortions of retrieved specific humidity and temperature profiles in the Arctic lower troposphere.
更多查看译文
关键词
atmospheric retrieval,far-infrared,satellite observation
AI 理解论文
溯源树
样例
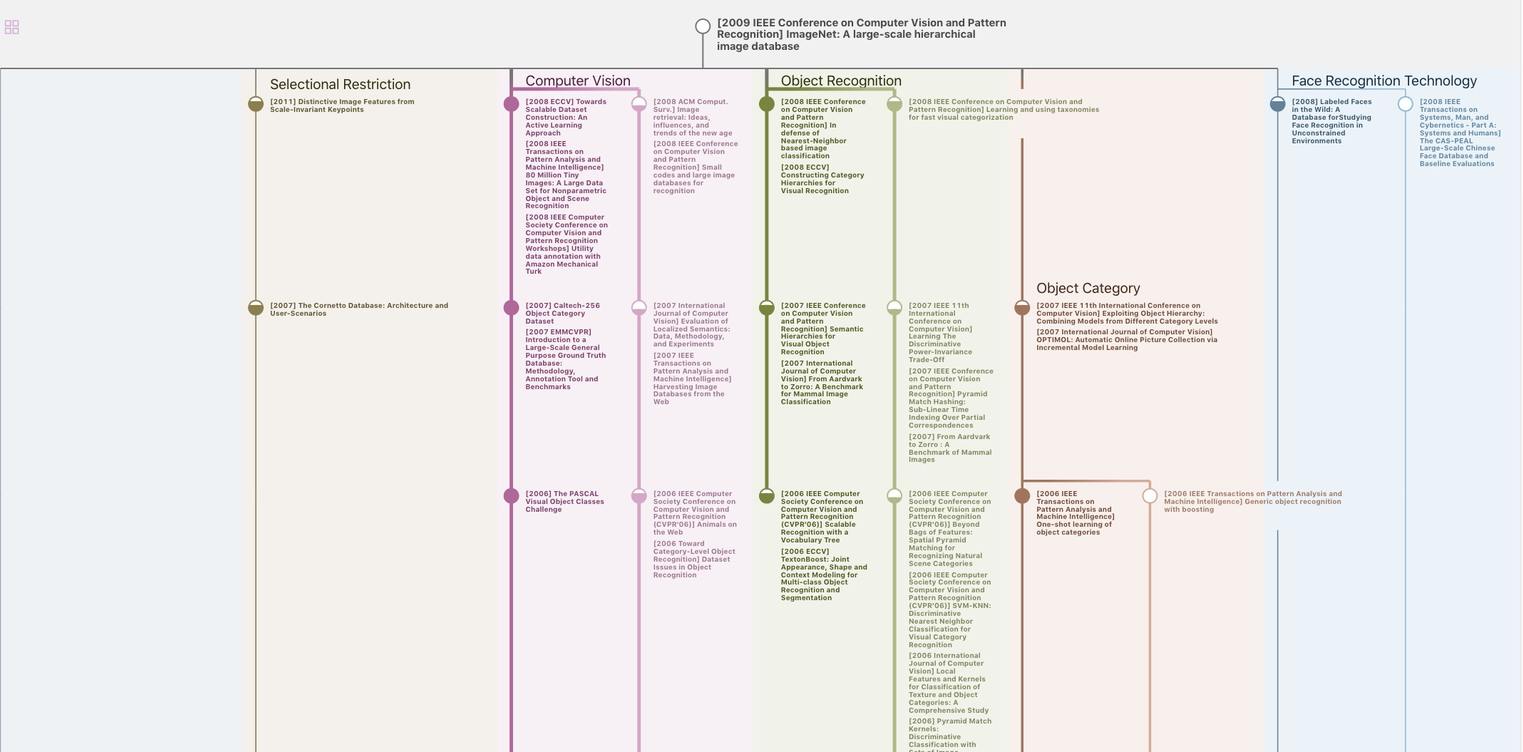
生成溯源树,研究论文发展脉络
Chat Paper
正在生成论文摘要