Identification of potential solid-state Li-ion conductors with semi-supervised learning
ENERGY & ENVIRONMENTAL SCIENCE(2023)
摘要
Despite ongoing efforts to identify high-performance electrolytes for solid-state Li-ion batteries, thousands of prospective Li-containing structures remain unexplored. Here, we employ a semi-supervised learning approach to expedite identification of superionic conductors. We screen 180 unique descriptor representations and use agglomerative clustering to cluster similar to 26 000 Li-containing structures. The clusters are then labeled with experimental ionic conductivity data to assess the fitness of the descriptors. By inspecting clusters containing the highest conductivity labels, we identify 212 promising structures that are further screened using bond valence site energy and nudged elastic band calculations. Li3BS3 is identified as a potential high-conductivity material and selected for experimental characterization. With sufficient defect engineering, we show that Li3BS3 is a superionic conductor with room-temperature ionic conductivity greater than 1 x 10(-3) S cm(-1). While the semi-supervised method shows promise for identification of superionic conductors, the results illustrate a continued need for descriptors that explicitly encode for defects.
更多查看译文
关键词
solid-state solid-state,learning,li-ion,semi-supervised
AI 理解论文
溯源树
样例
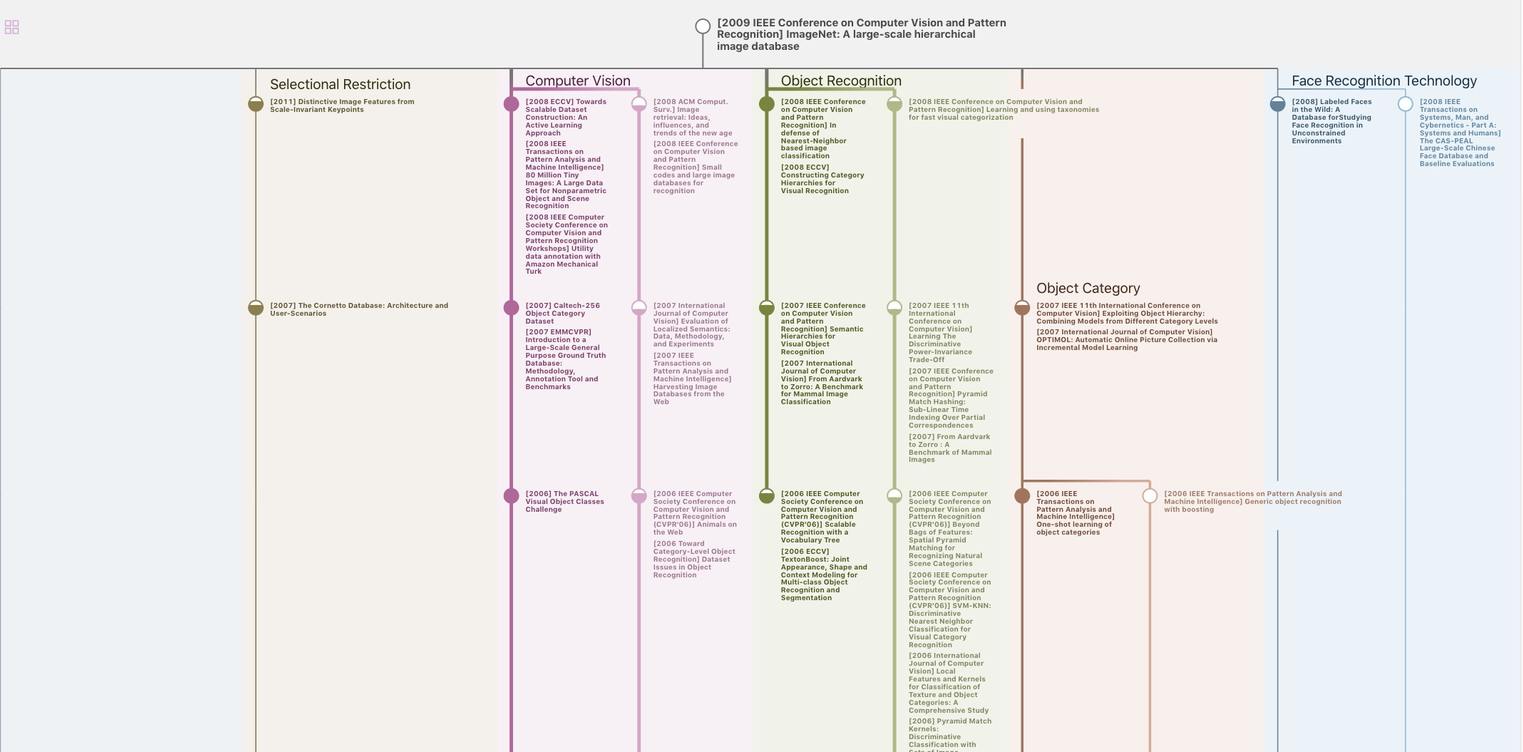
生成溯源树,研究论文发展脉络
Chat Paper
正在生成论文摘要