Adversarial Modeling Regularization for Modeling and Linearization of Power Amplifiers
IEEE TRANSACTIONS ON MICROWAVE THEORY AND TECHNIQUES(2023)
摘要
Overfitting problem is very common in the coefficient identification process of digital predistortion (DPD) technique. Especially in some related DPD topics, the overfitting problem seriously limits the linearization performance. In this article, to improve the robustness of coefficient identification, we present the adversarial modeling regularization (AMR) technique. By regarding the linear-in-coefficient power amplifier (PA) behavioral models as the prior knowledge of the PA itself, the proposed regularization constrains the PA model's coefficients by evaluating the fitting performance of other adversarial models. In addition, we present two complexity reduction strategies for the AMR. The AMR is extended and validated under four related tasks that are prone to overfitting: the few-sample learning, the piecewise DPD technique, the aliasing-sampling technique, and the band-limited technique. The experiment results show that the proposed technique can dramatically improve the forward behavioral modeling and linearization performance compared with the other state-of-the-art algorithms in all tasks.
更多查看译文
关键词
Behavioral sciences,Fitting,Training,Complexity theory,Task analysis,Estimation,Predistortion,Adversarial modeling regularization (AMR),digital predistortion (DPD),linearization,overfitting,power amplifier (PA),robustness
AI 理解论文
溯源树
样例
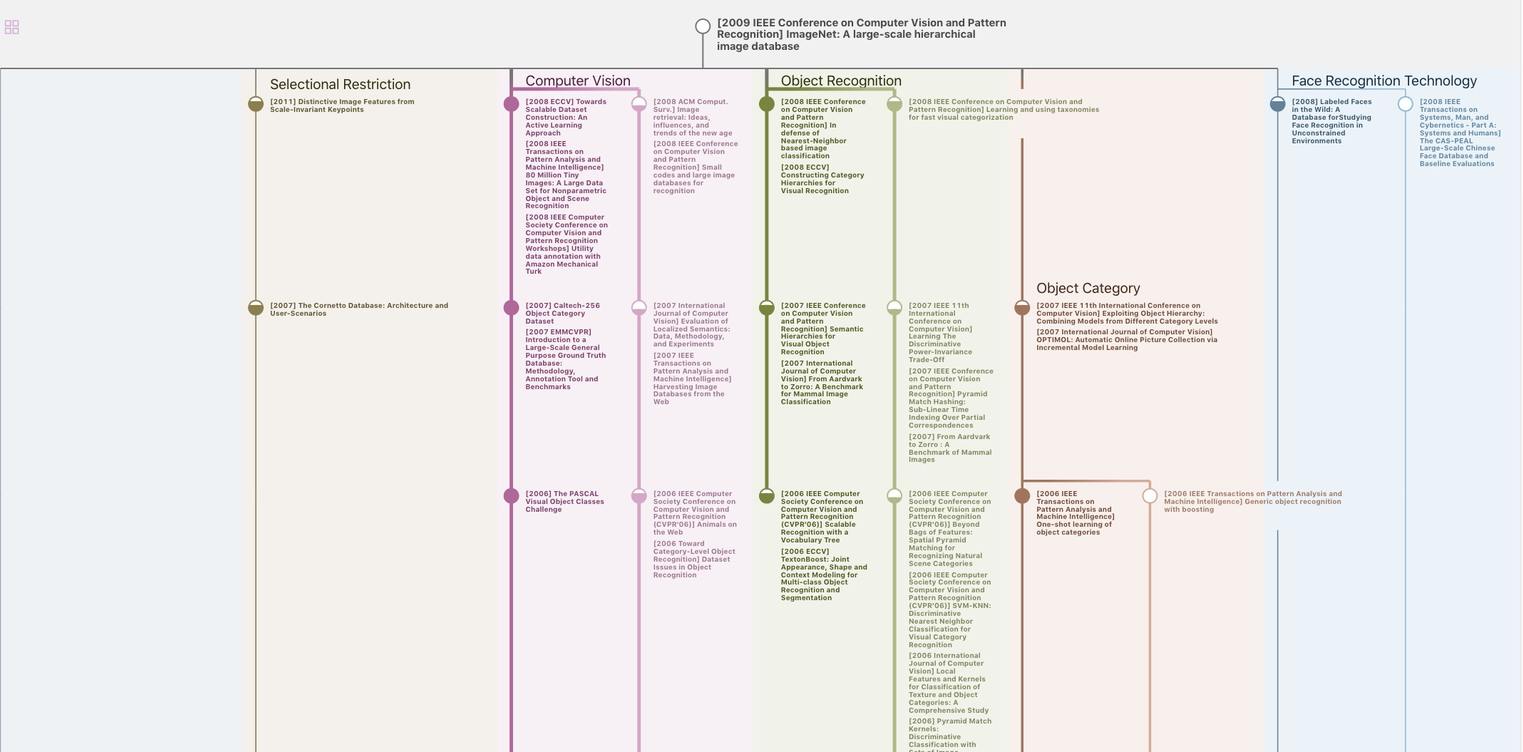
生成溯源树,研究论文发展脉络
Chat Paper
正在生成论文摘要