Millimeter-Wave Image Deblurring via Cycle-Consistent Adversarial Network
ELECTRONICS(2023)
摘要
Millimeter-wave (MMW) imaging has a tangible prospect in concealed weapon detection for security checks. Typically, a one-dimensional (1D) linear antenna array with mechanical scanning along a perpendicular direction is employed for MMW imaging. To achieve high-resolution imaging, the target under test needs to keep steady enough during the mechanical scanning process since slight movement can induce large phase variation for MMW systems, which will result in a blurred image. However, in the scenario of imaging of a human body, sometimes it is difficult to meet this requirement, especially for the elderly. Such blurred MMW images would reduce the detection accuracy of the concealed weapons. In this paper, we propose a deblurring method based on cycle-consistent adversarial network (Cycle GAN). Specifically, the Cycle GAN can learn the mapping between the blurred MMW images and the focused ones. To minimize the effect of the shaking blur, we introduce an identity loss. Moreover, a mean squared error loss (MSE loss) is utilized to stabilize the training, so as to obtain more refined deblurred results. The experimental results demonstrate that the proposed method can efficiently suppress the blurring effect in the MMW image.
更多查看译文
关键词
millimeter-wave imaging,mechanical scanning,image deblurring,Cycle GAN
AI 理解论文
溯源树
样例
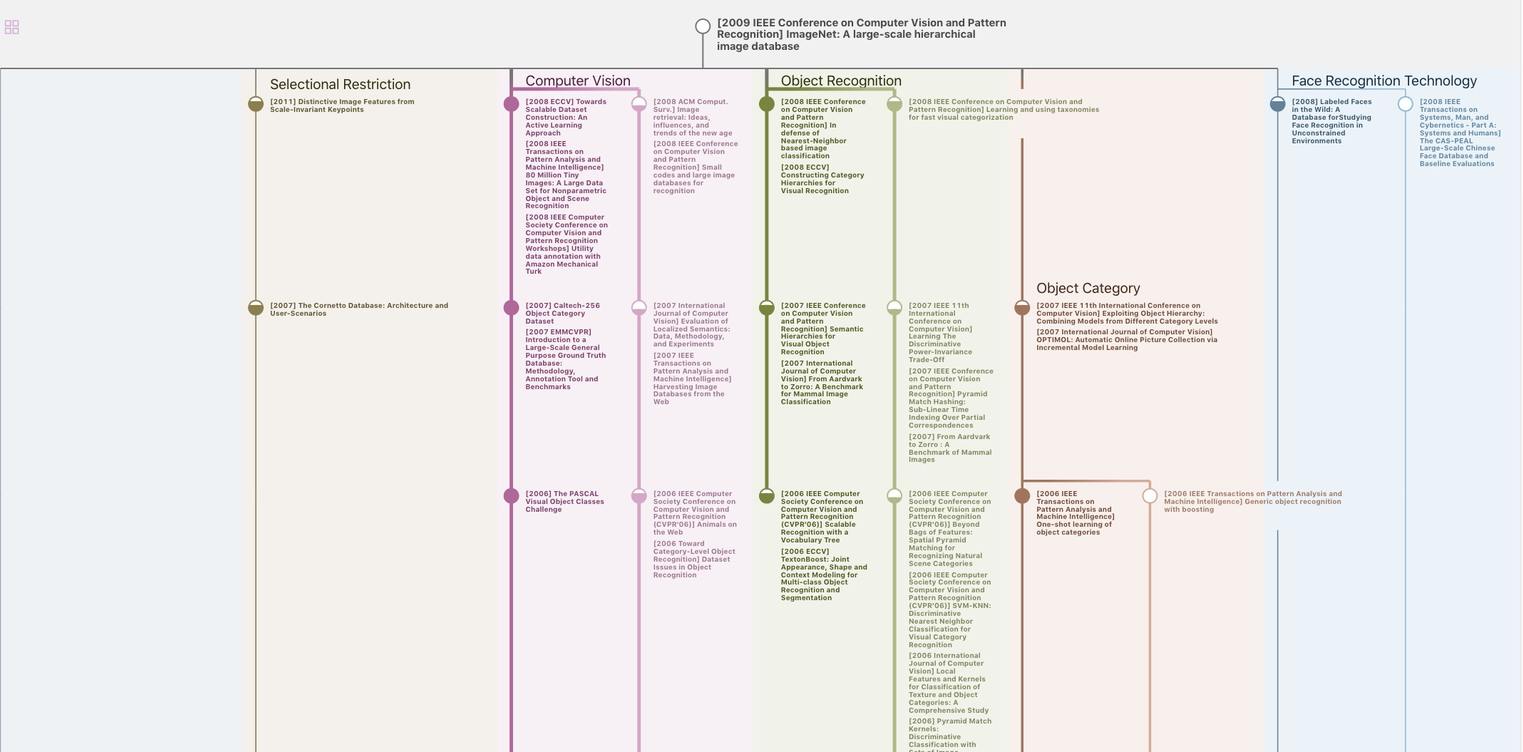
生成溯源树,研究论文发展脉络
Chat Paper
正在生成论文摘要