EDMH: Efficient discrete matrix factorization hashing for multi-modal similarity retrieval
INFORMATION PROCESSING & MANAGEMENT(2023)
Abstract
Hashing has been an emerging topic and has recently attracted widespread attention in multi -modal similarity search applications. However, most existing approaches rely on relaxation schemes to generate binary codes, leading to large quantization errors. In addition, amounts of existing approaches embed labels into the pairwise similarity matrix, leading to expensive time and space costs and losing category information. To address these issues, we propose an Efficient Discrete Matrix factorization Hashing (EDMH). Specifically, EDMH first learns the latent subspaces for individual modality through matrix factorization strategy, which preserves the semantic structure representation information of each modality. In particular, we develop a semantic label offset embedding learning strategy, improving the stability of label embedding regression. Furthermore, we design an efficient discrete optimization scheme to generate compact binary codes discretely. Eventually, we present two efficient learning strategies EDMH-L and EDMH-S to pursue high-quality hash functions. Extensive experiments on various widely-used databases verify that the proposed algorithms produce significant performance and outperform some state-of-the-art approaches, with an average improvement of 2.50% (for Wiki), 2.66% (for MIRFlickr) and 2.25% (for NUS-WIDE) over the best available results, respectively.
MoreTranslated text
Key words
Cross-modal retrieval,Similarity search,Supervised hashing,Discrete optimization,Matrix factorization
AI Read Science
Must-Reading Tree
Example
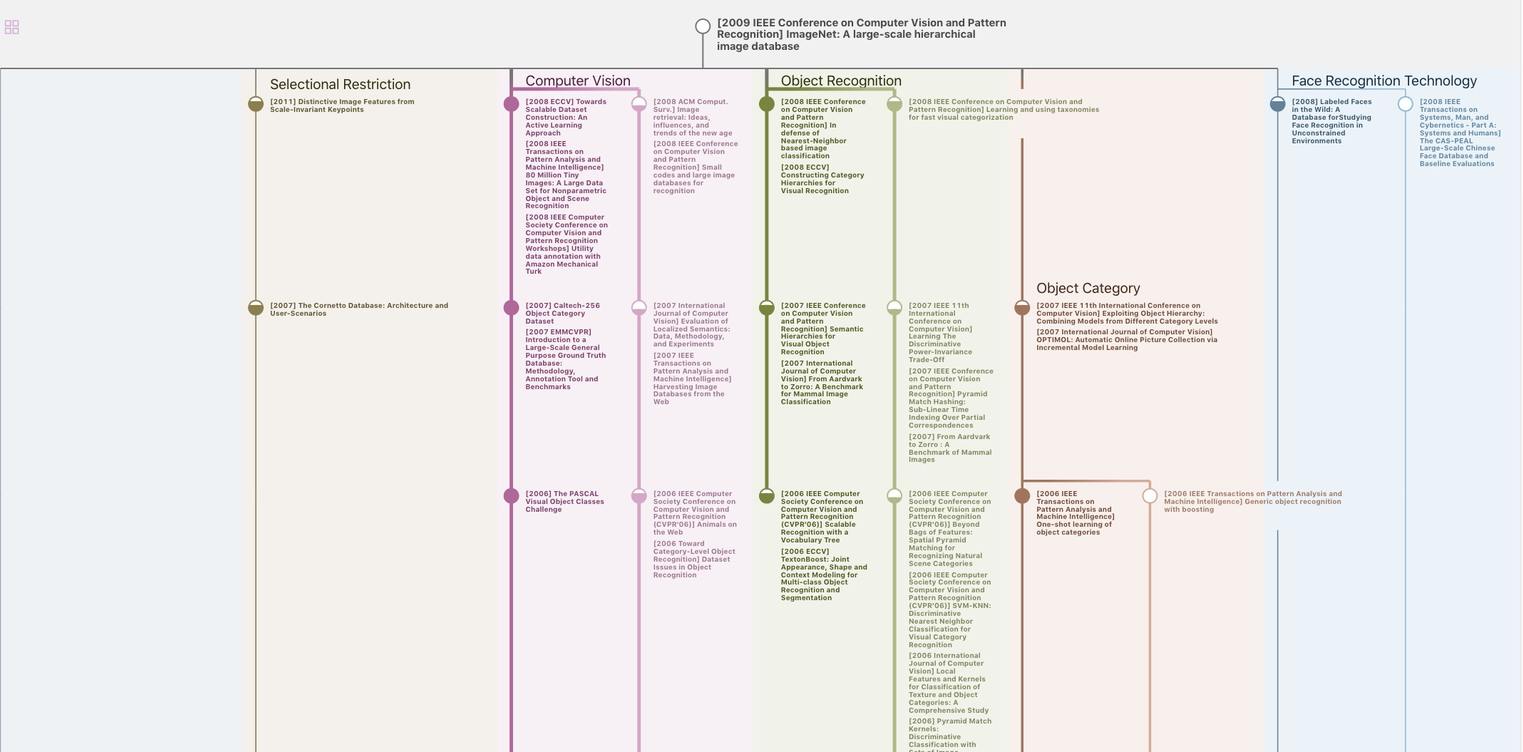
Generate MRT to find the research sequence of this paper
Chat Paper
Summary is being generated by the instructions you defined