An intelligent approach to extract chatter and metal removal rate features impromptu from milling sound signal
PROCEEDINGS OF THE INSTITUTION OF MECHANICAL ENGINEERS PART E-JOURNAL OF PROCESS MECHANICAL ENGINEERING(2023)
摘要
Vibration monitoring and control have been the main concern of machining industries. Traditional monitoring processes have several drawbacks such as; higher computational time, manual feature detection and requirement of supervision. To overcome these difficulties, this study proposes a hybrid approach based on Self-Organizing Maps (SOM) and multi-layer perceptron - back propagation neural network (MLP-BPNN) using sound signals for monitoring self-induced tool vibration (chatter) and metal removal rate (MRR) in milling operation. Initially, acquired vibration signals are decomposed for extracting desired machining data. Further, SOM has been applied on the reconstructed signals for data mapping and automatic feature selection. Selected features have been used as an input in MLP-BPNN training for the development of prediction models of machining quality and MRR. Finally, it has been observed that the proposed data-driven methodology can be well adapted for the automatic feature selection and can predict machining quality and MRR with nearly 98% accuracy.
更多查看译文
关键词
SOM,LM-BPNN,condition monitoring,milling chatter,SBLMD
AI 理解论文
溯源树
样例
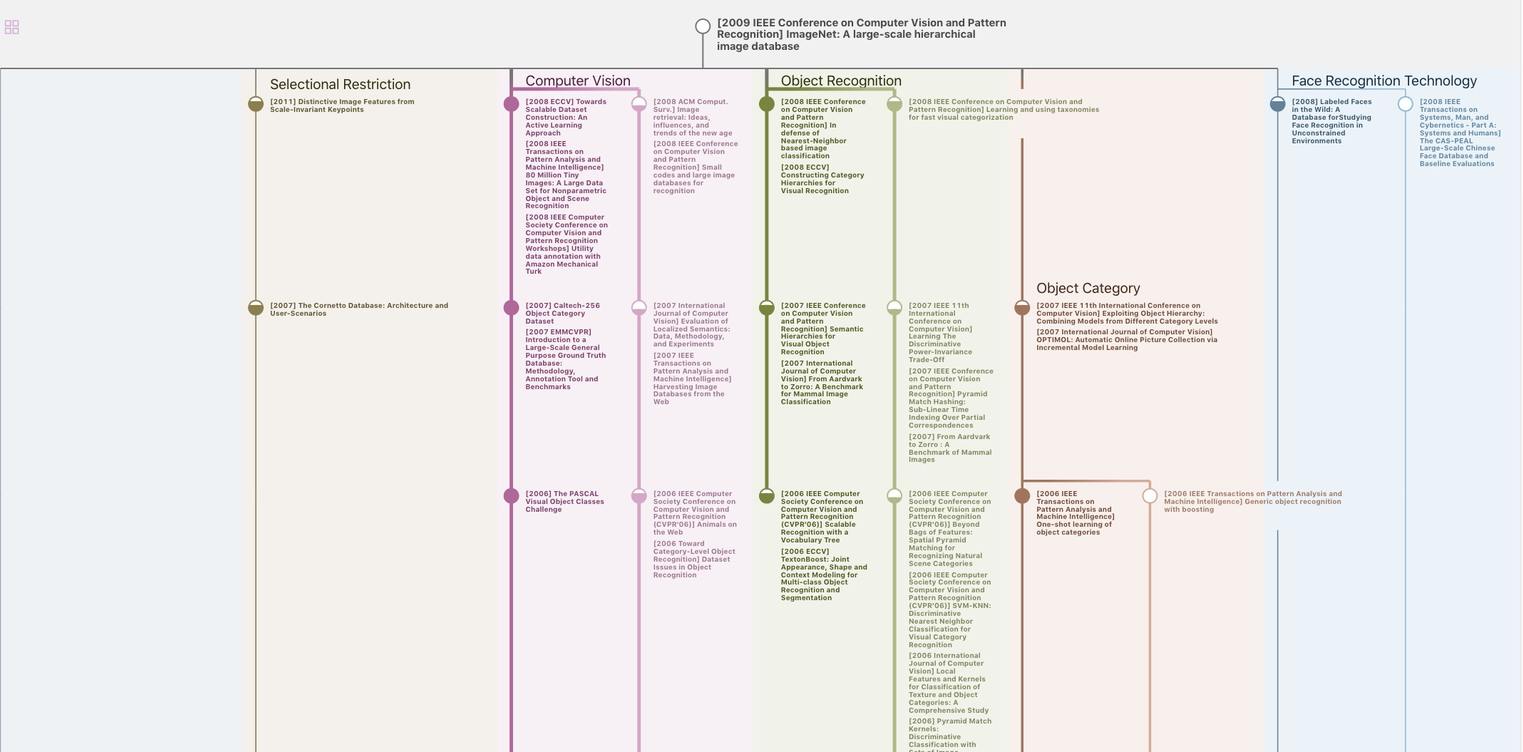
生成溯源树,研究论文发展脉络
Chat Paper
正在生成论文摘要