Explainable human activity recognition based on probabilistic spatial partitions for symbiotic workplaces
INTERNATIONAL JOURNAL OF COMPUTER INTEGRATED MANUFACTURING(2023)
摘要
In recent years, smart workplaces that adapt to human activity and motion behavior have been proposed for cognitive production systems. In this respect, methods for identifying the feelings and activities of human workers are being investigated to improve the cognitive capability of smart machines such as robots in shared working spaces. Recognizing human activities and predicting the possible next sequence of operations may simplify robot programming and improve collaboration efficiency. However, human activity recognition still requires explainable models that are versatile, robust, and interpretative. Therefore, recognizing and analyzing human action details using continuous probability density estimates in different workplace layouts is essential. Three scenarios are considered: a standalone, a one-piece flow U-form, and a human-robot hybrid workplace. This work presents a novel approach to human activity recognition based on a probabilistic spatial partition (HAROPP). Its performance is compared to the geometric-bounded activity recognition method. Results show that spatial partitions based on probabilistic density contain 20% fewer data frames and 10% more spatial areas than the geometric bounding box. The approach, on average, detects human activities correctly for 81% of the cases for a pre-known workplace layout. HAROPP has scalability and applicability potential for cognitive workplaces with a digital twin in the loop for pushing the cognitive capabilities of machine systems and realizing human-centered environments.
更多查看译文
关键词
Human activity recognition,continuous probabilistic model,workplace layout,human motion capture,human-robot collaboration
AI 理解论文
溯源树
样例
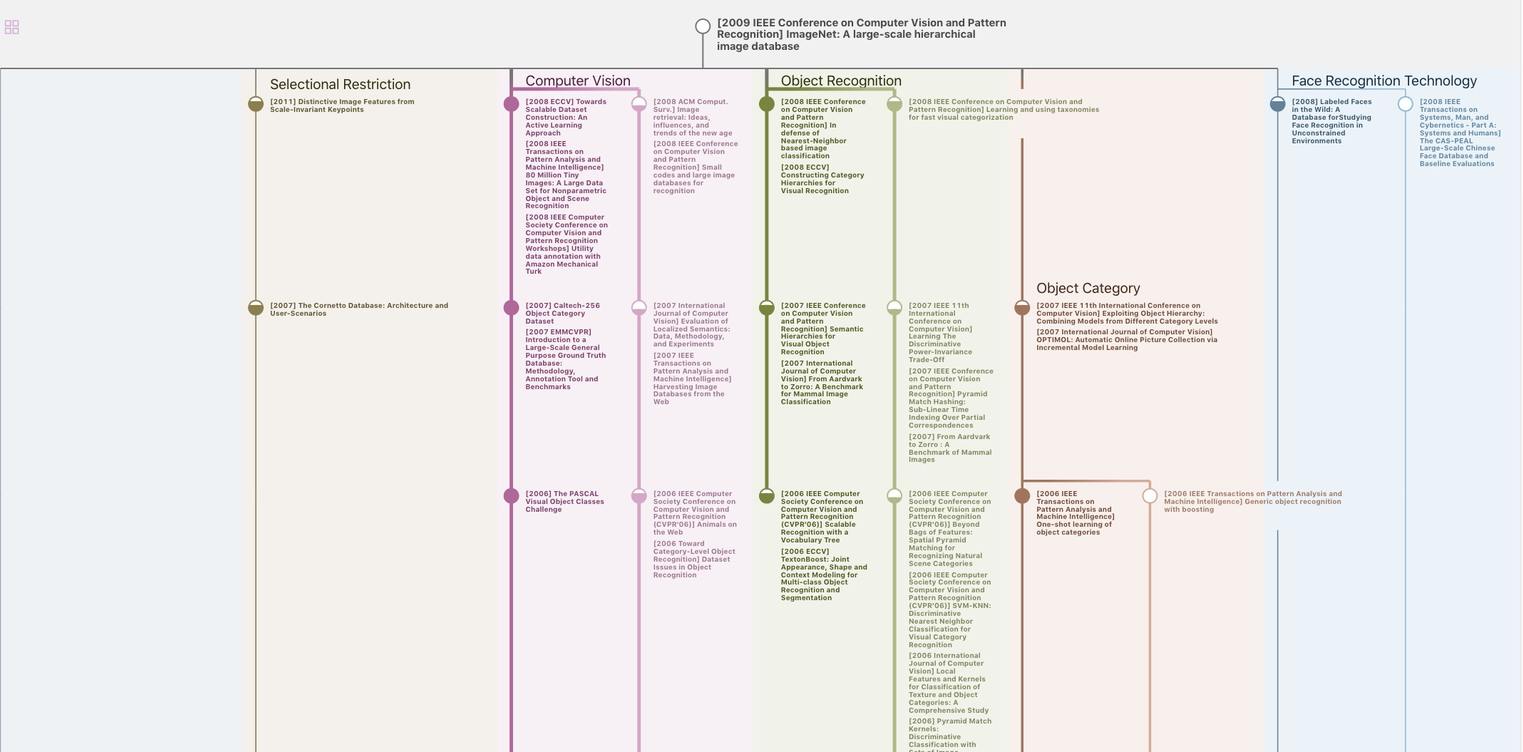
生成溯源树,研究论文发展脉络
Chat Paper
正在生成论文摘要