Attentional Extractive Summarization
APPLIED SCIENCES-BASEL(2023)
Abstract
In this work, a general theoretical framework for extractive summarization is proposed-the Attentional Extractive Summarization framework. Although abstractive approaches are generally used in text summarization today, extractive methods can be especially suitable for some applications, and they can help with other tasks such as Text Classification, Question Answering, and Information Extraction. The proposed approach is based on the interpretation of the attention mechanisms of hierarchical neural networks, which compute document-level representations of documents and summaries from sentence-level representations, which, in turn, are computed from word-level representations. The models proposed under this framework are able to automatically learn relationships among document and summary sentences, without requiring Oracle systems to compute the reference labels for each sentence before the training phase. These relationships are obtained as a result of a binary classification process, the goal of which is to distinguish correct summaries for documents. Two different systems, formalized under the proposed framework, were evaluated on the CNN/DailyMail and the NewsRoom corpora, which are some of the reference corpora in the most relevant works on text summarization. The results obtained during the evaluation support the adequacy of our proposal and suggest that there is still room for the improvement of our attentional framework.
MoreTranslated text
Key words
siamese neural networks,hierarchical neural networks,attention mechanisms,extractive summarization
AI Read Science
Must-Reading Tree
Example
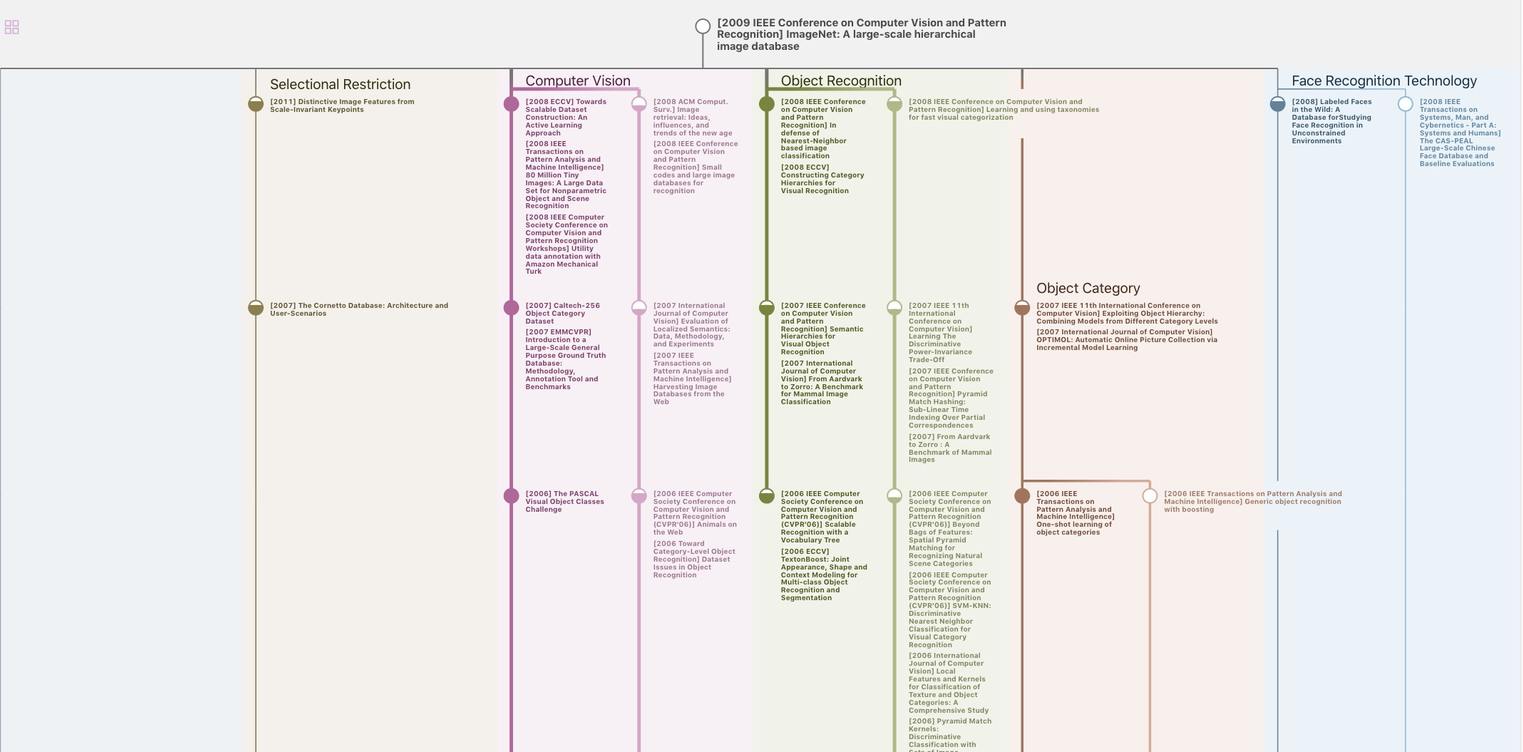
Generate MRT to find the research sequence of this paper
Chat Paper
Summary is being generated by the instructions you defined