Crack Severity Classification from Timber Cross-Sectional Images Using Convolutional Neural Network
APPLIED SCIENCES-BASEL(2023)
摘要
Featured Application Application of convolutional neural network (CNN) for automatic evaluation of internal crack severity in building square timbers. In our previous article, we constructed a simple CNN and employed cross-sectional pictures covered with silver paint to make the cracks easier to recognize. However, in this article, we confirmed that the severity of the internal cracks could be evaluated with high accuracy by a ResNet50-CNN (Residual Neural Network 50 CNN), even in the case of unpainted images. Given that quality managers must evaluate numerous timbers, this automation process reduces the workload. Cedar and cypress used for wooden construction have high moisture content after harvesting. To be used as building materials, they must undergo high-temperature drying. However, this process causes internal cracks that are invisible on the outer surface. These defects are serious because they reduce the strength of the timber, i.e., the buckling strength and joint durability. Therefore, the severity of internal cracks should be evaluated. A square timber was cut at an arbitrary position and assessed based on the length, thickness, and shape of the cracks in the cross-section; however, this process is time-consuming and labor-intensive. Therefore, we used a convolutional neural network (CNN) to automatically evaluate the severity of cracks from cross-sectional timber images. Previously, we used silver-painted images of cross-sections so that the cracks are easier to observe; however, this task was burdensome. Hence, in this study, we attempted to classify crack severity using ResNet (Residual Neural Network) from unpainted images. First, ResNet50 was employed and trained with supervised data to classify the crack severity level. The classification accuracy was then evaluated using test images (not used for training) and reached 86.67%. In conclusion, we confirmed that the proposed CNN could evaluate cross-sectional cracks on behalf of humans.
更多查看译文
关键词
timber,crack,convolutional neural network,ResNet,human fuzziness
AI 理解论文
溯源树
样例
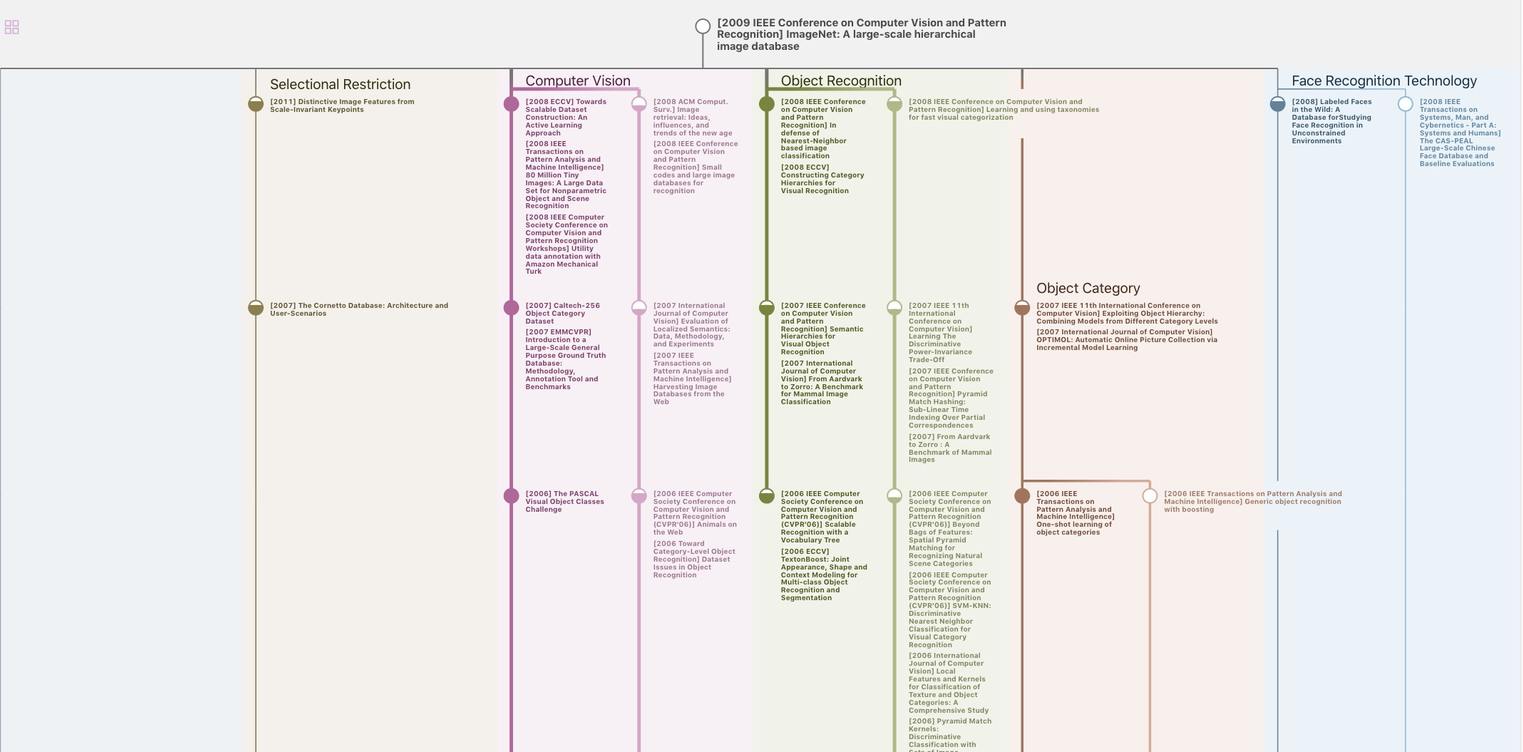
生成溯源树,研究论文发展脉络
Chat Paper
正在生成论文摘要